A Method For Predicting Hobbing Tool Wear Based On Cnc Real-Time Monitoring Data And Deep Learning
PRECISION ENGINEERING-JOURNAL OF THE INTERNATIONAL SOCIETIES FOR PRECISION ENGINEERING AND NANOTECHNOLOGY(2021)
摘要
Intelligent monitoring and diagnosis of tool status are of great significance for improving the manufacturing efficiency and accuracy of the workpiece. It is difficult to quickly and accurately predict the wear state of worm gear hob under different working conditions. This paper proposes a novel approach to predict hob wear status based on CNC real-time monitoring data. Based on the open platform communication unified architecture (OPC UA) technology and orthogonal test, the machine data of motor power, current, etc. related to tool wear are collected online in the worm gear machining process. And then, an improved deep belief network (DBN) is used to generate a tool wear model by training data. A growing DBN with transfer learning is introduced to automatically decide its best model structure, which can accelerate its learning process, improve training efficiency and model performance. The experiment results show that the proposed method can effectively predict hob wear status under multi-cutting conditions. To show the advantages of the proposed approach, the performance of the DBN is compared with the traditional back propagation neural network (BP) method in terms of the mean squared error (MSE). The compared results show that this tool wear prediction method has better prediction accuracy than the traditional BP method during worm gear hobbing.
更多查看译文
关键词
Tool wear, OPC UA, Deep belief network, Worm gear hobbing, Transfer learning
AI 理解论文
溯源树
样例
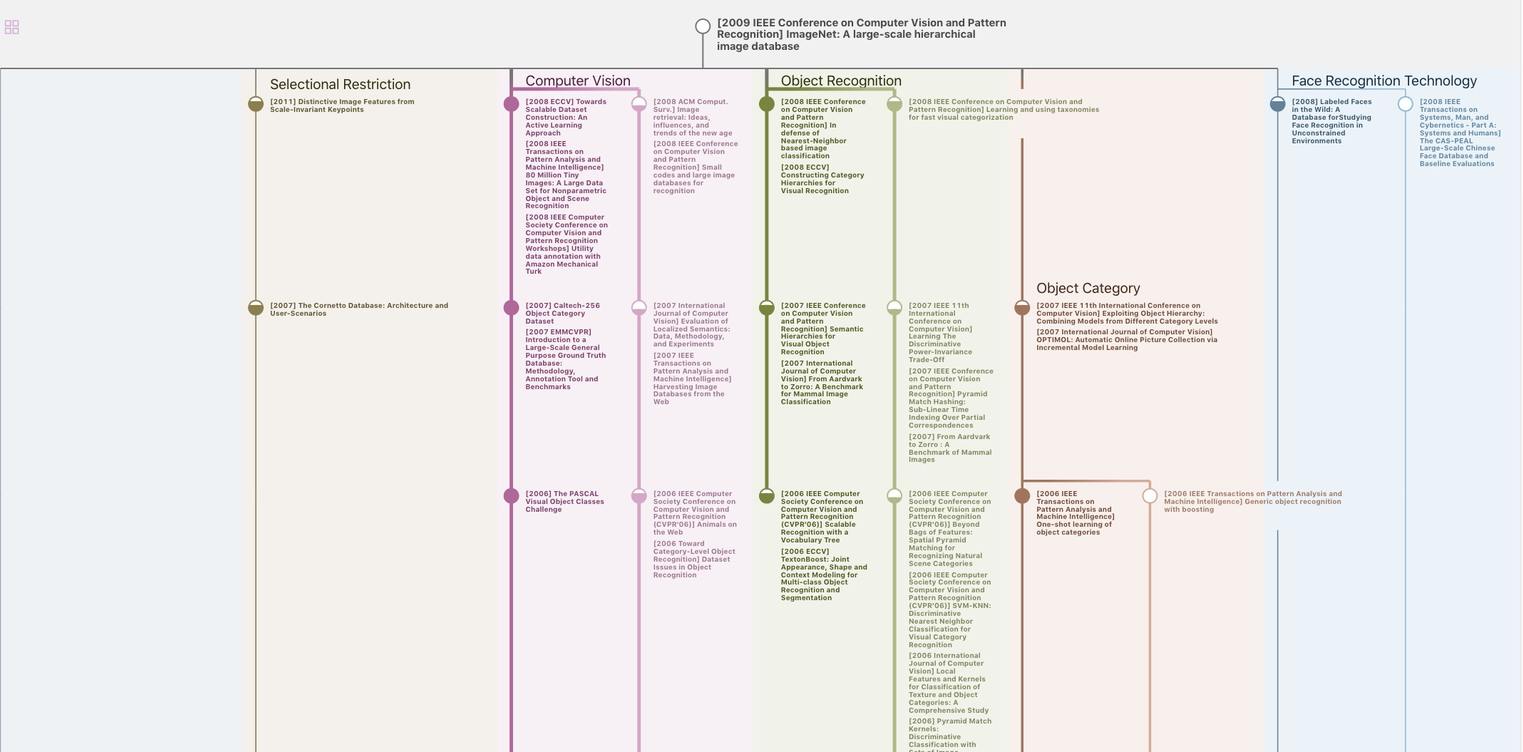
生成溯源树,研究论文发展脉络
Chat Paper
正在生成论文摘要