Looking For Twins: How To Build Better Counterfactuals With Matching
ITALIAN POLITICAL SCIENCE REVIEW-RIVISTA ITALIANA DI SCIENZA POLITICA(2021)
摘要
A primary challenge for researchers that make use of observational data is selection bias (i.e. the units of analysis exhibit systematic differences and dis-homogeneities due to non-random selection into treatment). This article encourages researchers in acknowledging this problem and discusses how and more importantly - under which assumptions they may resort to statistical matching techniques to reduce the imbalance in the empirical distribution of pre-treatment observable variables between the treatment and control groups. With the aim of providing a practical guidance, the article engages with the evaluation of the effectiveness of peacekeeping missions in the case of the Bosnian civil war, a research topic in which selection bias is a structural feature of the observational data researchers have to use, and shows how to apply the Coarsened Exact Matching (CEM), the most widely used matching algorithm in the fields of Political Science and International Relations.
更多查看译文
关键词
causation, coarsened exact matching, peacekeeping, selection bias, statistical matching
AI 理解论文
溯源树
样例
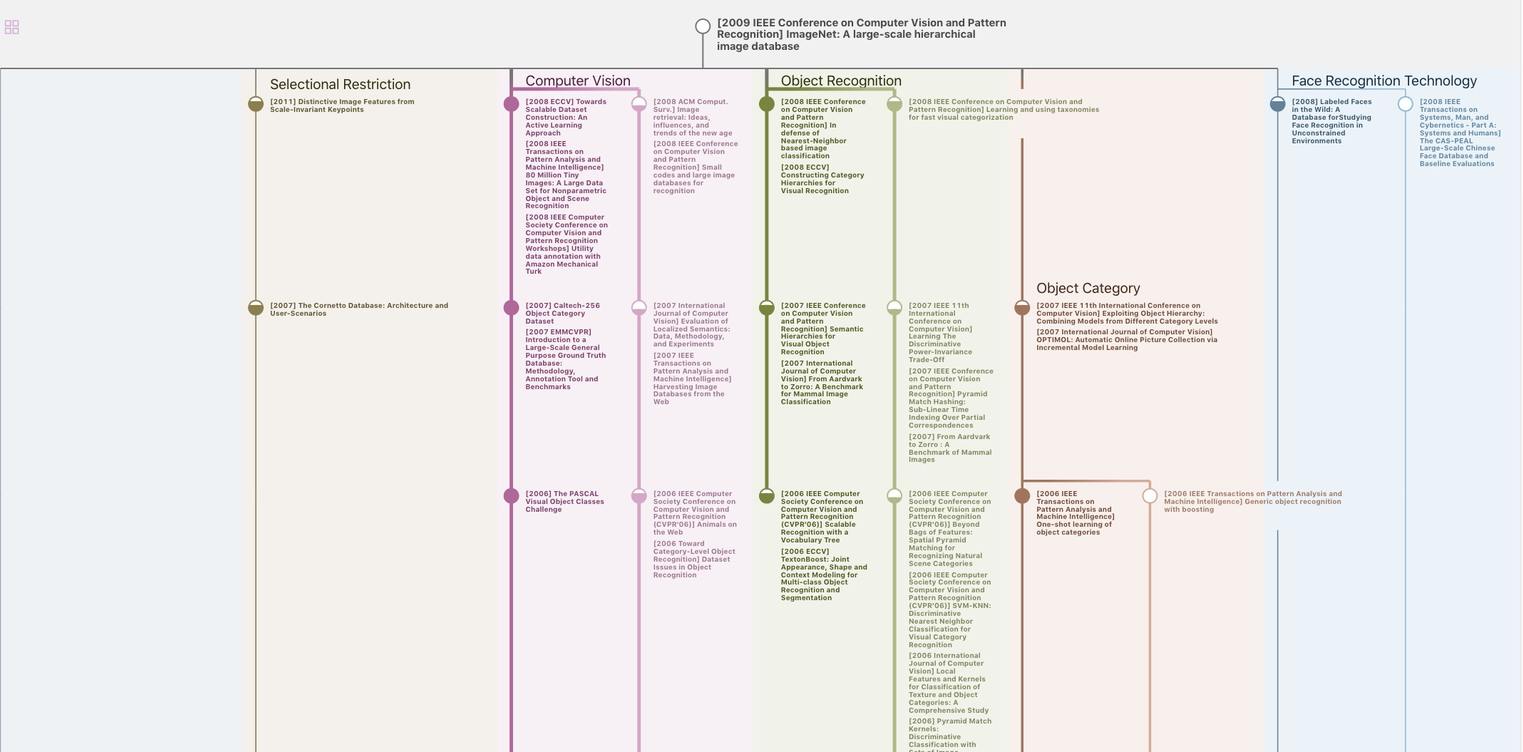
生成溯源树,研究论文发展脉络
Chat Paper
正在生成论文摘要