Graph-Based Hand-Object Meshes And Poses Reconstruction With Multi-Modal Input
IEEE ACCESS(2021)
摘要
Estimating the hand-object meshes and poses is a challenging computer vision problem with many practical applications. In this paper, we introduce a simple yet efficient hand-object reconstruction algorithm. To this end, we exploit the fact that both the poses and the meshes are graphs-based representations of the hand-object with different levels of details. This allows taking advantage of the powerful Graph Convolution networks (GCNs) to build a coarse-to-fine Graph-based hand-object reconstruction algorithm. Thus, we start by estimating a coarse graph that represents the 2D hand-object poses. Then, more details (e.g. third dimension and mesh vertices) are gradually added to the graph until it represents the dense 3D hand-object meshes. This paper also explores the problem of representing the RGBD input in different modalities (e.g. voxelized RGBD). Hence, we adopted a multi-modal representation of the input by combining 3D representation (i.e. voxelized RGBD) and 2D representation (i.e. RGB only). We include intensive experimental evaluations that measure the ability of our simple algorithm to achieve state-of-the-art accuracy on the most challenging datasets (i.e. HO-3D and FPHAB).
更多查看译文
关键词
Three-dimensional displays, Shape, Image reconstruction, Convolution, Pose estimation, Feature extraction, Solid modeling, Hand pose estimation, hand shape estimation, hand-object interaction, graph convolution, machine learning
AI 理解论文
溯源树
样例
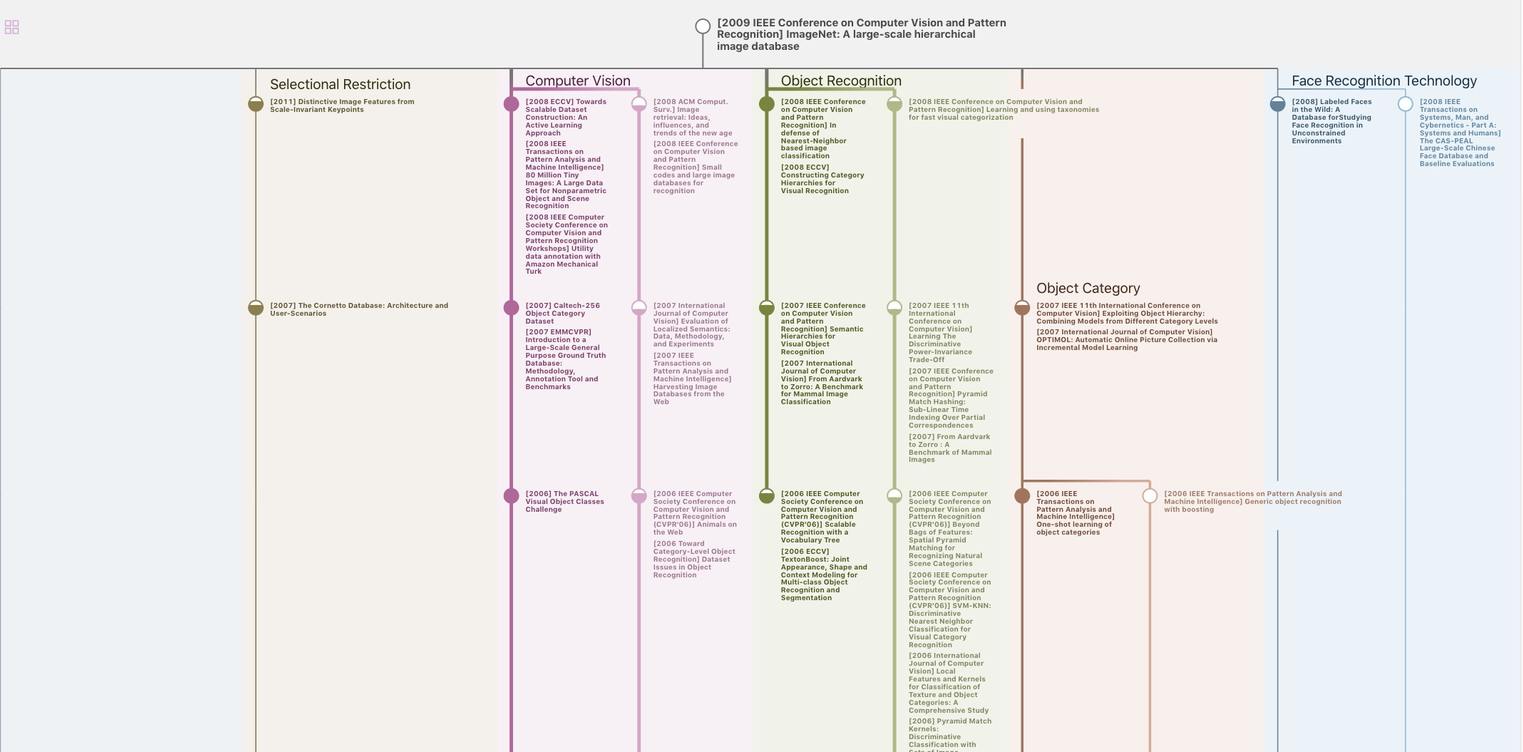
生成溯源树,研究论文发展脉络
Chat Paper
正在生成论文摘要