Cascade Of U-Nets In The Detection And Classification Of Coronary Artery Calcium In Thoracic Low-Dose Ct
MEDICAL IMAGING 2020: COMPUTER-AIDED DIAGNOSIS(2020)
摘要
Low-dose thoracic CT (LDCT) screening has provided a low risk method of obtaining useful clinical information with lower quality images. Coronary artery calcium (CAC), a major indicator of cardiovascular disease, can be visualized on LDCT images. Additionally, the U-Net architecture has shown outstanding performance in a variety of medical imaging tasks, including image segmentation. Thus, the purpose of this study is to analyze the potential of the U-Net in the classification and localization of CAC in LDCT images. This study was performed with 814 LDCT cases with radiologist-determined CAC severity scores. A total of 3 truth masks per image were manually created for training of 3 U-Nets that were used to define the CAC search region, identify CAC candidates, and eliminate false positives (namely, aortic valve calcifications). Additionally, a single network tasked with only CAC candidate identification was tested to assess the need for different sections of the cascade of U-Nets. All CAC segmentation tasks were assessed using ROC analysis in the task of determining whether or not a case contained any CAC. The area under the ROC curve (AUC) as a performance metric and preliminary analysis showed potential for extension to a full classification task. CAC detection through the total cascade of 3 networks achieved and AUC of 0.97 +/- 0.01. Overall, this study shows significant promise in the localization and classification of CAC in LDCT images using a cascade of U-Nets.
更多查看译文
AI 理解论文
溯源树
样例
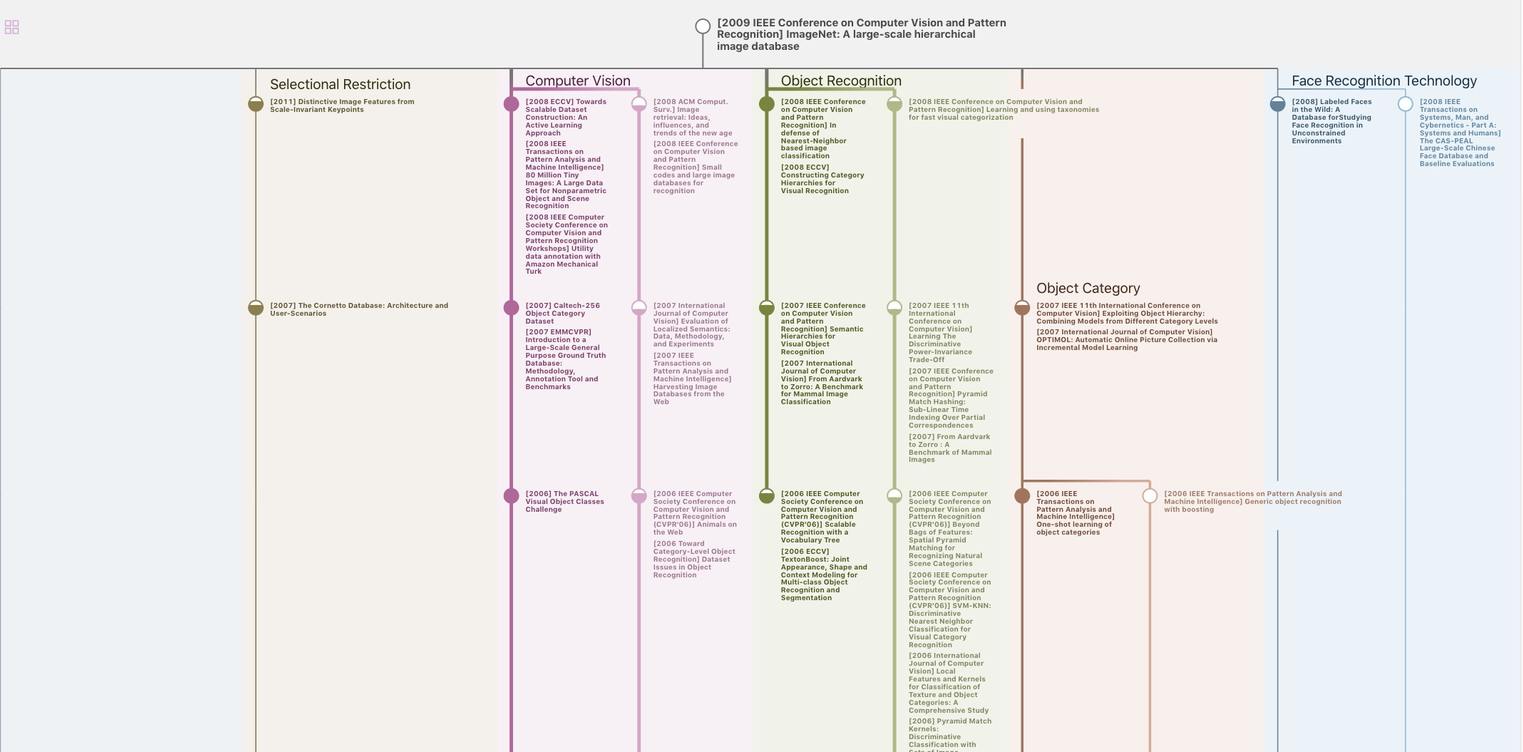
生成溯源树,研究论文发展脉络
Chat Paper
正在生成论文摘要