Kinetics Of Perovskite Degradation: Machine Learning With Physics And Chemistry Inspired Features
2021 IEEE 48TH PHOTOVOLTAIC SPECIALISTS CONFERENCE (PVSC)(2021)
摘要
Understanding the rate of degradation of perovskite films is essential for achieving long-term stability and for developing predictions that can provide a basis for device performance warranties. We focus on understanding and predicting the rate of change of material level properties of absorbance (A), quantitative photoluminescence (PL), and carrier diffusion length (Ld) in perovskite films. An essential aspect of our recent progress hinges on the development of physics-inspired and chemistry-inspired features that are incorporated into machine learning models for A-T80, PL-T80, and Ld-T80 (the time elapsed for a property to degrade to 80% of its initial value), each as a function of temperature, oxygen, humidity, and illumination intensity. The models are based on over 100 degradation experiments where A, PL, and Ld are determined via in-situ measurements in an environmental chamber. Using "Good Ole-Fashioned Human Intelligence," we have developed a full kinetic rate equation (with rate orders, rate constants, and activation energies) for the degradation of MAPbI3 that accurately predict the initial rate of material transformation as a function of oxygen, humidity, illumination, and temperature. Machine learning methods are then developed that utilize these engineered features to carry-out accurate predictions for A-T80, PL-T80, and Ld-T80.
更多查看译文
关键词
perovskite, machine learning, accelerated testing
AI 理解论文
溯源树
样例
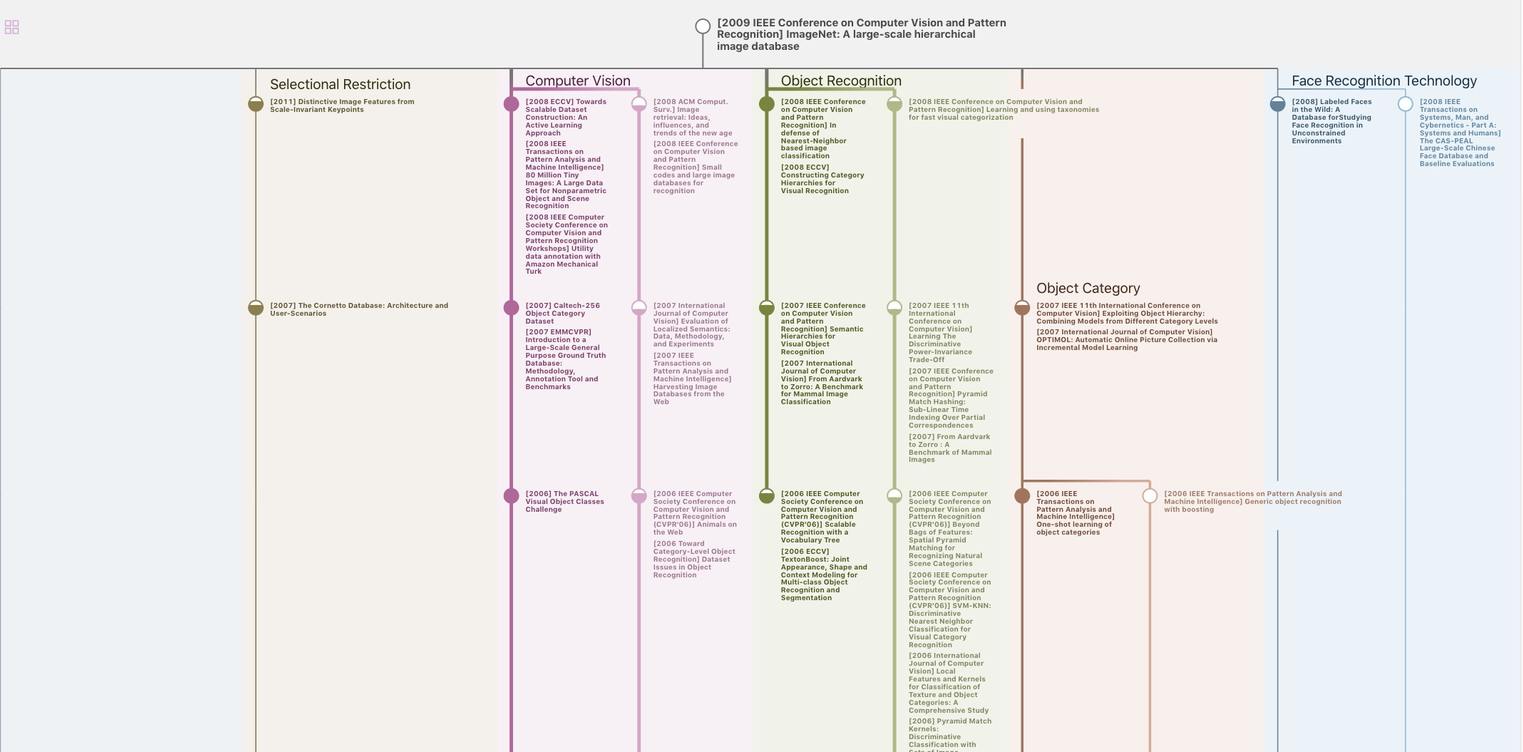
生成溯源树,研究论文发展脉络
Chat Paper
正在生成论文摘要