Understanding CNN based Anthropomorphic Model Observer using Classification Images
MEDICAL IMAGING 2021: IMAGE PERCEPTION, OBSERVER PERFORMANCE, AND TECHNOLOGY ASSESSMENT(2021)
摘要
It is widely accepted that medical imaging reconstruction strategies should be optimized by maximizing the chance of a radiologist making a correct diagnostic. This implies organizing costly sessions with doctors to evaluate the images and provide feedback. Model Observers (MO) are algorithms designed to act as human surrogates in evaluating and providing feedback as a measure of diagnostic accuracy, and should be tuned to make the same diagnostic as the human's, regardless of the correctness. In this work, we use a previously trained and optimized Convolutional Neural Network (CNN) based MO to construct classification images that show how the diagnostic information is accessed by the MO in a form of a perceptual filter. A single MO was trained for a forced-localization task in simulated data with three different power-law noise backgrounds representing different levels of background variability. The classification images are computed in the same way as they would for a human observer using 10,000 simulated images with a defect. The frequency profile of the MO classification images show that frequency weights appear band-pass in nature and highly correlated to the frequency weights from the human observer classification images.
更多查看译文
关键词
Model observer,medical image quality assessment,machine learning,deep learning,CNN,U-Net
AI 理解论文
溯源树
样例
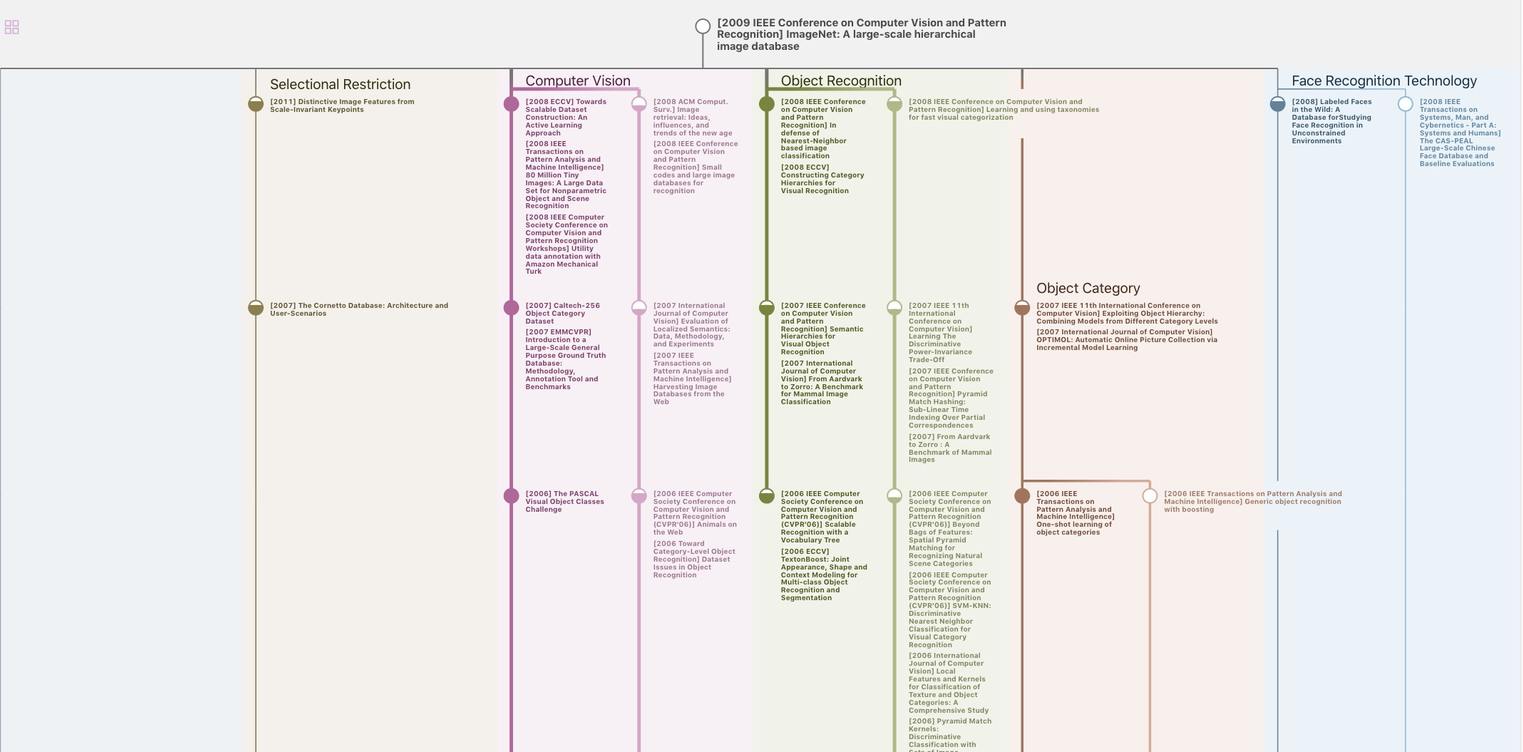
生成溯源树,研究论文发展脉络
Chat Paper
正在生成论文摘要