Cellwise outlier detection with false discovery rate control
CANADIAN JOURNAL OF STATISTICS-REVUE CANADIENNE DE STATISTIQUE(2022)
摘要
This article is concerned with detecting cellwise outliers in large data matrices. We introduce a novel method that is able to fully exploit dependence structures among variables while controlling the false discovery rate (FDR). We reframe cellwise outlier identification into a high-dimensional variable selection paradigm and construct "binate references" for data screening, estimation and information pooling. With the binate references, the proposed procedure forms a series of statistics that incorporate covariance information and utilizes a global symmetry property of these statistics to approximate the false discovery proportion. We show that the proposed method can control the asymptotic FDR under some mild conditions. Extensive numerical studies demonstrate that our method has reasonable FDR control and satisfactory power in comparison to existing methods.
更多查看译文
关键词
Dependence structure, empirical distribution, FDR control, feature screening, high-dimensional data, uniform convergence
AI 理解论文
溯源树
样例
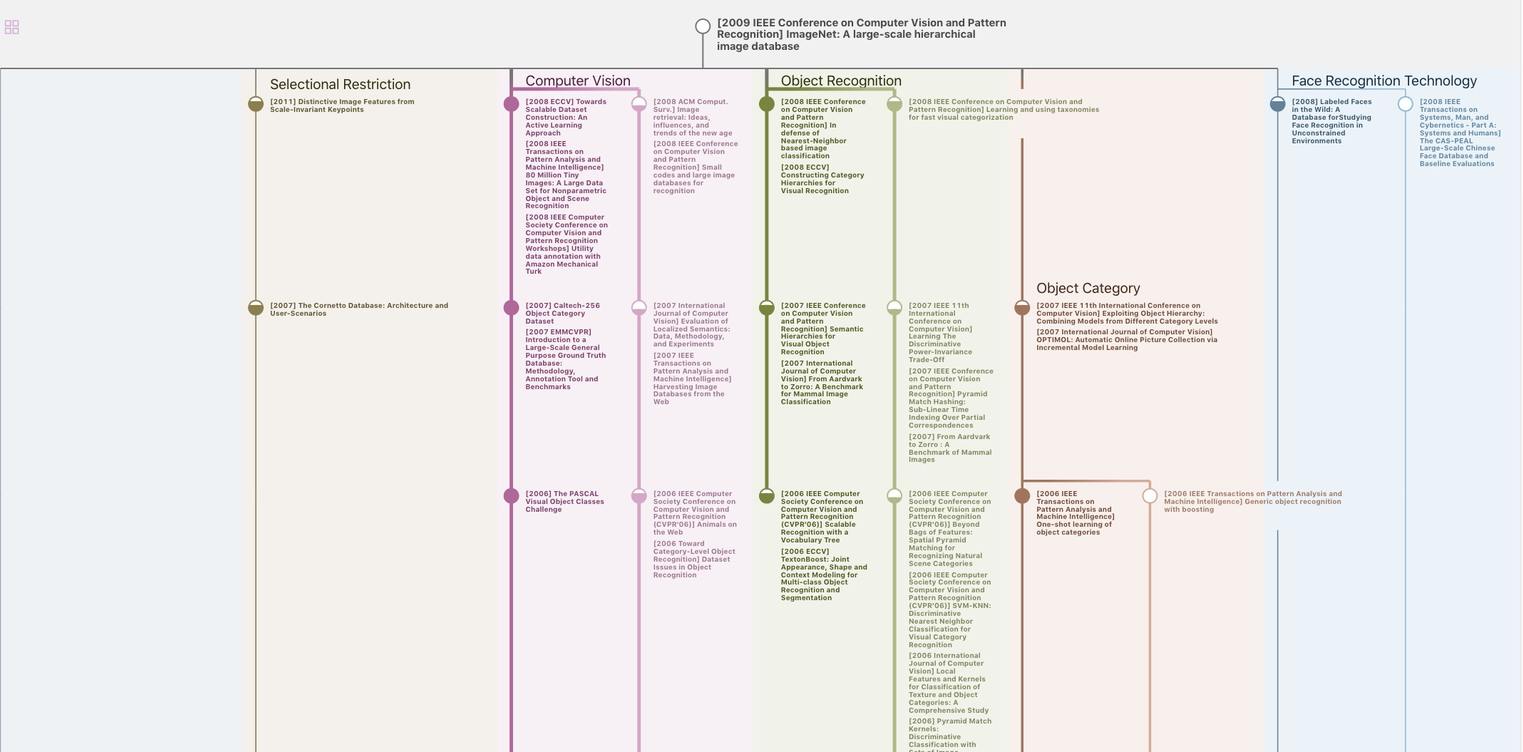
生成溯源树,研究论文发展脉络
Chat Paper
正在生成论文摘要