Image Segmentation in 3D Brachytherapy Using Convolutional LSTM
JOURNAL OF MEDICAL AND BIOLOGICAL ENGINEERING(2021)
摘要
Purpose The accuracy of the segmentation of the target lesion and at-risk surrounding organs is important for cervical cancer patients treated with three-dimensional (3D) brachytherapy. However, the nature of brachytherapy, organ deformities, metal induced artifacts caused by applicators, and limited operating time make this process a challenge. Deep learning segmentation has recently emerged as an approach to this problem. The basic concept proposed in this paper is accurate segmentation of a pelvic computed tomography image dataset such that patients can obtain more accurate radiotherapy. Methods In this work, we propose a solution based on 3D U-Net and Long Short-Term Memory (LSTM). The model was trained and tested on a computed tomography pelvic image dataset comprising 51 patients who underwent 3D brachytherapy. The organs that required segmentation included the bladder, bowels, sigmoid colon, rectum, and uterus. We also used self-ensemble to improve segmentation accuracy. The Dice coefficient was used as the evaluation metric to determine the segmentation results for all of the organs under consideration. Results The proposed model was evaluated using organ segmentation obtained from 10 patients with newly diagnosed stage I-IVA cervical cancer. The test results showed that segmentation conducted using the proposed model resulted in the following Dice coefficient values (mean, ± standard deviation): 87 (± 6.3) %, 72 (± 9.1) %, 72 (± 7.8) %, 95 (± 3.5) %, 86 (± 4.9) %, 77 (± 8.4) %, 73 (± 10.2) %, and 93 (± 3.5) % for the HRCTV, GTV, bowels, foley, bladder, rectum, sigmoid colon, and uterus, respectively. Conclusion This method shows that the combination of 3D U-Net and LSTM and self-ensemble post-processing has high potential for segmentation of a pelvic computed tomography dataset.
更多查看译文
关键词
Cervical cancer, Brachytherapy, Pelvic computed tomography image, 3D U-net, LSTM, Segmentation, Self-ensemble
AI 理解论文
溯源树
样例
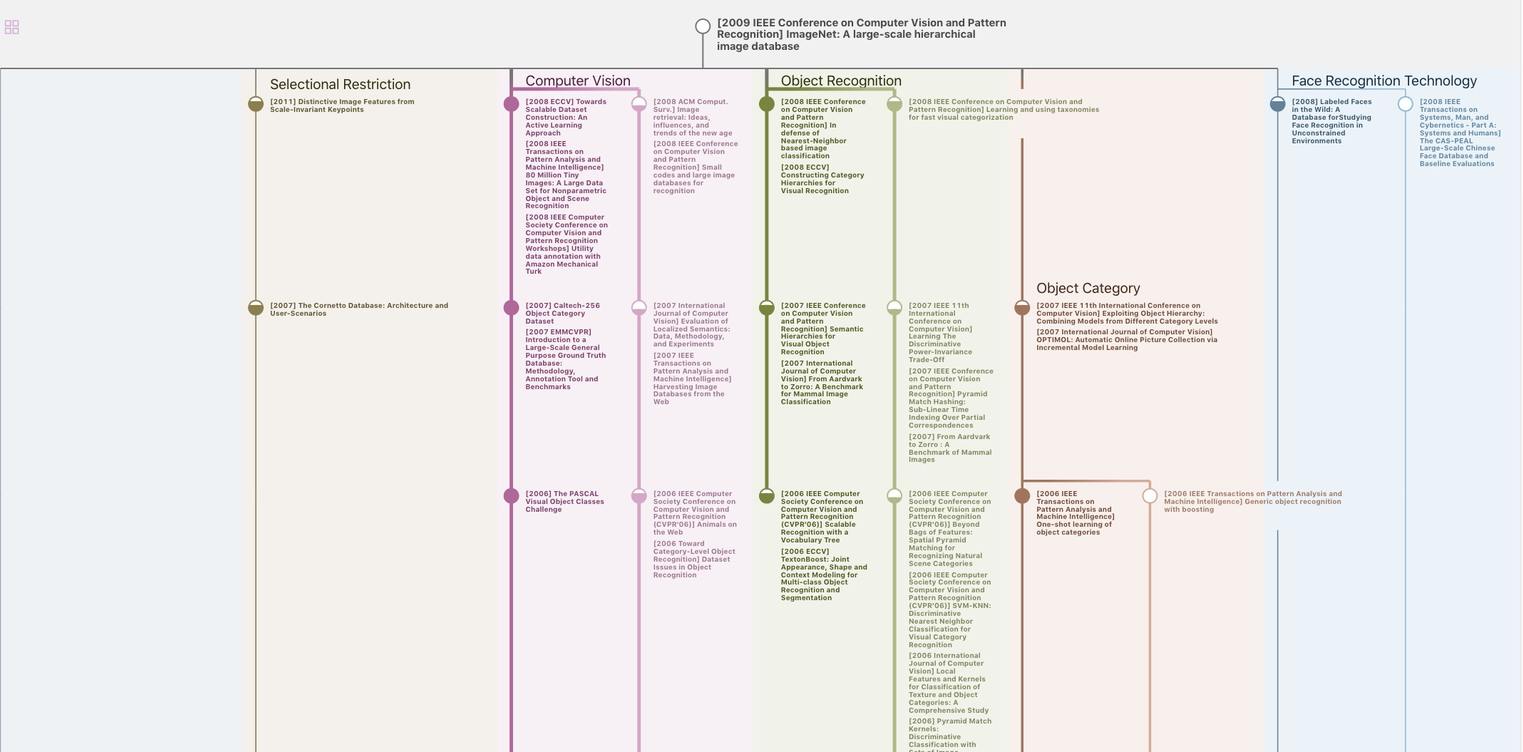
生成溯源树,研究论文发展脉络
Chat Paper
正在生成论文摘要