Beyond risk parity – A machine learning-based hierarchical risk parity approach on cryptocurrencies
Finance Research Letters(2021)
摘要
It has long been known that estimating large empirical covariance matrices can lead to very unstable solutions, with estimation errors more than offsetting the benefits of diversification. In this study, we employ the Hierarchical Risk Parity approach, which applies state-of-the-art mathematics including graph theory and unsupervised machine learning to a large portfolio of cryptocurrencies. An out-of-sample comparison with traditional risk-minimization methods reveals that Hierarchical Risk Parity outperforms in terms of tail risk-adjusted return, thereby working as a potential risk management tool that can help cryptocurrency investors to better manage portfolio risk. The results are robust to different rebalancing intervals, covariance estimation windows and methodologies.
更多查看译文
关键词
Machine learning,Graph theory,Hierarchical tree clustering,Asset allocation,Cryptocurrencies
AI 理解论文
溯源树
样例
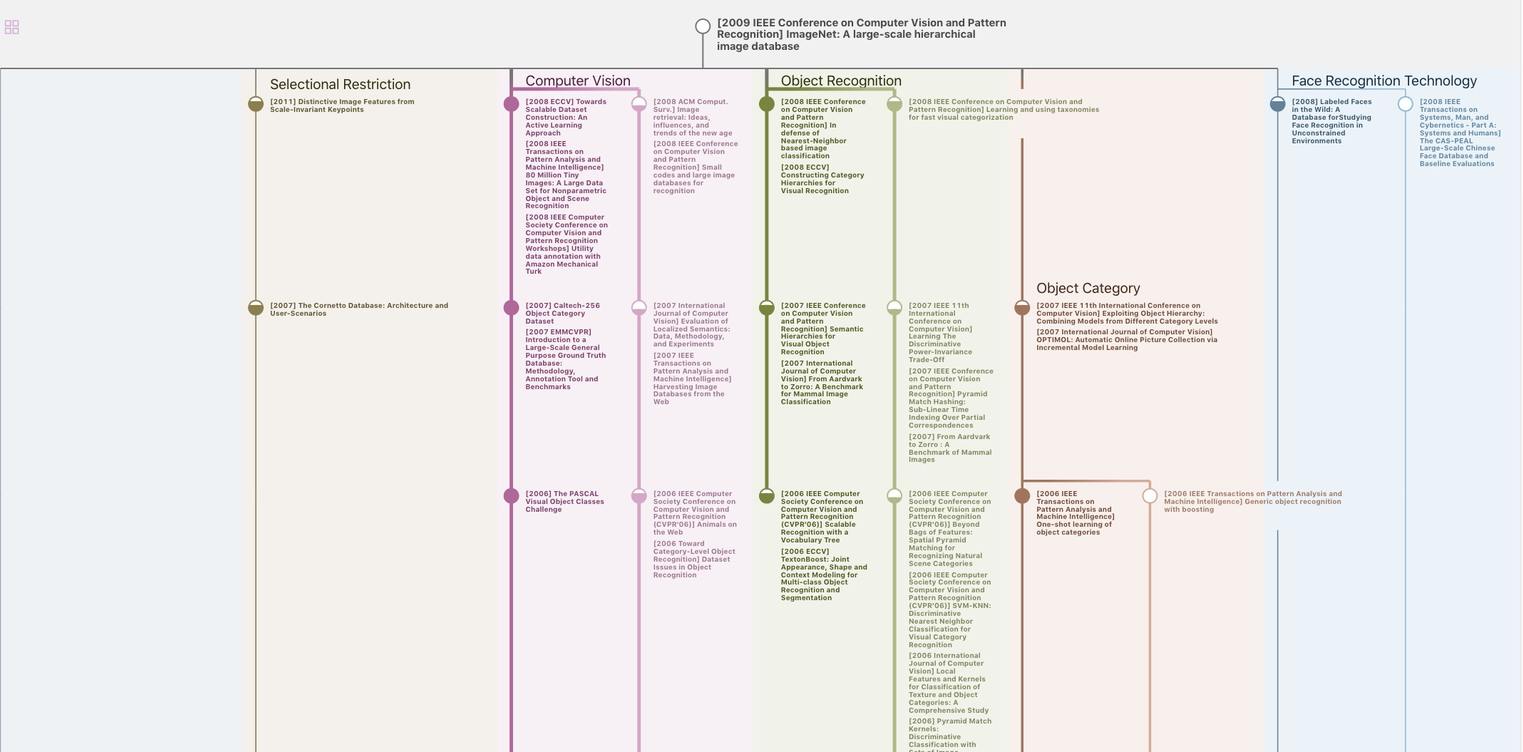
生成溯源树,研究论文发展脉络
Chat Paper
正在生成论文摘要