Research on Rolling Bearing Fault Diagnosis Method Based on Improved LMD and CMWPE
JOURNAL OF FAILURE ANALYSIS AND PREVENTION(2021)
摘要
To enhance the precision of rolling bearing fault diagnosis, a new rolling bearing fault diagnosis method based on improved local mean decomposition (LMD), compound multi-scale weighted permutation entropy (CMWPE), and support vector machine (SVM) is proposed. Firstly, the improved LMD algorithm is adopted to accomplish the adaptive decomposition of rolling bearing vibration signals. By computing the Pearson correlation coefficients between each component and the initial signal, the components with higher correlation are selected for signal reconstruction to accomplish the mission of noise reduction. Then, a feature extraction approach based on CMWPE is employed to extract corresponding feature parameters from the de-noised signals and construct a multi-scale nonlinear fault feature set with good stability and high recognition. Finally, the high-dimensional fault feature set is input into the SVM to achieve rolling bearing fault diagnosis. The experimental results reveal that the proposed approach can precisely distinguish various fault types of rolling bearings under the same fault degrees. For inner ring failures of different fault degrees, this method also has good identification correctness. Compared with several typical fault diagnosis approaches, the proposed method has a more trustworthy diagnosis result.
更多查看译文
关键词
Rolling bearing, Local mean decomposition, Composite multi-scale weighted permutation entropy, Support vector machine, Fault diagnosis
AI 理解论文
溯源树
样例
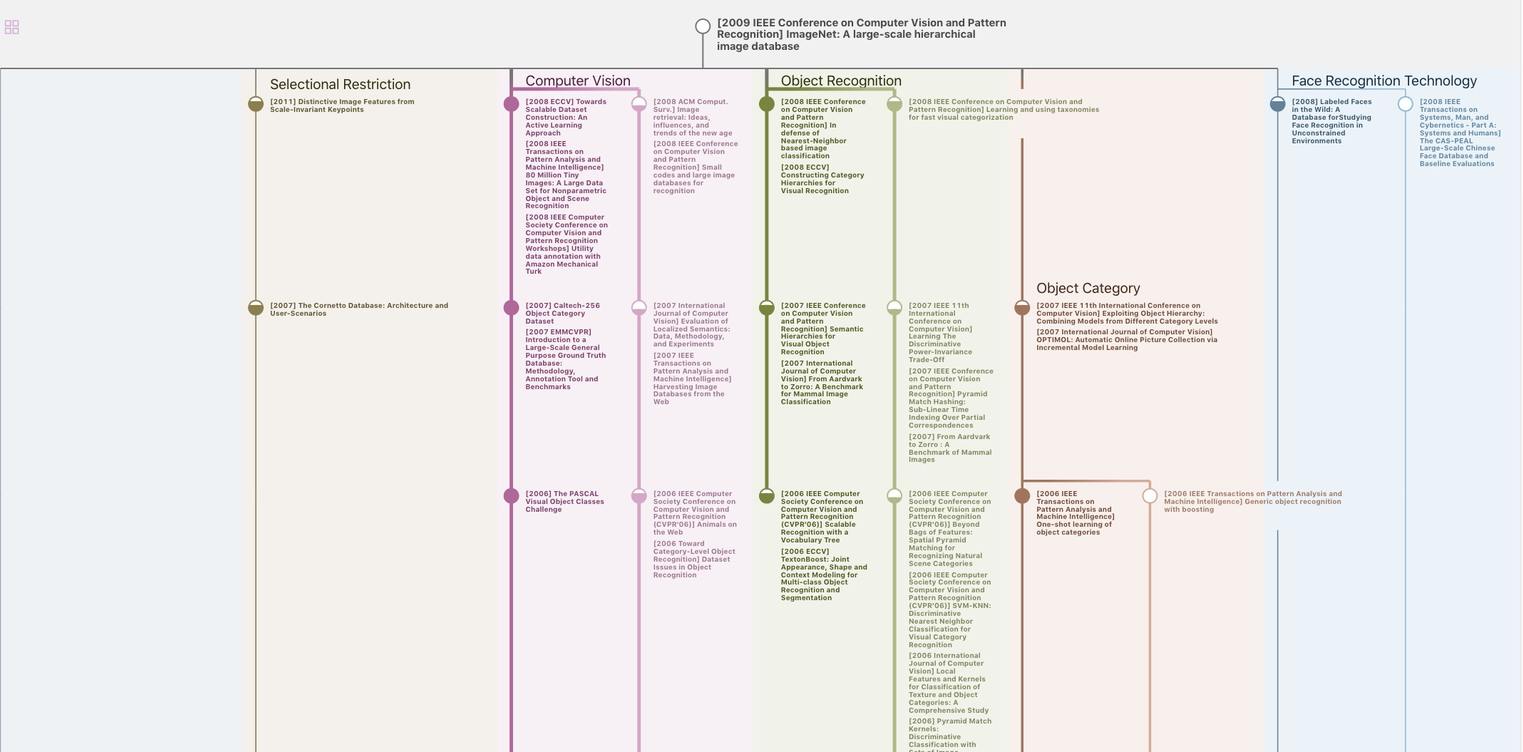
生成溯源树,研究论文发展脉络
Chat Paper
正在生成论文摘要