A Scalable Sequential Principal Component Analysis Algorithm (Seqpca) With Application To User Access Control Analysis
2017 IEEE INTERNATIONAL CONFERENCE ON BIG DATA (BIG DATA)(2017)
摘要
Principal Component Analysis (PCA) is a powerful tool for data exploration and dimensionality reduction, and has broad applications in customer behavior and feedback mining. With the recent breakthroughs in big data technology, PCA becomes even more prevalent in large-scale data mining and business analytics, especially in online customer behavior analysis. However, with the rapidly growing volume of data sets, there also exist challenges when PCA is applied to these areas. For example, computing the principal components and determining the best number of components to extract under limited computational resources are two fundamental yet challenging tasks. In this article, we introduce an algorithm called Sequential PCA (SeqPCA), which is able to conduct PCA sequentially on large data sets. With this technique, data analysts can determine the optimal number of components to extract without recomputing PCA many times. This algorithm is applied to the user access control analysis of the internal websites of a large company, and numerical results show that the algorithm has superior performance and enables real-time analysis of large user behavior data.
更多查看译文
关键词
scalable sequential principal component analysis algorithm,SeqPCA,user access control analysis,data exploration,dimensionality reduction,feedback mining,big data technology,large-scale data mining,business analytics,online customer behavior analysis,data sets,computational resources,Sequential PCA,data analysts,real-time analysis,user behavior data,internal Websites
AI 理解论文
溯源树
样例
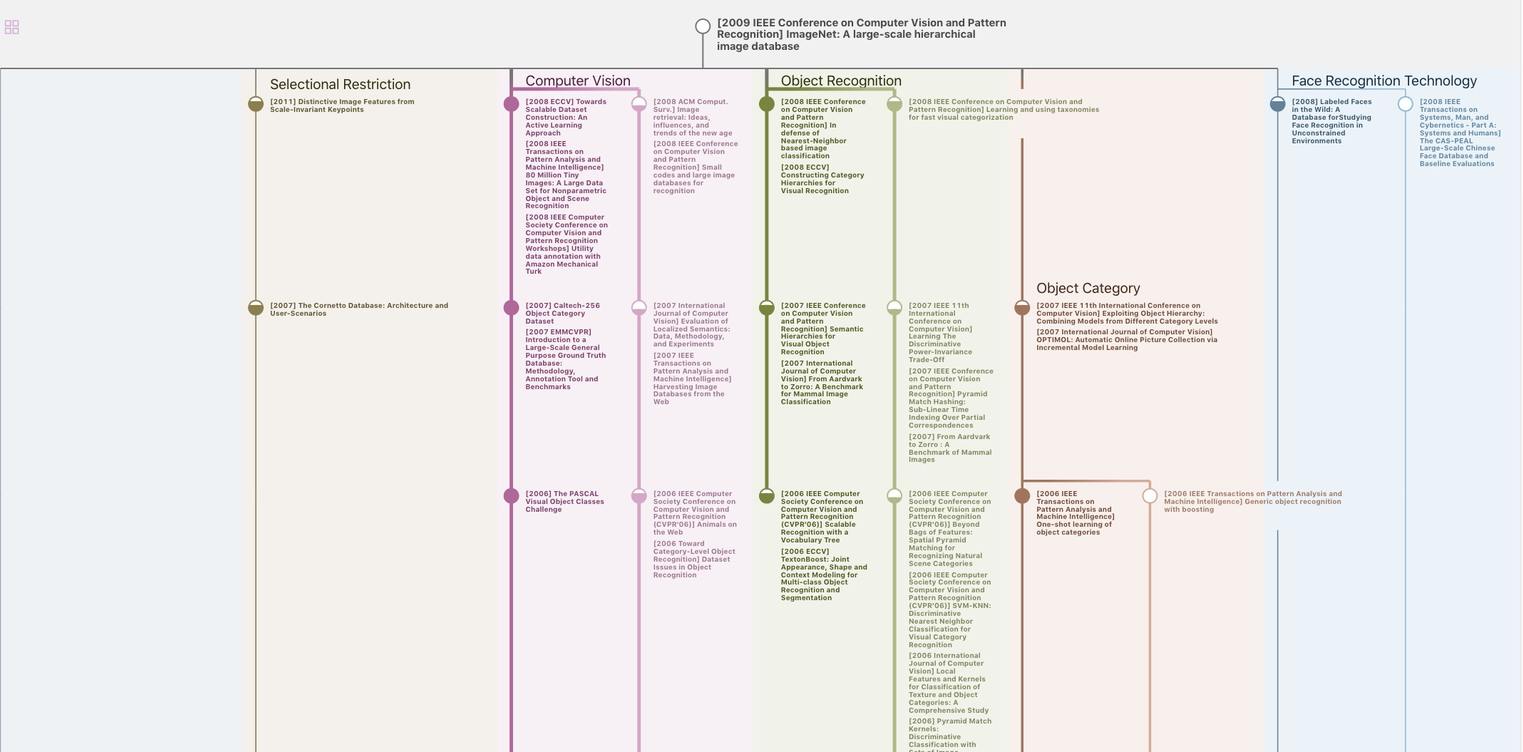
生成溯源树,研究论文发展脉络
Chat Paper
正在生成论文摘要