The Method of Classifying Fog Level of Outdoor Video Images Based on Convolutional Neural Networks
JOURNAL OF THE INDIAN SOCIETY OF REMOTE SENSING(2021)
摘要
The outdoor video has become an important mean of monitoring on-site and target recognition recently, which requires higher and higher quality of the outdoor video images. Foggy weather can cause a significant drop in the quality of outdoor video images, directly affecting the accuracy of monitoring on-site and target recognition. In order to automatically select high-quality images with no fog or mist from the outdoor video, a method of fog level classification in outdoor video images based on convolutional neural networks (CNN) is proposed in this paper. A type of CNN with three convolutional layers, three pooling layers, and three fully connected layers is constructed, and the parameters such as the convolution kernel size, pooling kernel size and padding size are adjusted to obtain the optimal network type. The network can automatically learn the fog concentration characteristics in the image, and can effectively divide the image into three levels: fog-free, mist and dense fog. The method is compared with K-means clustering, support vector machine, and random forest classification. The results show that the constructed CNN has the highest accuracy for fog classification, which can reach more than 99%. Nevertheless, the accuracies of K-means clustering, support vector machine (SVM), and random forest classification are 85.60%, 81.48%, and 89.48%, respectively. With the CNN model constructed in this study for fog level classification, high-quality images with no fog or mist can be automatically selected for outdoor video image analysis and applications.
更多查看译文
关键词
CNN, Outdoor video image, Fog level classification, K-means clustering, SVM, Random forest classification
AI 理解论文
溯源树
样例
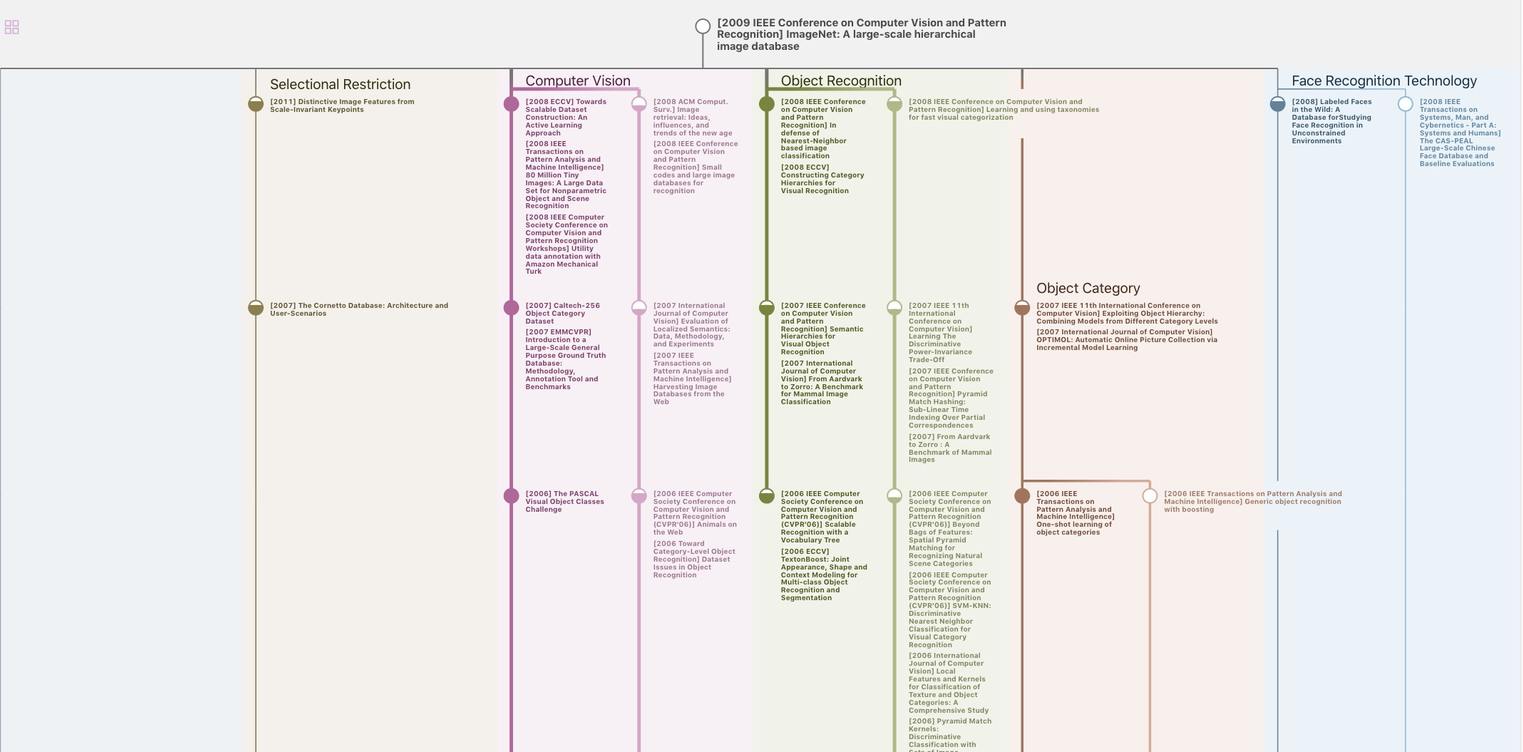
生成溯源树,研究论文发展脉络
Chat Paper
正在生成论文摘要