Dynamically adjusting the k-values of the ATCS rule in a flexible flow shop scenario with reinforcement learning
INTERNATIONAL JOURNAL OF PRODUCTION RESEARCH(2023)
摘要
Given the fact that finding the optimal sequence in a flexible flow shop is usually an NP-hard problem, priority-based sequencing rules are applied in many real-world scenarios. In this contribution, an innovative reinforcement learning approach is used as a hyper-heuristic to dynamically adjust the k-values of the ATCS sequencing rule in a complex manufacturing scenario. For different product mixes as well as different utilisation levels, the reinforcement learning approach is trained and compared to the k-values found with an extensive simulation study. This contribution presents a human comprehensible hyper-heuristic, which is able to adjust the k-values to internal and external stimuli and can reduce the mean tardiness up to 5%.
更多查看译文
关键词
Sequencing rules, dynamic adjustment, simulation study, reinforcement learning, production planning and control
AI 理解论文
溯源树
样例
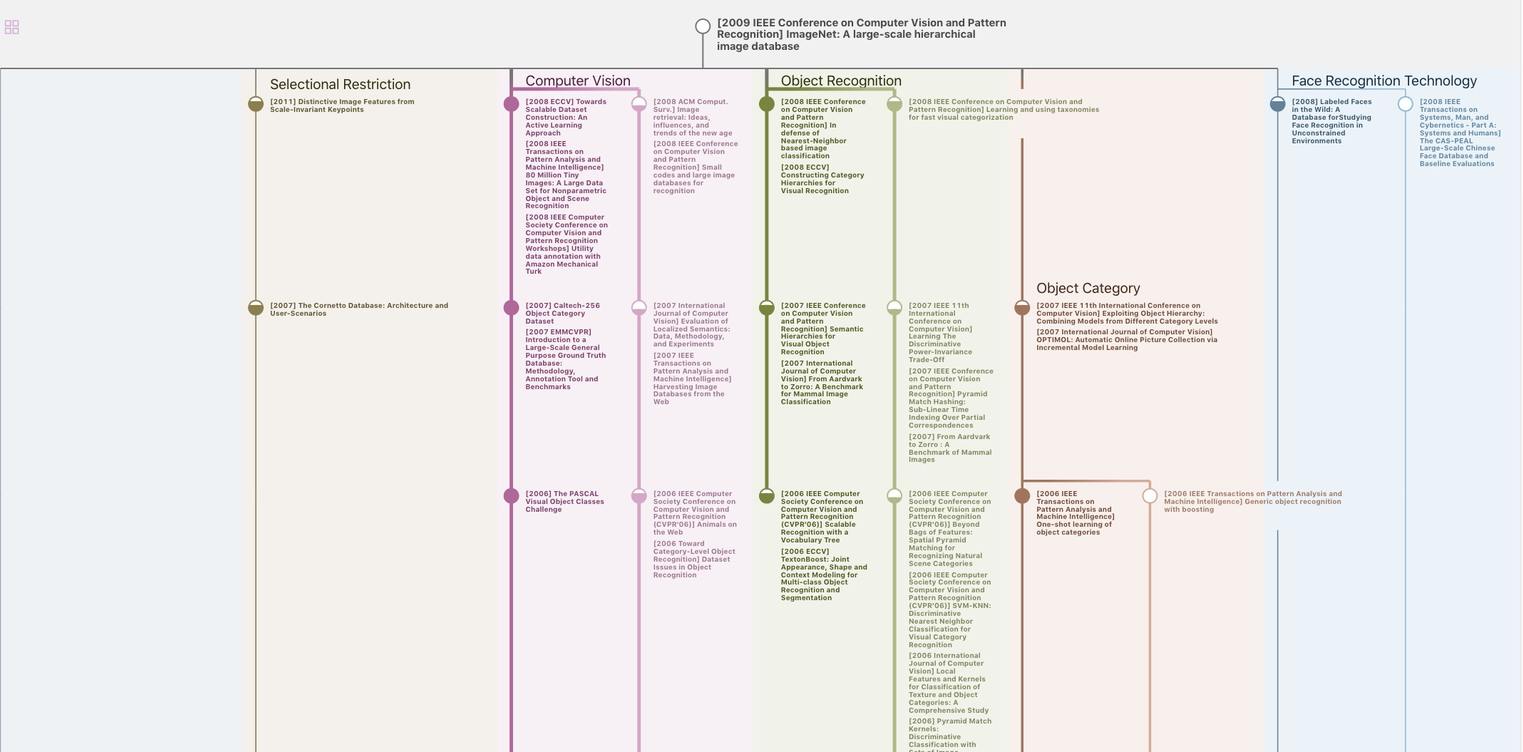
生成溯源树,研究论文发展脉络
Chat Paper
正在生成论文摘要