Machine Learning Methods For Probabilistic Locked-Mode Predictors In Tokamak Plasmas
PHYSICS OF PLASMAS(2021)
摘要
A rotating tokamak plasma can interact resonantly with the external helical magnetic perturbations, also known as error fields. This can lead to locking and then to disruptions. We leverage machine learning (ML) methods to predict the locking events. We use a coupled third-order nonlinear ordinary differential equation model to represent the interaction of the magnetic perturbation and the plasma rotation with the error field. This model is sufficient to describe qualitatively the locking and unlocking bifurcations. We explore using ML algorithms with the simulation data and experimental data, focusing on the methods that can be used with sparse datasets. These methods lead to the possibility of the avoidance of locking in real-time operations. We describe the operational space in terms of two control parameters: the magnitude of the error field and the rotation frequency associated with the momentum source that maintains the plasma rotation. The outcomes are quantified by order parameters that completely characterize the state, whether locked or unlocked. We use unsupervised ML methods to classify locked/unlocked states and note the usefulness of a certain normalization of the order parameters. Three supervised ML classifiers are used in suite to estimate the probability of locking in the region of control parameter space with hysteresis, i.e., the set of control parameters for which both locked and unlocked states can exist. The results show that a neural network gives the best estimate of the locking probability. An analogy of the present locking model with the van der Waals equation of state is also provided. Published under an exclusive license by AIP Publishing.
更多查看译文
AI 理解论文
溯源树
样例
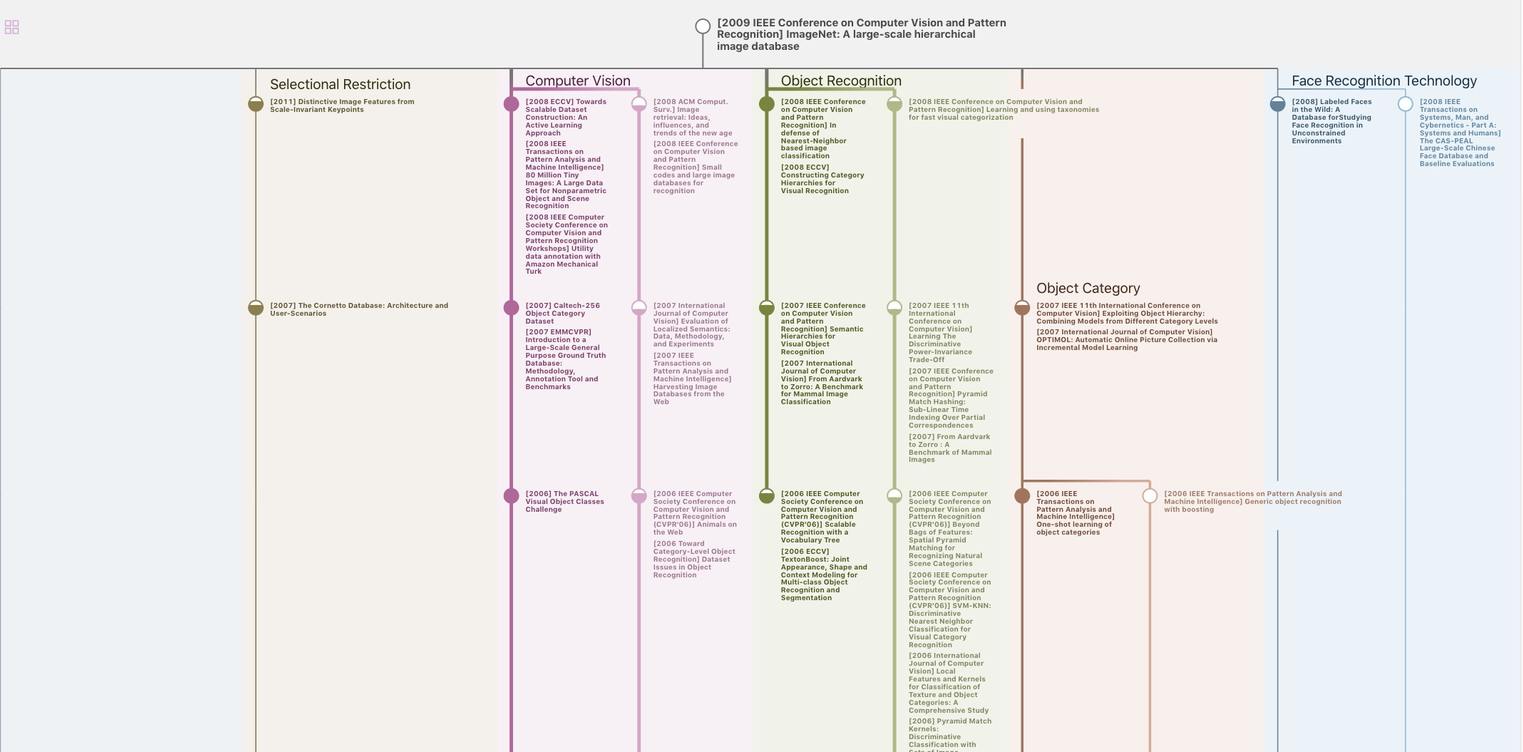
生成溯源树,研究论文发展脉络
Chat Paper
正在生成论文摘要