Demystifying Dark Matter For Online Experimentation
2017 IEEE INTERNATIONAL CONFERENCE ON BIG DATA (BIG DATA)(2017)
摘要
The rise of online controlled experimentation, a.k.a. A/B testing began around the turn of the millennium with the emergence of internet giants like Amazon, Bing, Facebook, Google, LinkedIn, and Yahoo. A step towards good experimental design includes the planning for sample size, confidence level, metrics to be measured and test duration. Generally, these factors impact the quality and validity of an experiment. In practice, additional factors may also impact the validity of an experiment. One such critical factor is the discrepancy between the planned bucket size and the actual bucket size. We call this hidden gap "Experimentation Dark Matter". Experimentation dark matter is invisible to A/A or A/B validation of experimental analysis but can impact the validity of an experiment. In this paper, we have demonstrated in detail, this gap that may cause the loss of statistical power as well as the loss of representativeness and generalizability of an experiment. We have proposed a framework to monitor experimentation dark matter that may go unnoticed in a balanced AB test. We have further discussed the remediation of a recent dark matter issue using our framework. This scalable, low-latency framework is effective and applicable to similar online controlled experimentation systems.
更多查看译文
关键词
online experimentation, quality assurance, bucket size gap, dark matter, loss of traffic, data quality
AI 理解论文
溯源树
样例
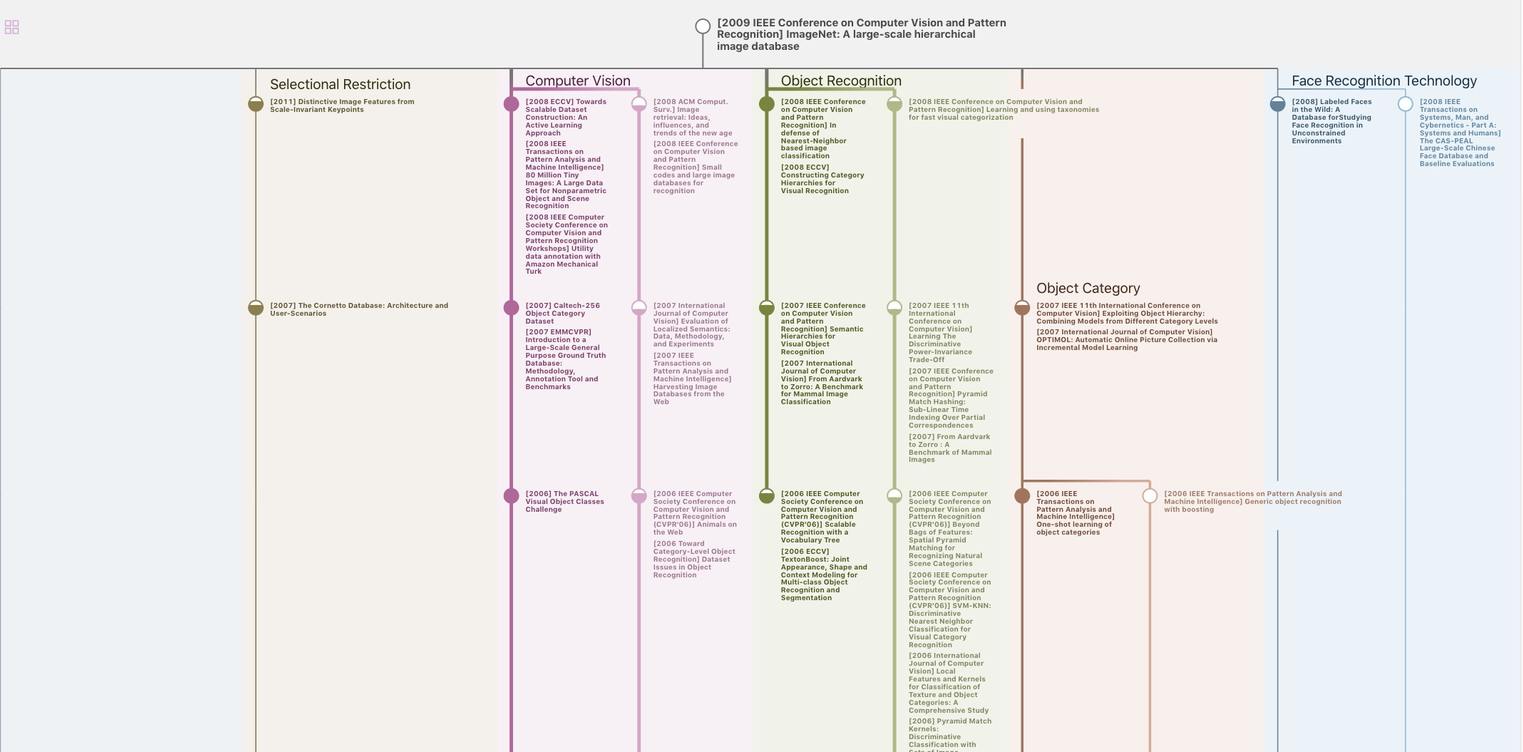
生成溯源树,研究论文发展脉络
Chat Paper
正在生成论文摘要