3d Convolution For Multidate Crop Recognition From Multitemporal Image Sequences
INTERNATIONAL JOURNAL OF REMOTE SENSING
摘要
The increasing food demand is regarded as the main threat to nature today. In this scenario, Remote Rensing is an essential technology to assess and monitor the extent and productivity of cultivated land. However, most work on crop mapping from remote sensing imagery so far focused on temperate areas, where a single crop prevails throughout the year. This paper explores different Convolutional Neural Network architectures for multi-temporal crop recognition in tropical areas, with highly complex crop dynamics and many crops per year. The proposed models are a U-Net with bidirectional ConvLSTM added to its skip connections, a U-Net with 3D convolutions, and a U-Net with 3D convolutions and Atrous Temporal Pyramid Pooling. The paper also assesses the gain of a post-processing step based on fully connected conditional random fields (CRF) as a second look at the spatial context to improve the networks' predictions. In an extensive experimental analysis conducted on two public multitemporal datasets of tropical areas with complex crop dynamics, all proposed models achieved better results than state-of-the-art methods based on recurrent networks in terms of Overall Accuracy and Average F1-Score. The 3D convolution with the Atrous Temporal Pyramid Pooling model stood up as the best performing architecture, with gains up to 4.3% over the baseline. The proposed CRF post-processing also proved beneficial for all tested architectures, bringing improvements of up to 2.7% in terms of average F1-score.
更多查看译文
AI 理解论文
溯源树
样例
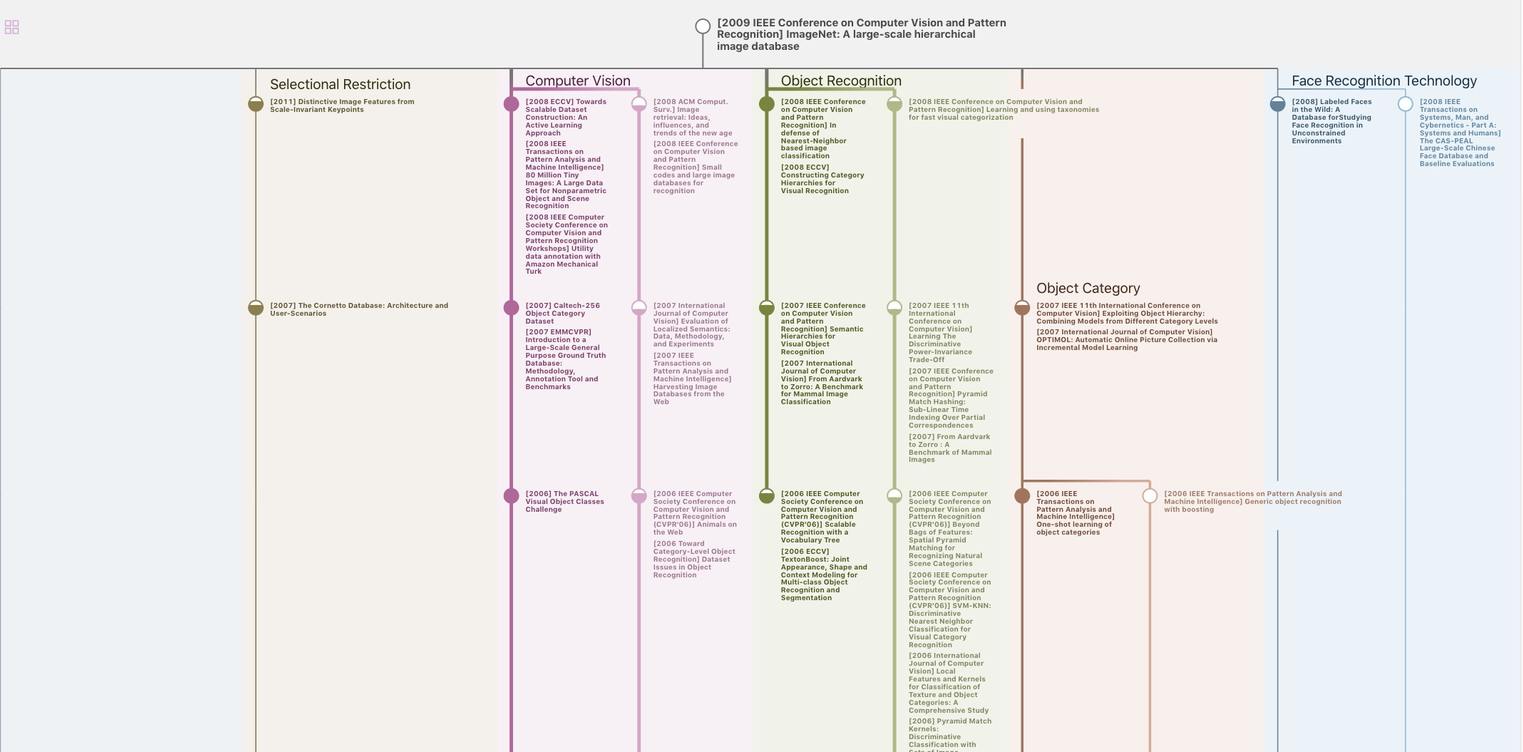
生成溯源树,研究论文发展脉络
Chat Paper
正在生成论文摘要