Tests of Equal Forecasting Accuracy for Nested Models with Estimated CCE Factors*
JOURNAL OF BUSINESS & ECONOMIC STATISTICS(2022)
摘要
In this article, we propose new tests of equal predictive ability between nested models when factor-augmented regressions are used to forecast. In contrast to the previous literature, the unknown factors are not estimated by principal components but by the common correlated effects (CCE) approach, which employs cross-sectional averages of blocks of variables. This makes for easy interpretation of the estimated factors, and the resulting tests are easy to implement and they account for the block structure of the data. Assuming that the number of averages is larger than the true number of factors, we establish the limiting distributions of the new tests as the number of time periods and the number of variables within each block jointly go to infinity. The main finding is that the limiting distributions do not depend on the number of factors but only on the number of averages, which is known. The important practical implication of this finding is that one does not need to estimate the number of factors consistently in order to apply our tests.
更多查看译文
关键词
Common correlated effects, Common factor model, Factor-augmented regression model, Forecasting
AI 理解论文
溯源树
样例
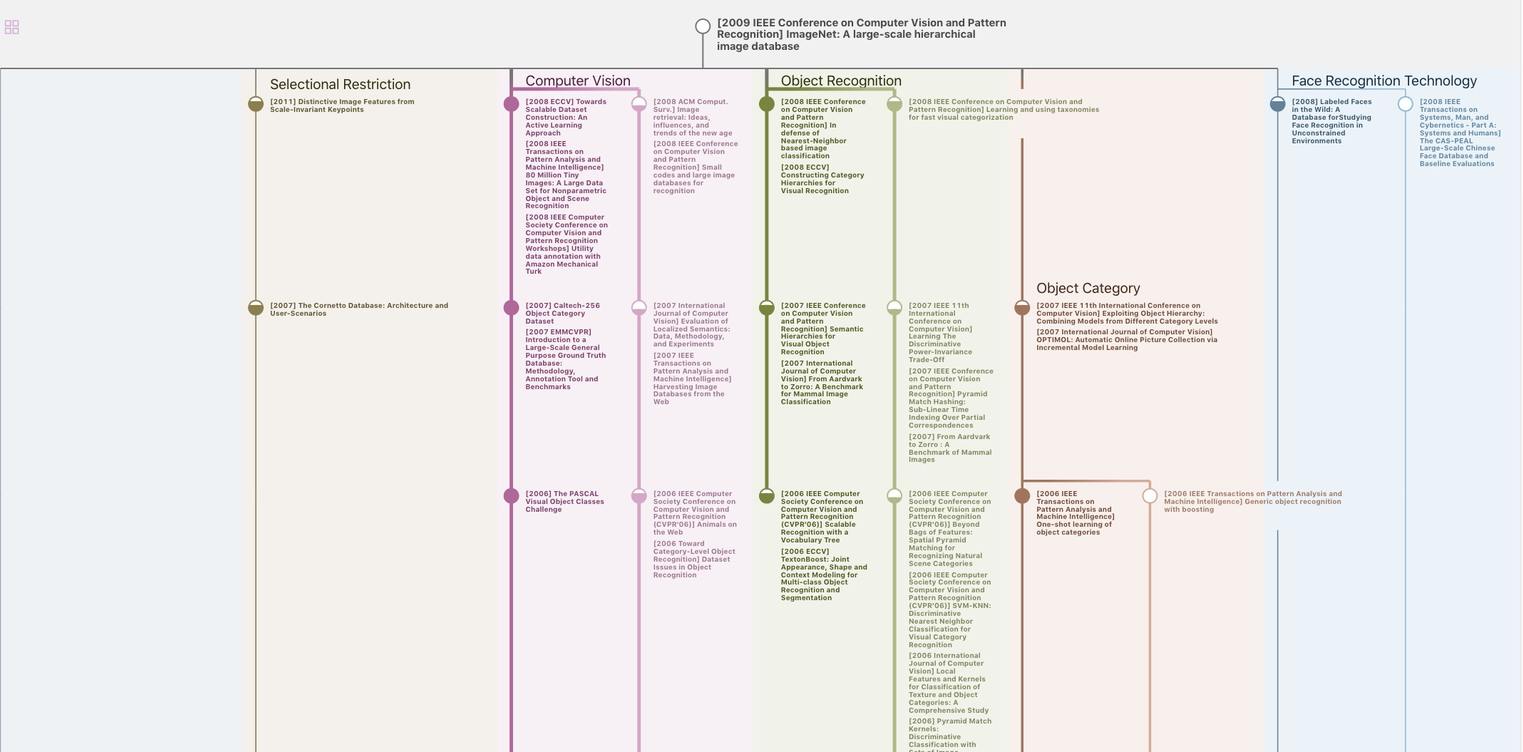
生成溯源树,研究论文发展脉络
Chat Paper
正在生成论文摘要