Using stratified Bayesian model averaging in probabilistic forecasts of precipitation over the middle and lower Yangtze River region
METEOROLOGY AND ATMOSPHERIC PHYSICS(2021)
摘要
To study the impact of training sample heterogeneity on the performance of Bayesian model averaging (BMA), two BMA experiments were performed on probabilistic quantitative precipitation forecasts for the middle and lower reaches of the Yangtze River in summer 2016 using TIGGE (THORPEX Interactive Grand Global Ensemble) multi-model and multi-member precipitation forecast data. The first experiment, conventional Bayesian model averaging (CBMA), pools the subsamples together in training and forecasting without categorisation. The second experiment, stratified sampling BMA (SBMA), divides all available training samples into three subsets according to their ensemble spread. The results show that spread is a good indicator to divide the ensemble precipitation samples into subsets, especially for heavy rainfall. Moreover, the optimal training period for BMA is about 50 days. The CBMA often filters out most information on the high spread cases and produces ill-fitted parameters for them. Additionally, CBMA deterministic forecast and percentile forecast are often underestimated. In contrast to CBMA, parameters estimated using SBMA with stratified samples of different properties are much more reasonable. The mean absolute error, continuous ranked probability scores and Brier scores of the two BMA experiments were examined for all available forecast cases. Although CBMA was slightly superior to the raw ensemble prediction system (REPS) forecasts in low-threshold forecasts, SBMA outperformed REPS, probability-matching ensemble mean, and CBMA in all evaluations.
更多查看译文
AI 理解论文
溯源树
样例
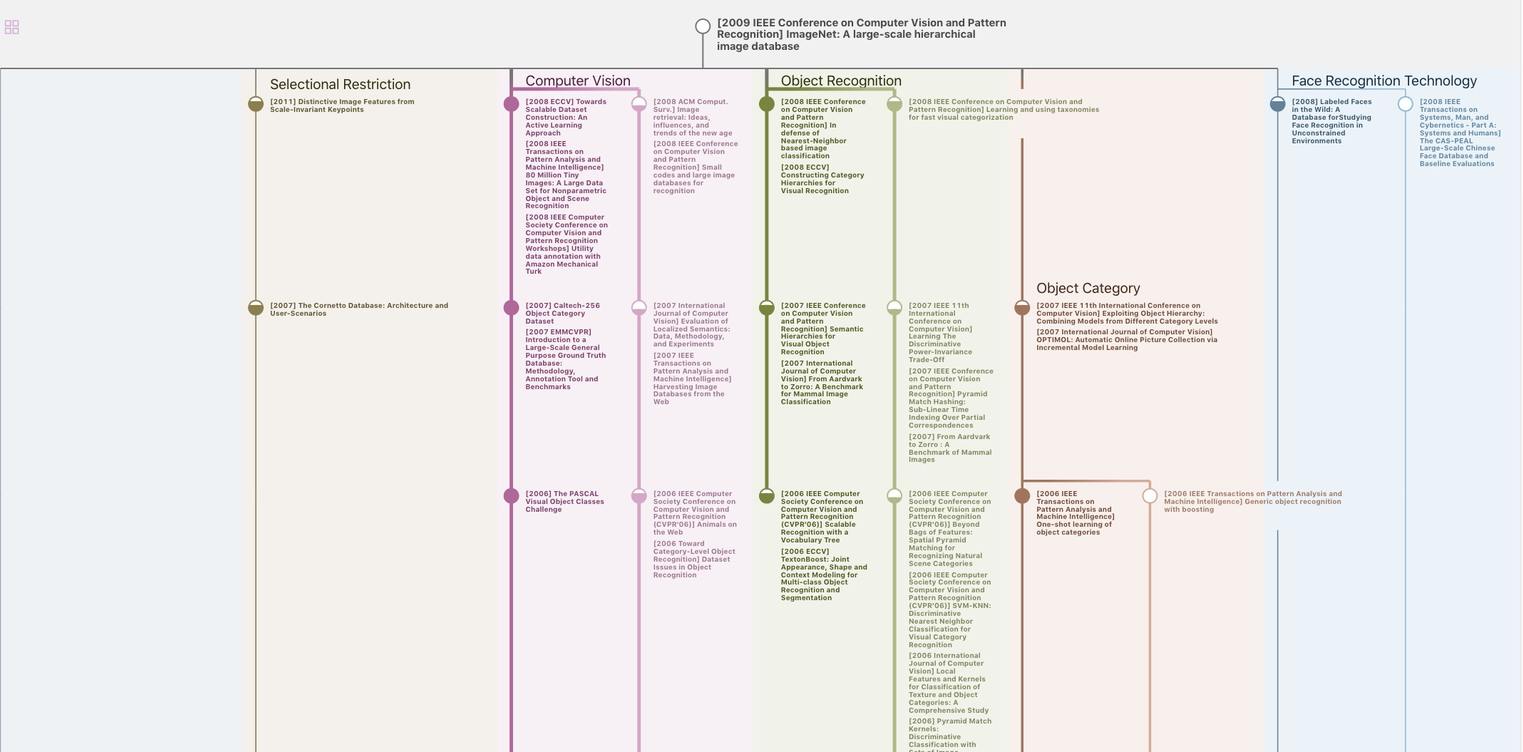
生成溯源树,研究论文发展脉络
Chat Paper
正在生成论文摘要