Dataset Reduction for Neural Network Based Digital Predistorters under Strong Nonlinearities
2021 IEEE Topical Conference on RF/Microwave Power Amplifiers for Radio and Wireless Applications (PAWR)(2021)
摘要
The artificial neural networks (ANN) are gaining momentum in the digital predistorters (DPD) thanks to their inherently good approximation capabilities. Under strong or complex power amplifier nonlinearities, the size of the ANN can increase and lead to long training periods which are unaffordable in fast-changing waveform scenarios like those proposed for 5G or 6G. In this work we combine the orthogonal matching pursuit technique together with dataset length reduction methods, to significantly shorten the ANN DPD coefficients update time.
更多查看译文
关键词
power amplifiers,predistortion,iterative learning control,machine learning algorithms,artificial neural networks
AI 理解论文
溯源树
样例
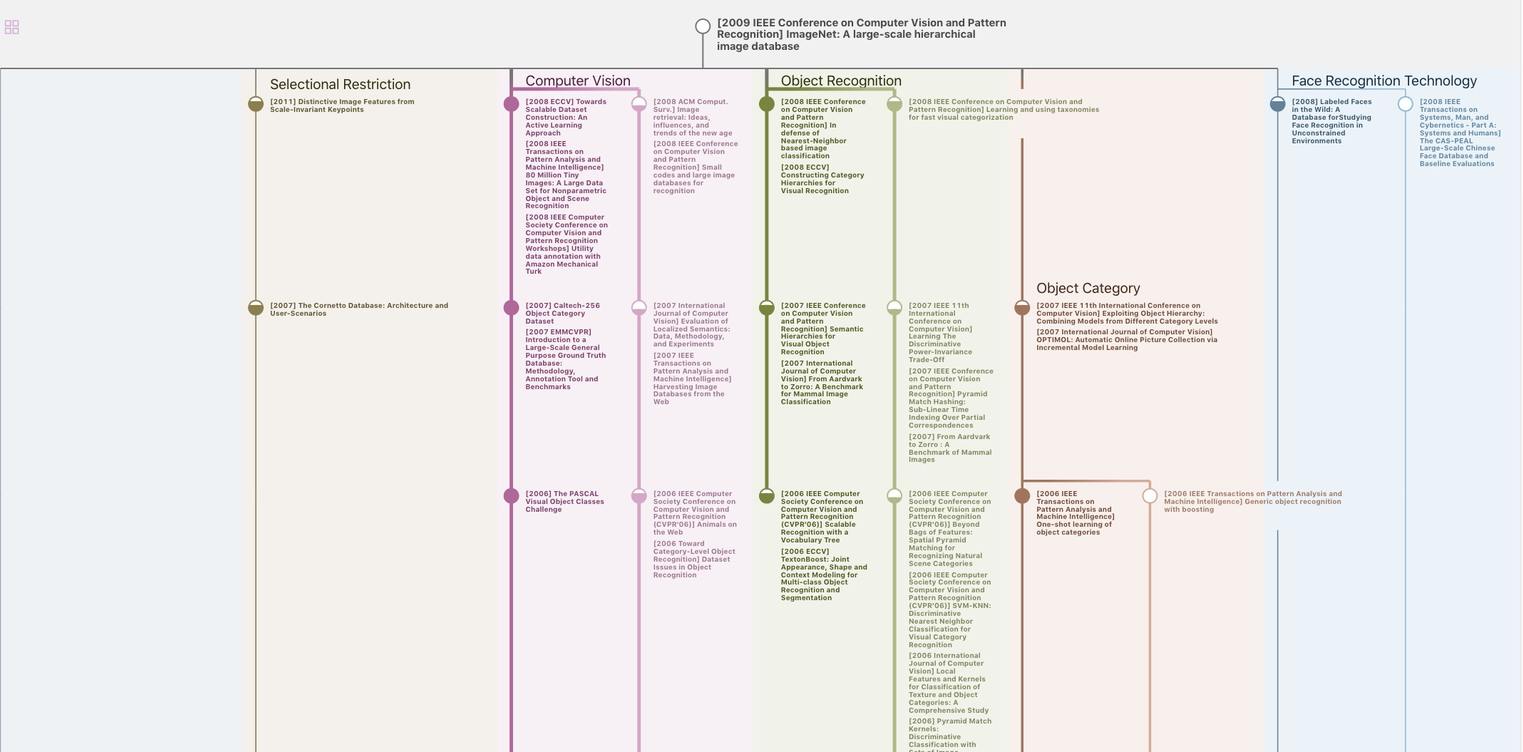
生成溯源树,研究论文发展脉络
Chat Paper
正在生成论文摘要