Robust Index Of Confidence Weighted Learning For Optimal Individualized Treatment Rule Estimation
STAT(2021)
摘要
Determination of optimal individual treatment rules (ITR) is a rapidly growing area in precision medicine; various parametric and non-parametric methods have been proposed. Existing methods, however, focus on the mean outcome and thus are sensitive to outliers, skewed and heavy-tailed outcome distributions. In this paper, we propose an optimal ITR estimation framework using a weighted classifier with robust weights based on measures of similarity. Compared to previous methods in the literature, this two-stage nonparametric model is novel and enjoys several advantages. First, due to its non-parametric nature, it is more flexible than regression-based parametric and semi-parametric models. Second, the similarity-based confidence index is essentially a weighted sum of indicator functions depending on the sign of pairwise outcome differences; therefore, it is robust to outliers, skewed and heavy-tailed outcome distributions. The performance of the proposed approach is demonstrated via simulation studies and an analysis of data from a randomized clinical trial for depression.
更多查看译文
关键词
biostatistics, nonparametric methods, classification
AI 理解论文
溯源树
样例
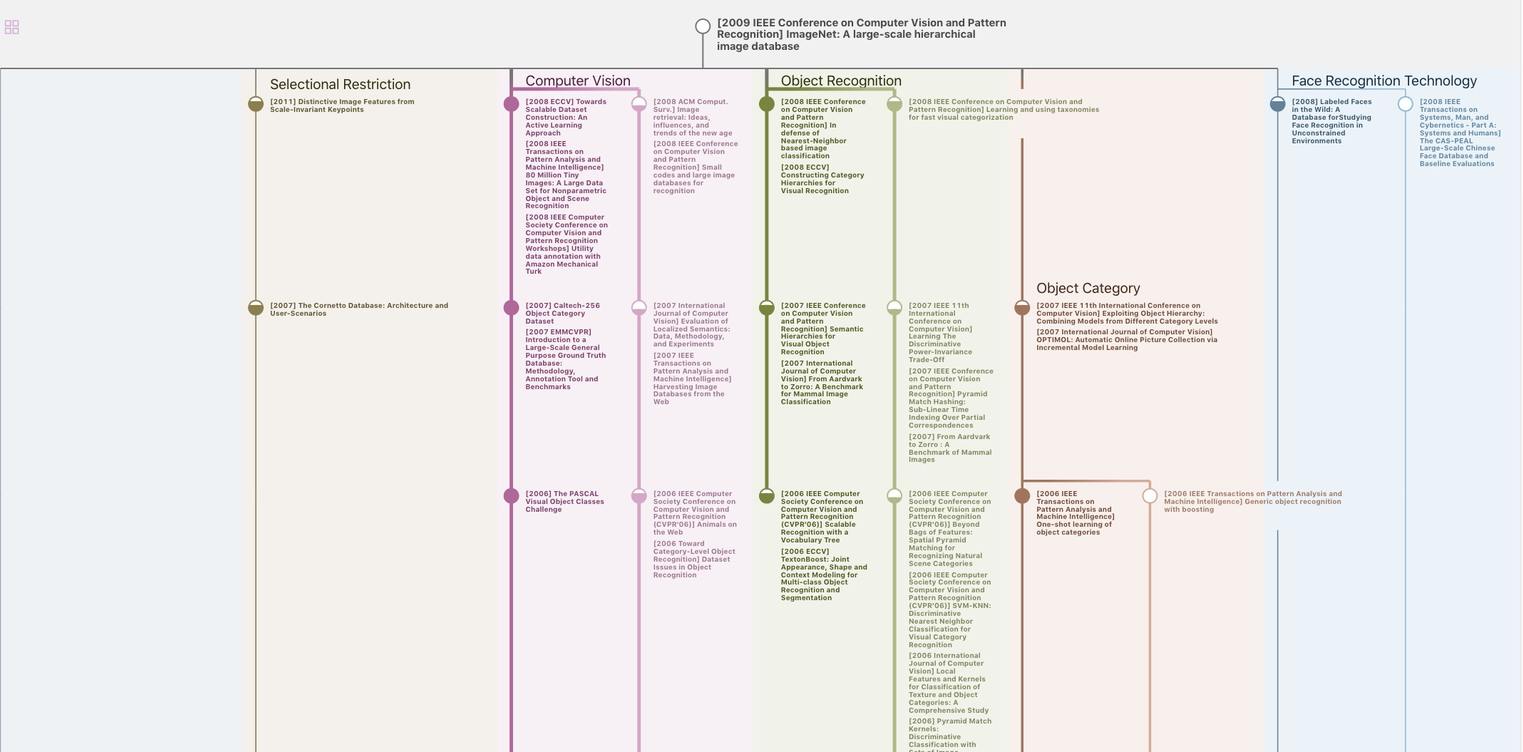
生成溯源树,研究论文发展脉络
Chat Paper
正在生成论文摘要