Detecting basic human activities and postural transition using robust machine learning techniques by applying dimensionality reduction methods
WAVES IN RANDOM AND COMPLEX MEDIA
摘要
In the whole world, approximately two billion people are using smartphones for their better life The smartphone's sensor data consist of high dimensions which increase complexity of training model and degrade overall performance of a classifier. We investigated and analyzed the performance of different machine learning algorithms with various dimensionality reduction techniques on publicly available smartphone-based recognition of human activities and postural transitions dataset. The highest accuracy was obtained at set 1 using support vector machine (SVM) linear kernel (97.40%) followed by set 3 using eXtreme boosting (XGBoost) (96.92%), set 2 using adaptive boosting multi-class (AdaBoost.M1) (95.69%), set 4 using AdaBoost. M1 (82.17%). Using different dimensionality reduction methods for set 1, principal component analysis (PCA) provided the highest accuracy using average neural network (AvNNET) (98.27%), while chi-squared gives the accuracy of 93.72% using the XGBoost classifier. For set 2, PCA gives the highest accuracy of 95.83% using the AvNNET classifier while for set 3 Chi-squared gives an accuracy of 84.46% using average neural network (AvNNET), SVM.L, and stochastic Gradient Boosting Machine (GBM) classifiers. The results reveal that overall dimensionality reduction methods outer performed. The outcomes will be helpful in making wise decisions in detecting various activities in anti-terrorists, surveillance, etc.
更多查看译文
关键词
Human activities, postural transition, machine learning classification, dimensionality reduction
AI 理解论文
溯源树
样例
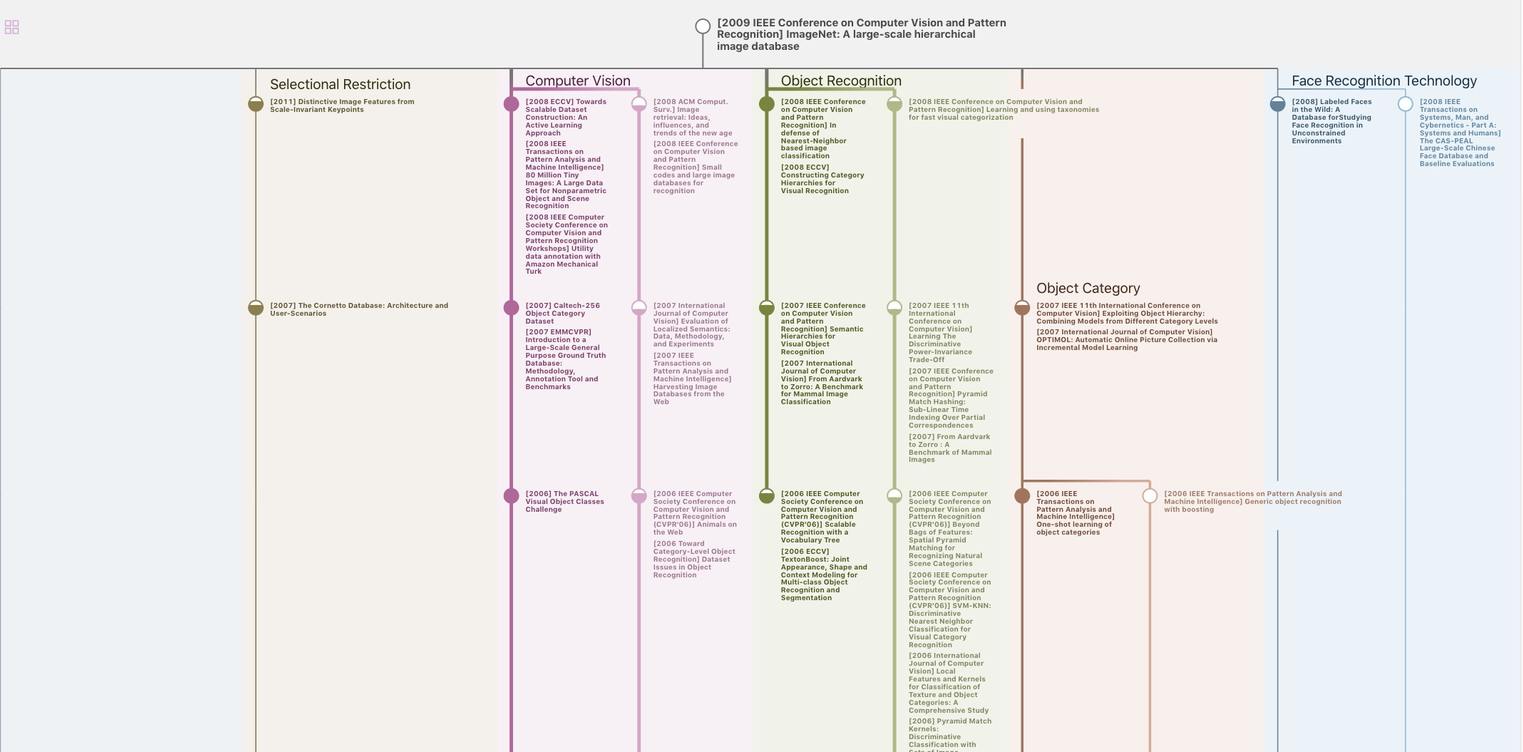
生成溯源树,研究论文发展脉络
Chat Paper
正在生成论文摘要