Coronary Artery Disease Detection By Using Support Vector Machines And Gaussian Mixture Model
2019 MEDICAL TECHNOLOGIES CONGRESS (TIPTEKNO)(2019)
摘要
In this study, a technique which detects the anomalies in ECG by using the state-of-the-art signal processing and machine learning methods is developed to perform the robust detection of coronary artery disease (CAD). For this purpose, by using the wideband recordings on European ST-T database, a novel feature extraction technique which obtains ECG features that are critical for the reliable detection of CAD is developed. By using the extracted features, a supervised learning technique based on support vector machines (SVM) and kernel functions which performs the robust detection of CAD is developed. In cases where ischaemic ECG data is missing, an unsupervised learning technique based on Gaussian mixture model (GMM) which performs the robust detection of CAD by using only basal ECG data is developed. A Neyman-Pearson type of approach is developed to perform the robust detection of the outliers that correspond to CAD. The performance results of the proposed technique over European ST-T database show that the technique provides highly reliable detection of CAD.
更多查看译文
关键词
ECG, feature extraction, support vector machines, kernel functions, unsupervised learning, clustering, Gaussian mixture model, Neyman-Pearson criterion, anomaly detection
AI 理解论文
溯源树
样例
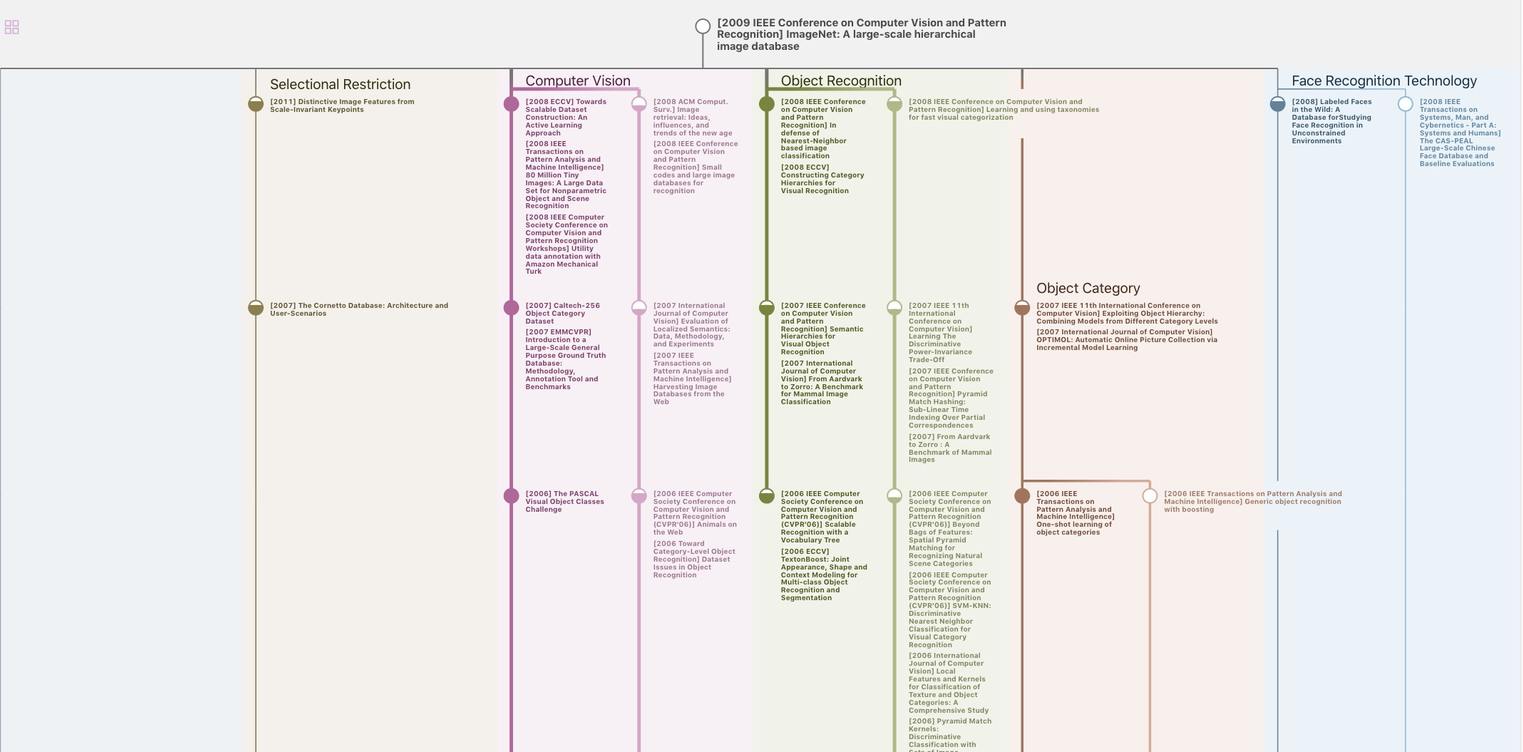
生成溯源树,研究论文发展脉络
Chat Paper
正在生成论文摘要