Transistor Compact Model Based on Multigradient Neural Network and Its Application in SPICE Circuit Simulations for Gate-All-Around Si Cold Source FETs
IEEE Transactions on Electron Devices(2021)
摘要
Transistor compact model (TCM) is the key bridge between process technology and circuit design. Typically, TCM is desired to capture the nonlinear device electronic characteristics and their high-order derivatives. However, for the novel devices in advanced and future technologies, establishing TCM based on analytical equations and extracting model parameters becomes tedious. The model fitting capability for device outputs’ high-order derivatives is also limited. These drawbacks hinder fast and accurate device to circuit evaluation cycles. We develop a TCM based on multigradient neural network (MNN) using computational graph in the PyTorch framework. This MNN model is able to simultaneously capture the transistor dc/ac characteristics, such as
${I}-{V}/{Q}-{V}$
, their derivatives (
${G}-{V}/{C}-{V}$
), and higher order derivatives accurately. Moreover, the model architecture can be widely adapted to various device types. Based on this model scheme, software is developed to enable the automated model generation for standard SPICE simulation. Finally, the model and software are validated for novel gate-all-around (GAA) Si cold source field-effect transistors (CSFET), and 19-stage ring oscillator and two-stage operational amplifier circuit simulations have also been demonstrated. This work reduces the cycle of novel device compact model creation and circuit benchmark simulation from months or weeks to hours. In addition, it enables more precise circuit simulation for analog and RF circuits, and it provides a rapid solution for early stage design technology cooptimization (DTCO).
更多查看译文
关键词
Circuit simulation,device compact model,field-effect transistor (FET),multigradient neural network (MNN)
AI 理解论文
溯源树
样例
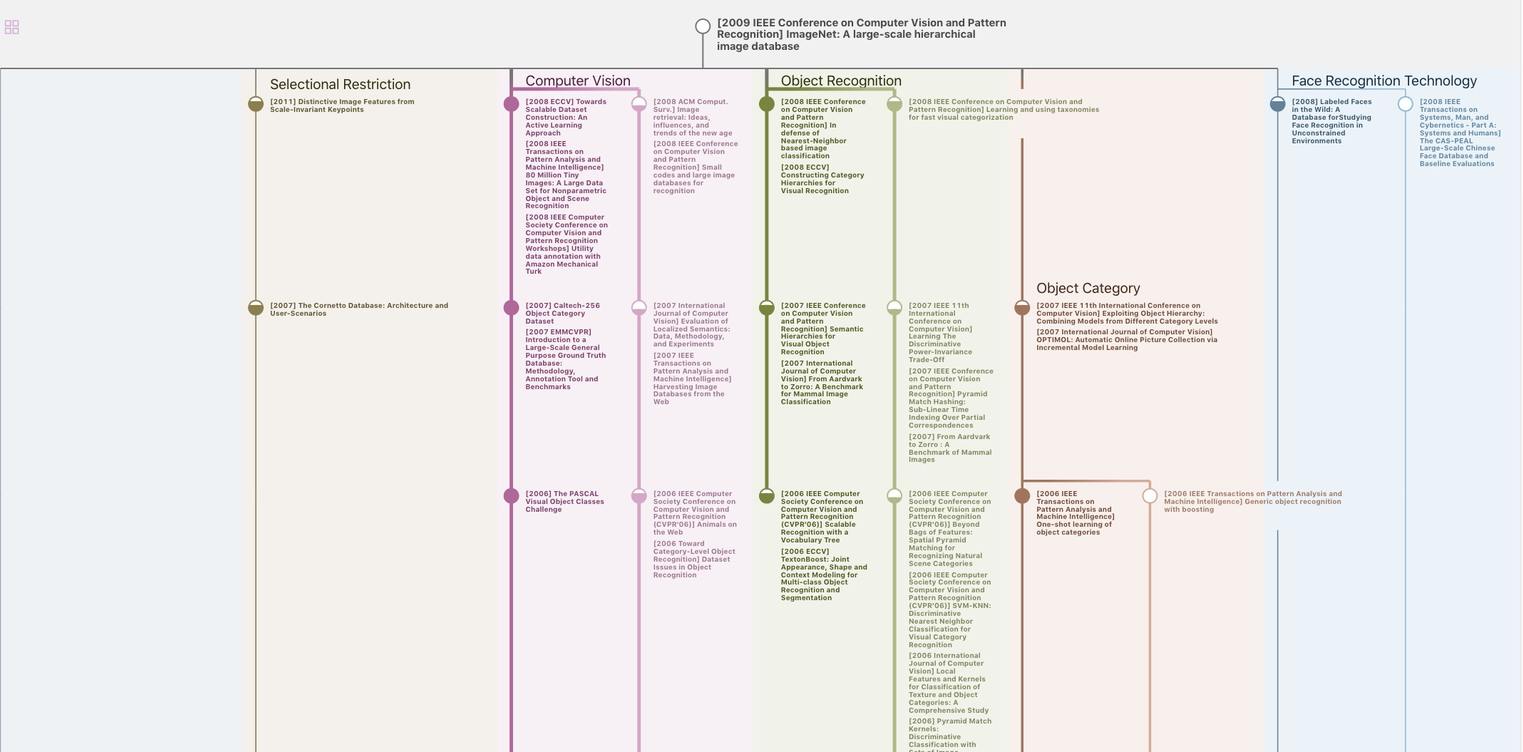
生成溯源树,研究论文发展脉络
Chat Paper
正在生成论文摘要