Zem: Zero-Cycle Bit-Masking Module For Deep Learning Refresh-Less Dram
IEEE ACCESS(2021)
摘要
In sub-20 nm technologies, DRAM cells suffer from poor retention time. With the technology scaling, this problem tends to be worse, significantly increasing refresh power of DRAM. This is more problematic in memory heavy applications such as deep learning systems, where a large amount of DRAM is required, DRAM refresh power contributes to a considerable portion of total system power. With the growth in deep learning workloads, this is set to get worse. In this work, we present a zero-cycle bit-masking (ZEM) scheme that exploits the asymmetry of retention failures, to eliminate DRAM refresh in the inference of convolution neural networks, natural language processing, and the image generation based on generative adversarial network. Through careful analysis, we derive a bit-error-rate (BER) threshold that does not affect the accuracy of inference. Our proposed architecture, along with the techniques involved, are applicable to all types of DRAMs. Our results on 16Gb devices show that ZEM can improve the performance by up to 17.31% while reducing the total energy consumed by DRAM by up to 43.03%, dependent on the type of DRAM.
更多查看译文
关键词
Random access memory, Deep learning, Encoding, Task analysis, Memory management, Capacitors, Power demand, DNNs, inference, encoding, decoding, refresh-less DRAM
AI 理解论文
溯源树
样例
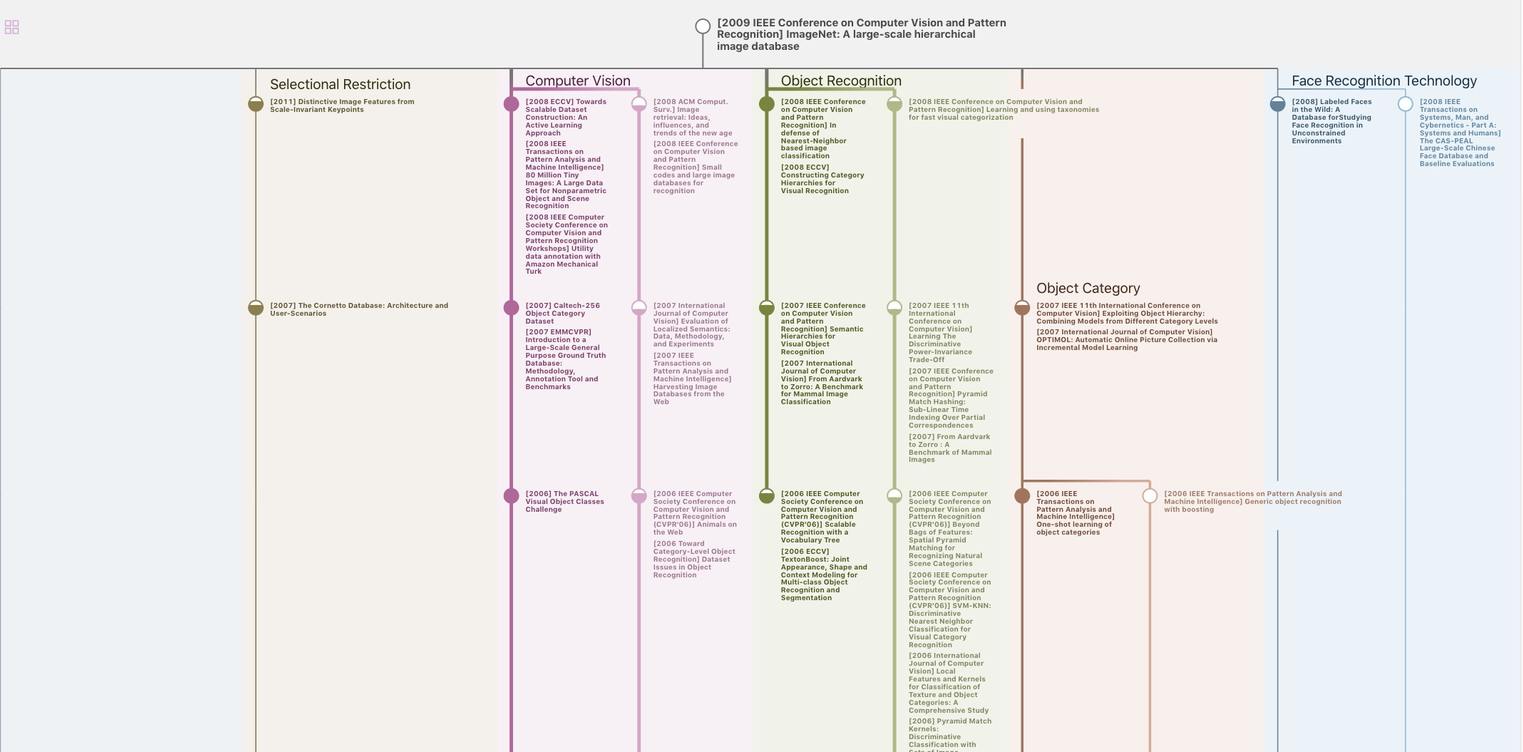
生成溯源树,研究论文发展脉络
Chat Paper
正在生成论文摘要