Deep Learning With Context Encoding For Semantic Brain Tumor Segmentation And Patient Survival Prediction
MEDICAL IMAGING 2020: COMPUTER-AIDED DIAGNOSIS(2020)
摘要
One of the most challenging problems encountered in deep learning-based brain tumor segmentation models is the misclassification of tumor tissue classes due to the inherent imbalance in the class representation. Consequently, strong regularization methods are typically considered when training large-scale deep learning models for brain tumor segmentation to overcome undue bias towards representative tissue types. However, these regularization methods tend to be computationally exhaustive, and may not guarantee the learning of features representing all tumor tissue types that exist in the input MRI examples. Recent work in context encoding with deep CNN models have shown promise for semantic segmentation of natural scenes, with particular improvements in small object segmentation due to improved representative feature learning. Accordingly, we propose a novel, efficient 3DCNN based deep learning framework with context encoding for semantic brain tumor segmentation using multimodal magnetic resonance imaging (mMRI). The context encoding module in the proposed model enforces rich, class-dependent feature learning to improve the overall multi-label segmentation performance. We subsequently utilize context augmented features in a machine-learning based survival prediction pipeline to improve the prediction performance. The proposed method is evaluated using the publicly available 2019 Brain Tumor Segmentation (BraTS) and survival prediction challenge dataset. The results show that the proposed method significantly improves the tumor tissue segmentation performance and the overall survival prediction performance.
更多查看译文
关键词
Brain tumor segmentation,convolutional neural network,context encoding,multimodal magnetic resonance imaging,survival prediction
AI 理解论文
溯源树
样例
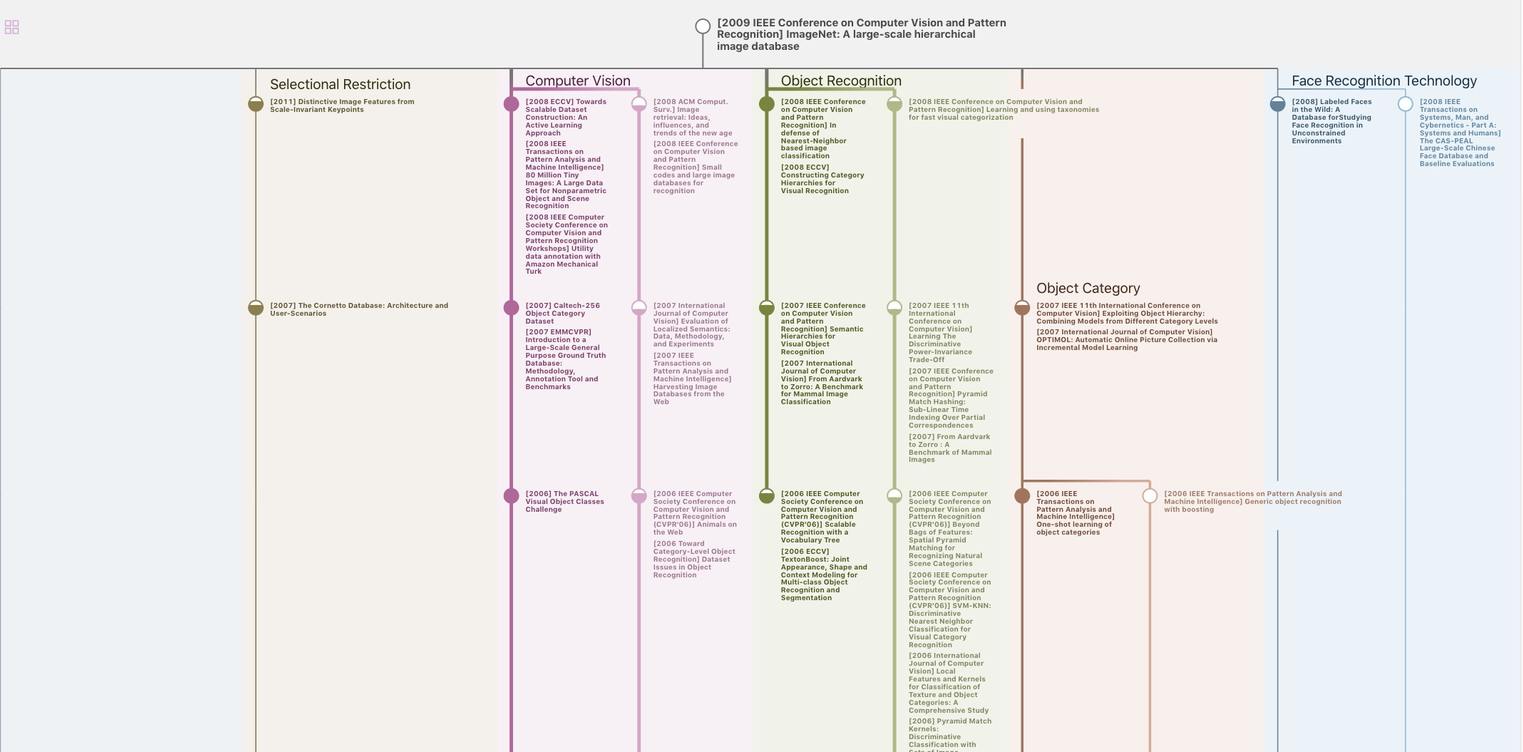
生成溯源树,研究论文发展脉络
Chat Paper
正在生成论文摘要