Performance Optimization In Scale-Out Storage Using Design Of Experiment As Heuristic
2017 IEEE INTERNATIONAL CONFERENCE ON BIG DATA (BIG DATA)(2017)
摘要
As we enter the big data era, the use of data servers continues to increase across the vast range of online services. This will require service and storage providers to improve the capacity and performance of their datacenter infrastructure to meet ever-increasing demands. Adding or replacing more advanced storage hardware can increase the performance, but causes great cost increment to providers. This challenges providers to continue searching for new ways to optimize performance in datacenters, with particular focus on reducing costs and improving efficiency. In this study, tuning configuration parameters for performance optimization is approached systematically and mathematically. Design of Experiment (DOE) as heuristic method uses a series of designed tests from factors with different levels, and mathematically calculates the factors effects on performance. Two different scale-out storage with Ceph-based test environments are prepared for the DOE performance optimization study. One cluster is based on hard disk drives (HDDs) and the other is based on solid state drives (SSDs). A series of tests with IOPS dependent 4 kB to 1 MB block sizes and mixed read-write (0/100-100/0%) are collected through DOE suggested configuration settings on both test environments. These results are compared with the Ceph default configuration setting performance. The comparison results show that DOE suggested configuration setting on both test environments improve up to 35% and 24% of performance in IOPS, respectively. This optimization eventually reduces the overall cost of data cluster without adding or changing hardware. The results demonstrate that DOE is a heuristic method to improve performance efficiently and effectively by simply tuning the configuration.
更多查看译文
关键词
Ceph, storage performance, scale-out, DOE, HDD, SSD, optimization, tuning, Ceph configuration
AI 理解论文
溯源树
样例
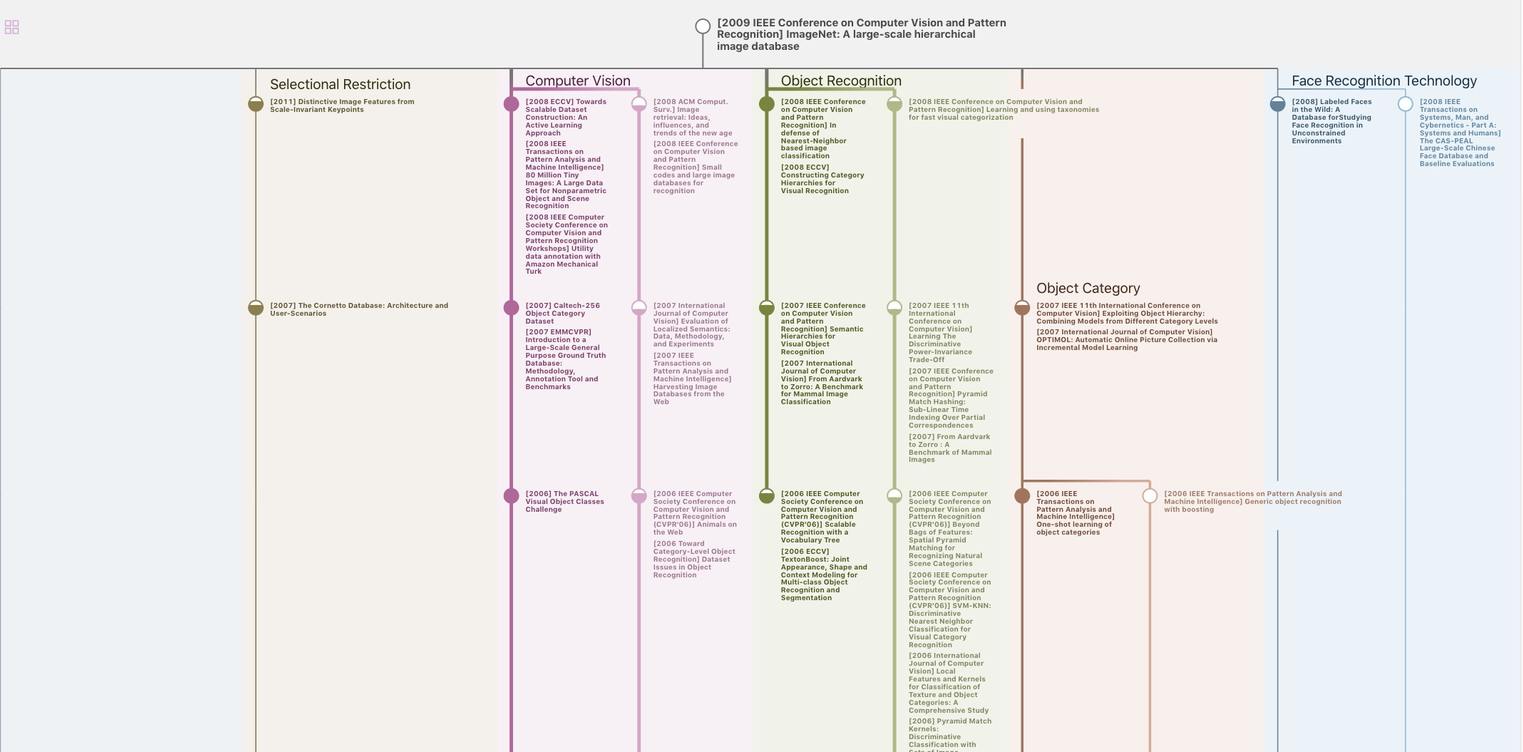
生成溯源树,研究论文发展脉络
Chat Paper
正在生成论文摘要