Cross-scale feature fusion connection for a YOLO detector
IET COMPUTER VISION(2022)
摘要
Multi-scale feature fusion is often used to address the issue of scale variations in object detection. However, most of the proposed network architectures only combine the features of two adjacent levels sequentially, so the first fusion nodes in both top-down and bottom-up pathways must be blank nodes that only have one input with no feature fusion. In this work, cross-scale feature fusion connection (CFFC) is proposed which aims to enhance the entire feature hierarchy by propagating the features of each level more efficiently. The proposed method reuses and aggregates all the features of other scales to the blank nodes in both top-down and bottom-up pathways. Furthermore, the authors remove the 1 x 1 convolutional layer and replace the shortcut with concatenation before fusing multiple features. These concatenated feature maps are then supervised by the channel attention block at the fusion nodes. This modification allows the network to learn the important degree of each level in concatenated feature maps along the channel dimension. It is also observed that the proposed method alleviates the inconsistency in feature pyramids with fewer parameters. The performance of a YOLO object detector equipped with the proposed method on the COCO test-dev 2017 is evaluated. The results show that the proposed method outperforms other architectures presented in the literature.
更多查看译文
关键词
computer vision, image processing, object detection
AI 理解论文
溯源树
样例
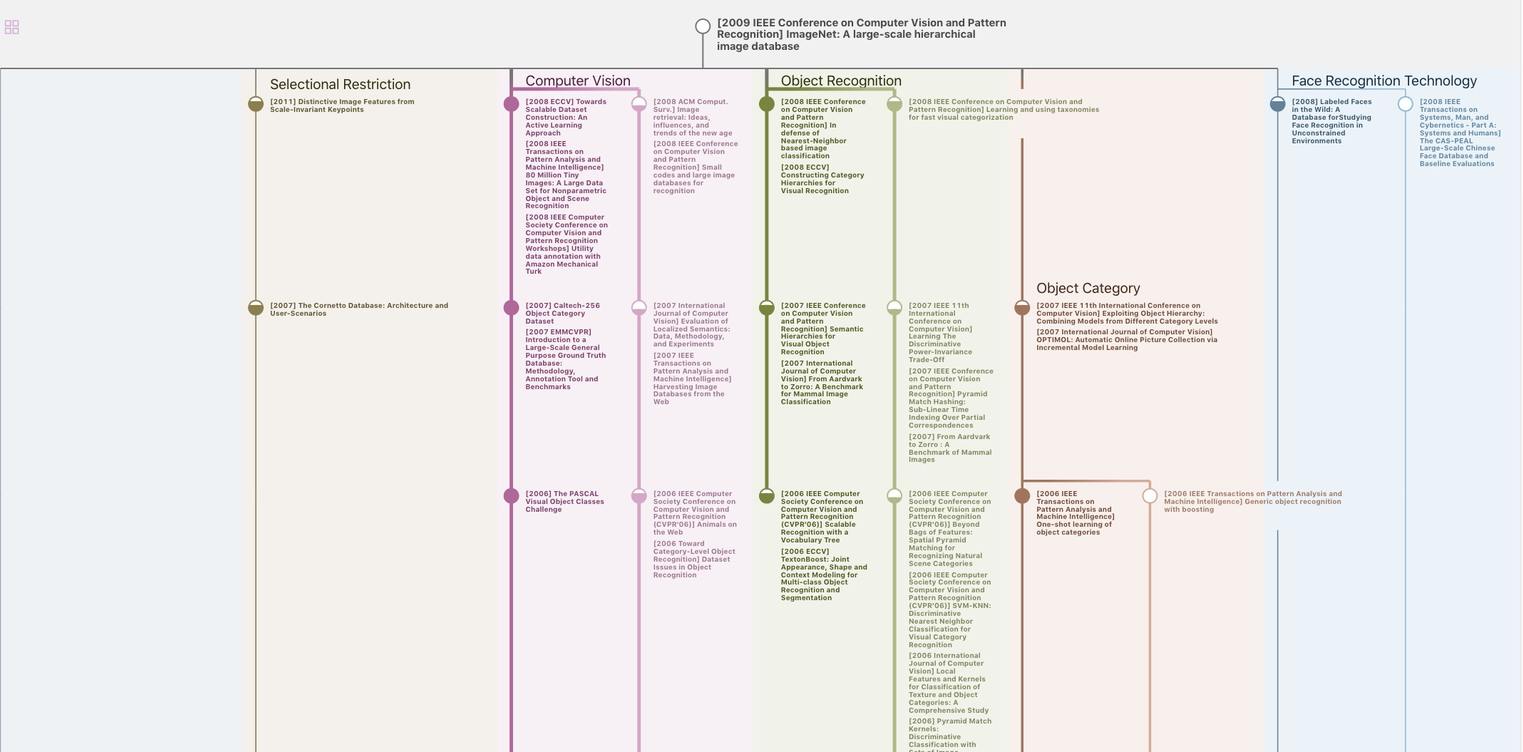
生成溯源树,研究论文发展脉络
Chat Paper
正在生成论文摘要