A Study Of Person Re-Identification Design Characteristics For Aerial Data
PATTERN RECOGNITION AND TRACKING XXXII(2021)
摘要
As the technological advances of the last decade have led to increased performance and availability of video cameras, along with the rise of deep learning-based image recognition, the task of person re-identification has almost exclusively been studied on datasets with ground-based, static camera settings. Yet re-identification applications on aerial-based data captured by Unmanned Aerial Vehicles (UAVs) can be particularly valuable for monitoring public events, border protection, and law enforcement. For a long time no publicly available UAV-based re-identification datasets of sufficient size for modern machine learning techniques existed, which prevented research in this area. Recently, however, two new large-scale UAV-based datasets have been released. We examine re-identification performances of common neural networks on the newly released PRAI-1581 and P-DESTRE aerial-based datasets for UAV-related error sources and data augmentation strategies to increase robustness against them. Our findings of common error sources for these UAV-based datasets include occlusions, camera angles, bad poses, and low resolutions. Furthermore, data augmentation techniques such as rotating images during training prove to be a promising aid for training on the UAV-based data with varying camera angles. By carefully selecting robust networks in addition to choosing adequate training parameters and data augmentation strategies we are able to surpass the original re-identification accuracies published by the authors of the PRAI-1581 and the P-DESTRE dataset respectively.
更多查看译文
关键词
re-identification, UAV, P-DESTRE, PRAI-1581, aerial
AI 理解论文
溯源树
样例
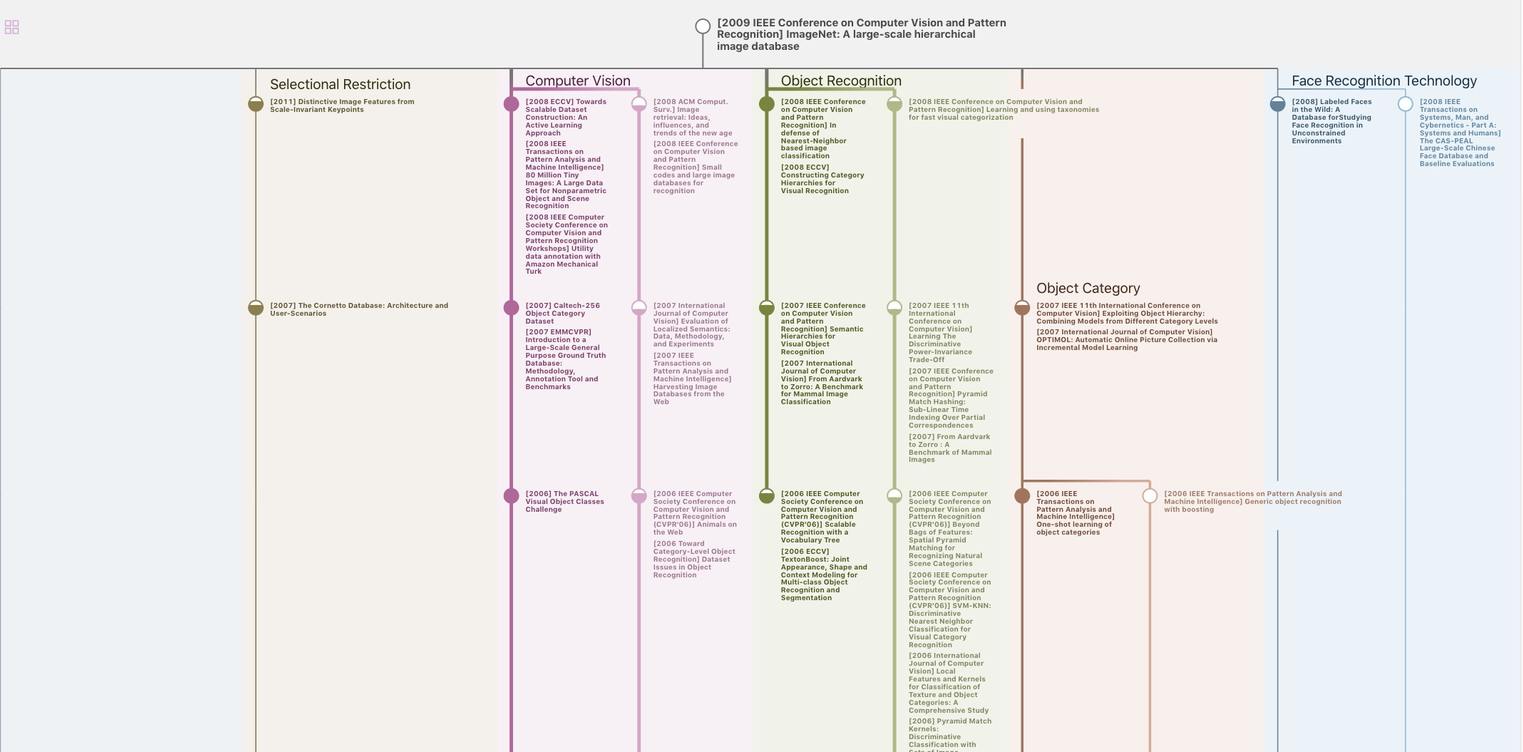
生成溯源树,研究论文发展脉络
Chat Paper
正在生成论文摘要