Sequential Stratified Splitting For Efficient Monte Carlo Integration
SEQUENTIAL ANALYSIS-DESIGN METHODS AND APPLICATIONS(2021)
摘要
The efficient evaluation of high-dimensional integrals is important from both theoretical and practical points of view. In particular, multidimensional integration plays a central role in Bayesian inference, statistical physics, data science, and machine learning. However, due to the curse of dimensionality, deterministic numerical methods are inefficient in the high-dimensional setting. Consequentially, for many practical problems one must resort to approximate estimation techniques such as Monte Carlo methods. In this article, we introduce a novel sequential Monte Carlo algorithm called stratified splitting. The method provides unbiased estimates and can handle various integrand types including indicator functions, which are important for rare-event probability estimation problems. We provide rigorous analysis of the efficiency of the proposed method and present a numerical demonstration of the algorithmic performance when applied to practical application domains. Our numerical experiments suggest that the stratified splitting method is capable of delivering accurate results for a variety of integration problems while requiring reasonable computational effort.
更多查看译文
关键词
Algorithmic efficiency, Markov chain Monte Carlo, multidimensional integration, multilevel splitting, sequential Monte Carlo
AI 理解论文
溯源树
样例
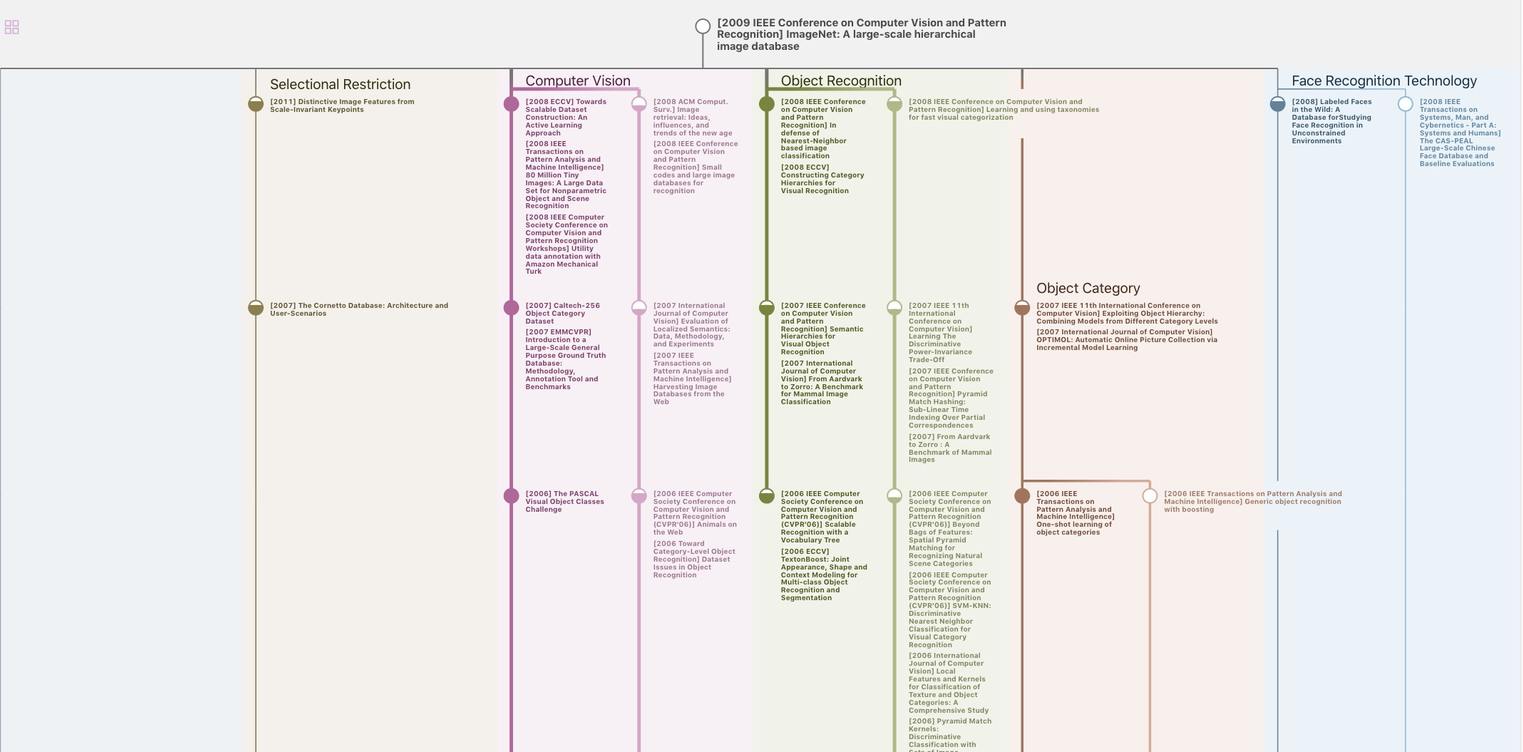
生成溯源树,研究论文发展脉络
Chat Paper
正在生成论文摘要