Low-rank, Orthogonally Decomposable Tensor Regression With Application to Visual Stimulus Decoding of fMRI Data
JOURNAL OF COMPUTATIONAL AND GRAPHICAL STATISTICS(2022)
摘要
We consider the problem of fitting a generalized linear model with a three-dimensional image covariate, such as one obtained by functional magnetic resonance imaging (fMRI). A major challenge for fitting such a model is that the image is a multidimensional array, called a tensor, containing tens of thousands of elements, called voxels. Because there is a parameter associated with each voxel, fitting the model entails estimating tens of thousands of parameters with a typical sample size on the order of hundreds of subjects. We propose to reduce the dimensionality of the problem by imposing a low-rank assumption on the parameter tensor, which not only reduces the number of parameters to estimate, but also exploits the implicit spatial information in fMRI data. In addition, we assume the parameter tensor is orthogonally decomposable, enabling us to penalize the so-called tensor "singular values" to obtain a low-rank estimate. We develop algorithms based on projected gradient descent and the proximal gradient method to estimate the model. In simulation, the proposed methods yielded estimates with smaller squared errors than an existing method based on alternating minimization. For visual stimulus decoding of a real fMRI dataset, the proposed methods resulted in better classification accuracies than the existing method. Visualization of the estimates revealed compact clusters of voxels with relatively large values, potentially representing brain regions relevant to the task. Supplementary files for this article are available online.
更多查看译文
关键词
High-dimensional low sample size, Imaging data, Low-rank approximation, Nuclear norm, Tensor decomposition
AI 理解论文
溯源树
样例
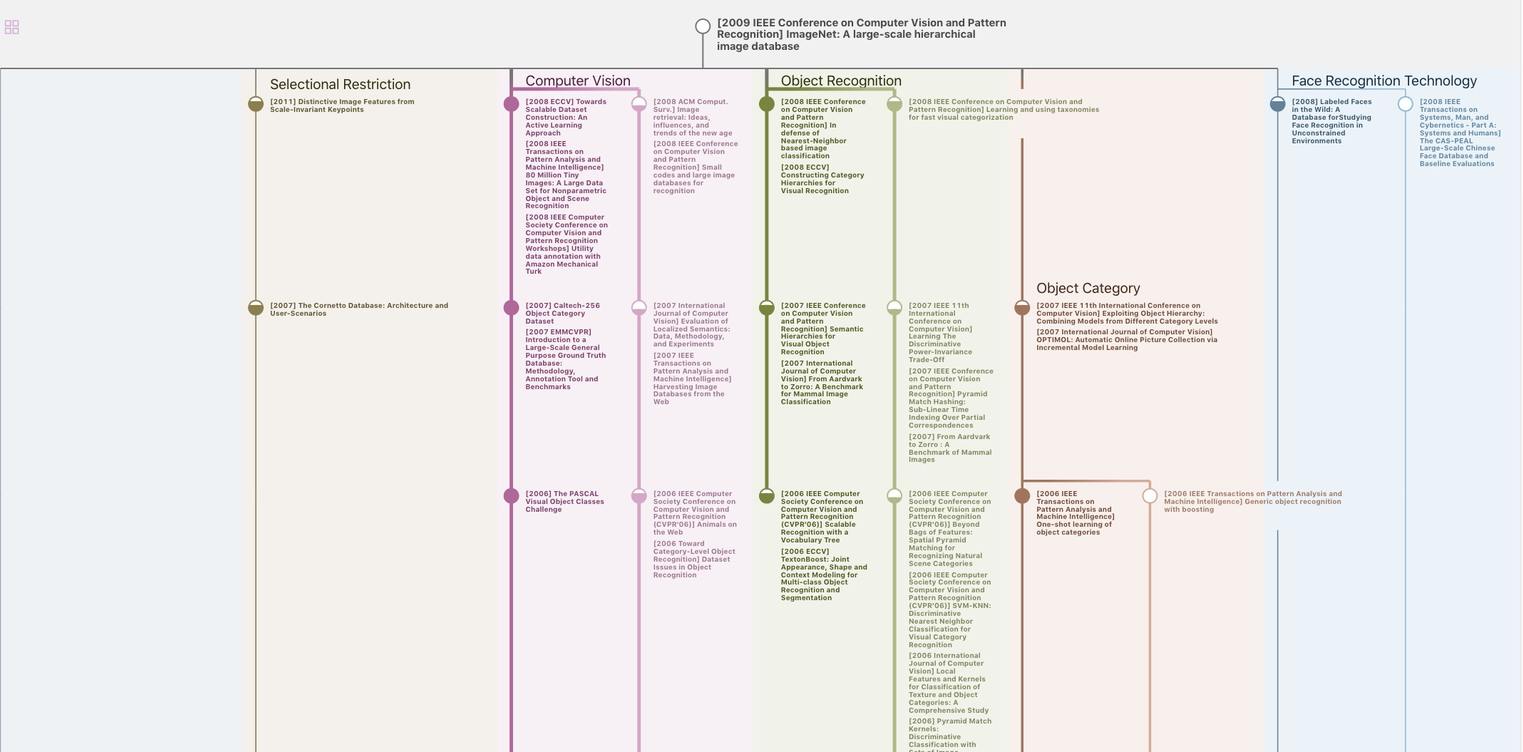
生成溯源树,研究论文发展脉络
Chat Paper
正在生成论文摘要