Q-Learnheuristics: Towards Data-Driven Balanced Metaheuristics
MATHEMATICS(2021)
摘要
One of the central issues that must be resolved for a metaheuristic optimization process to work well is the dilemma of the balance between exploration and exploitation. The metaheuristics (MH) that achieved this balance can be called balanced MH, where a Q-Learning (QL) integration framework was proposed for the selection of metaheuristic operators conducive to this balance, particularly the selection of binarization schemes when a continuous metaheuristic solves binary combinatorial problems. In this work the use of this framework is extended to other recent metaheuristics, demonstrating that the integration of QL in the selection of operators improves the exploration-exploitation balance. Specifically, the Whale Optimization Algorithm and the Sine-Cosine Algorithm are tested by solving the Set Covering Problem, showing statistical improvements in this balance and in the quality of the solutions.
更多查看译文
关键词
metaheuristics, balanced metaheuristics, Q-Learning, Whale Optimization Algorithm, Sine-Cosine Algorithm
AI 理解论文
溯源树
样例
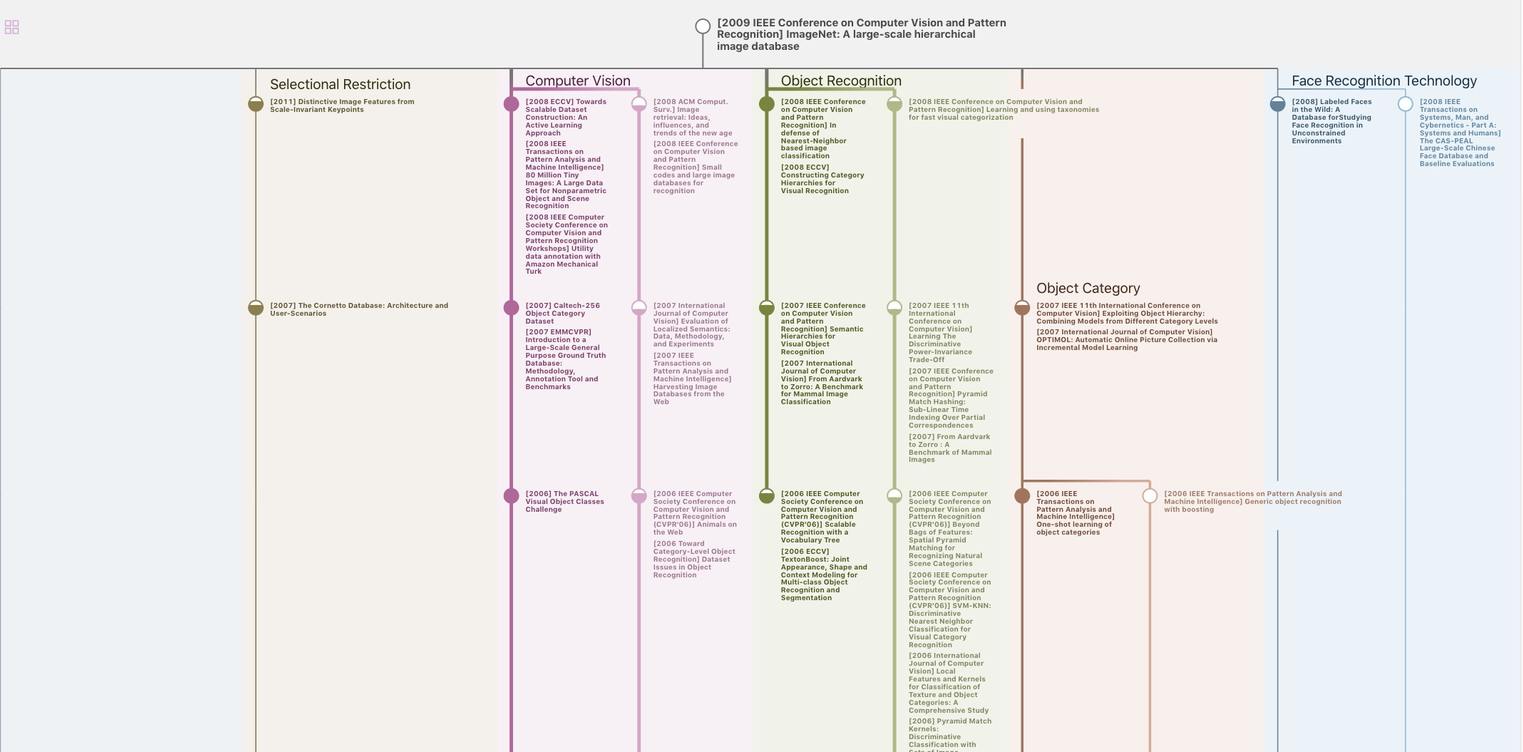
生成溯源树,研究论文发展脉络
Chat Paper
正在生成论文摘要