Traffic Volume Prediction: A Fusion Deep Learning Model Considering Spatial-Temporal Correlation
SUSTAINABILITY(2021)
摘要
In this paper, a fusion deep learning model considering spatial-temporal correlation is proposed to solve the problem of urban road traffic flow prediction. Firstly, this paper holds that the traffic flow of a section in the urban road network not only depends on the fluctuation of its own time series, but is also related to the traffic flow of other sections in the whole region. Therefore, a traffic flow similarity measurement method based on wavelet decomposition and dynamic time warping is proposed to screen the sections which are similar to the traffic flow state of the target section. Secondly, in order to improve the prediction accuracy, the unstable time series are reconstructed into stationary time series by differential method. Finally, taking the extracted traffic flow data of a similar section as an independent variable and the traffic flow data of target section as dependent variable, we input the above variables into the proposed CNN-LSTM fusion deep learning model for traffic flow prediction. The results show that the proposed model has a higher accuracy and stability than the other benchmark models. The MAPE can reach 92.68%, 93.39%, 85.14%, and 76.14% at a time interval of 5 min, 15 min, 30 min, and 60 min, and the other evaluation indexes are also better than the rest of the benchmark models.
更多查看译文
关键词
traffic flow prediction, urban traffic flow, traffic engineering, deep learning
AI 理解论文
溯源树
样例
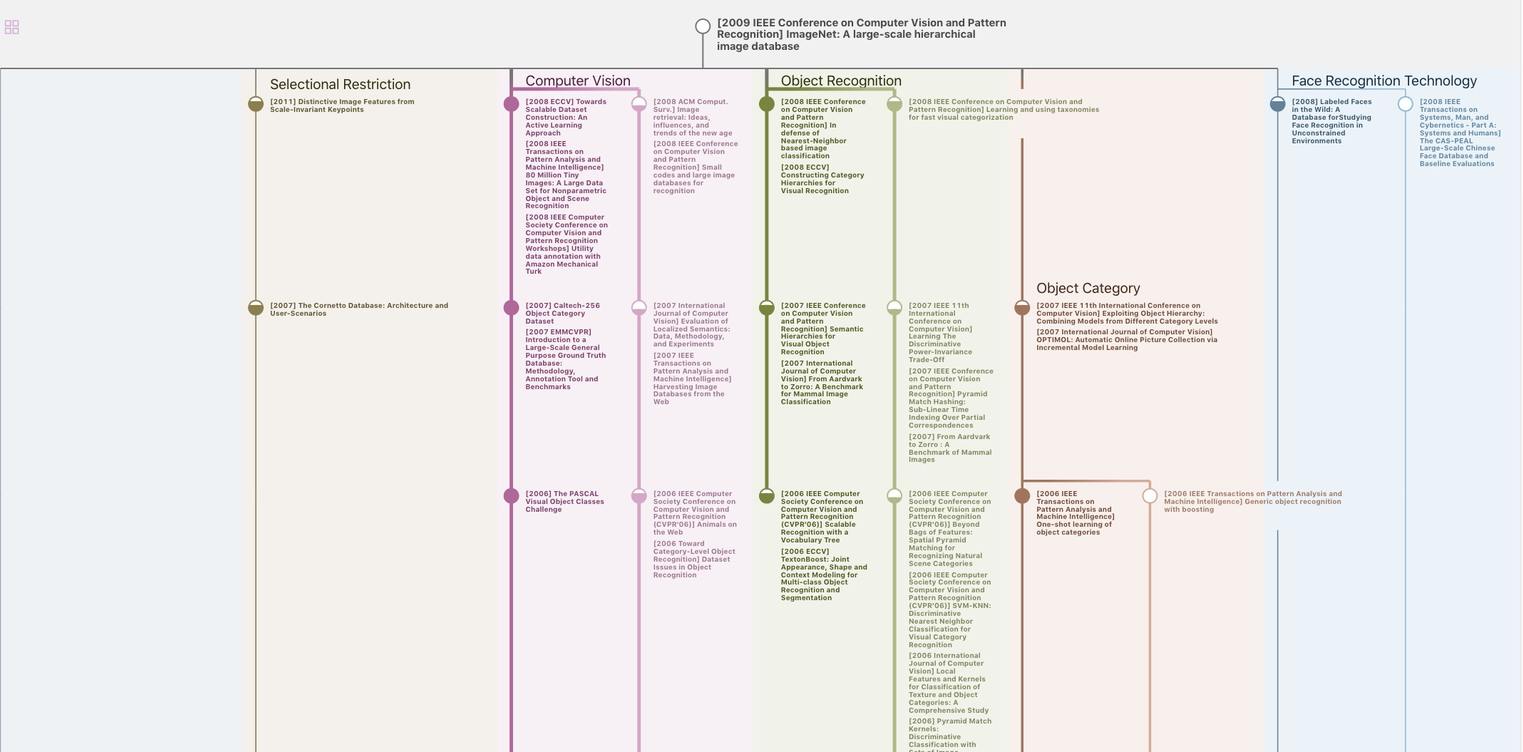
生成溯源树,研究论文发展脉络
Chat Paper
正在生成论文摘要