Imputation For Repeated Bounded Outcome Data: Statistical And Machine-Learning Approaches
MATHEMATICS(2021)
摘要
Real-life data are bounded and heavy-tailed variables. Zero-one-inflated beta (ZOIB) regression is used for modelling them. There are no appropriate methods to address the problem of missing data in repeated bounded outcomes. We developed an imputation method using ZOIB (i-ZOIB) and compared its performance with those of the naive and machine-learning methods, using different distribution shapes and settings designed in the simulation study. The performance was measured employing the absolute error (MAE), root-mean-square-error (RMSE) and the unscaled mean bounded relative absolute error (UMBRAE) methods. The results varied depending on the missingness rate and mechanism. The i-ZOIB and the machine-learning ANN, SVR and RF methods showed the best performance.
更多查看译文
关键词
imputation, bounded outcomes, repeated measures, zero-one-inflated beta distribution, machine learning
AI 理解论文
溯源树
样例
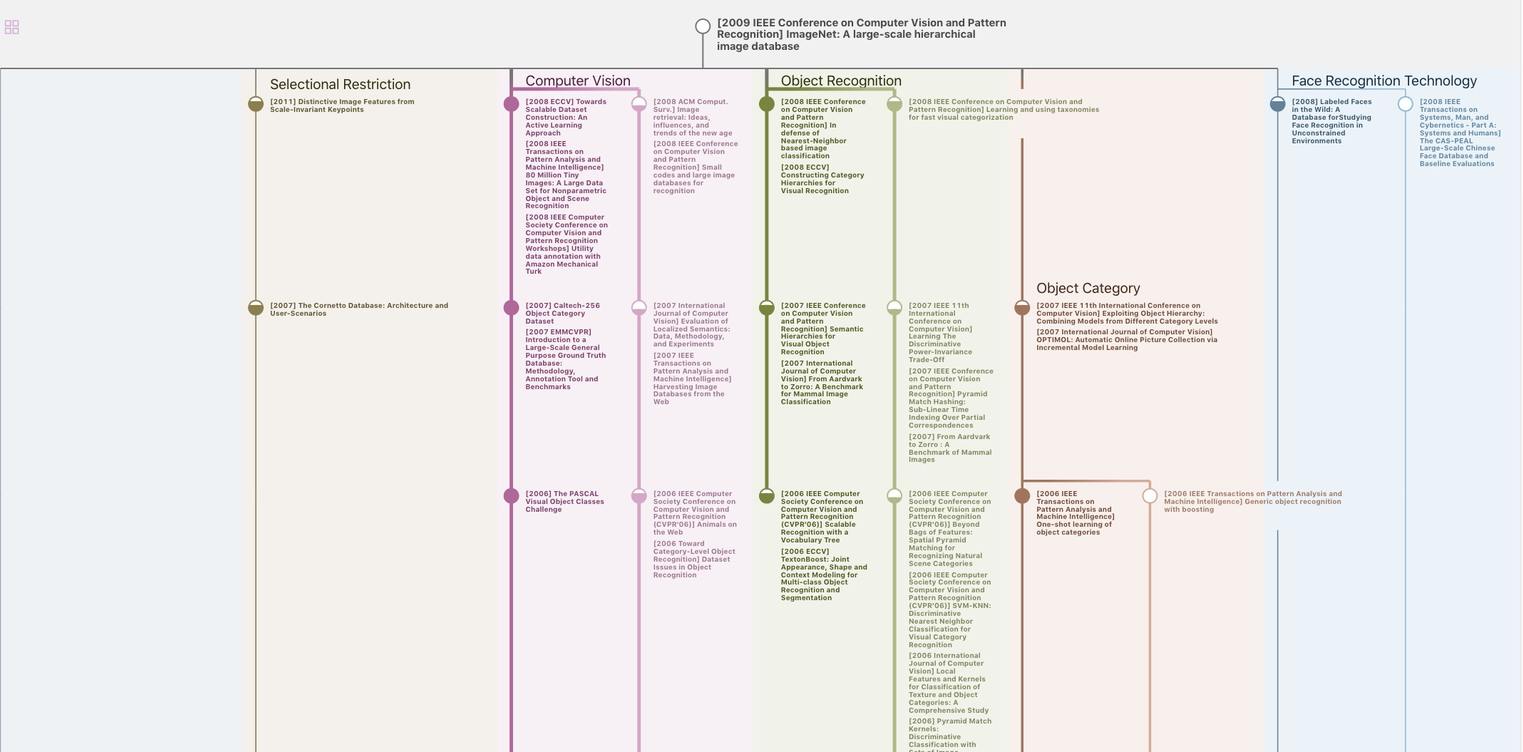
生成溯源树,研究论文发展脉络
Chat Paper
正在生成论文摘要