A Similarity Score Model For Aspect Category Detection
INTERNATIONAL JOURNAL OF ADVANCED COMPUTER SCIENCE AND APPLICATIONS(2021)
摘要
Aspect-based Sentiment Analysis (ABSA) aims to extract significant aspects of an item or product from reviews and predict the sentiment of each aspect. Previous similarity methods tend to extract aspect categories at the word level by combining Language Models (LM) in their models. A drawback for the LM model is its dependence on a large amount of labelled data for a specific domain to function well. This work proposes a mechanism to address labelled data dependency by a one-step approach experimenting to decide the best combinatory architectures of recurrent-based LM and the best semantic similarity measures for fostering a new aspect category detection model. The proposed model addresses drawbacks of previous aspect category detection models in an implicit manner. The datasets of this study, S1 and S2, are from standard SemEval online competition. The proposed model outperforms the previous baseline models in terms of the F1-score of aspect category detection. This study finds more relevant aspect categories by creating a more stable and robust model. The F1 score of our best model for aspect category detection is 79.03% in the restaurant domain for the S1 dataset. In dataset S2, the F1-score is 72.65% in the laptop domain and 75.11% in the restaurant domain.
更多查看译文
关键词
Aspect category detection, language model, semantic similarity
AI 理解论文
溯源树
样例
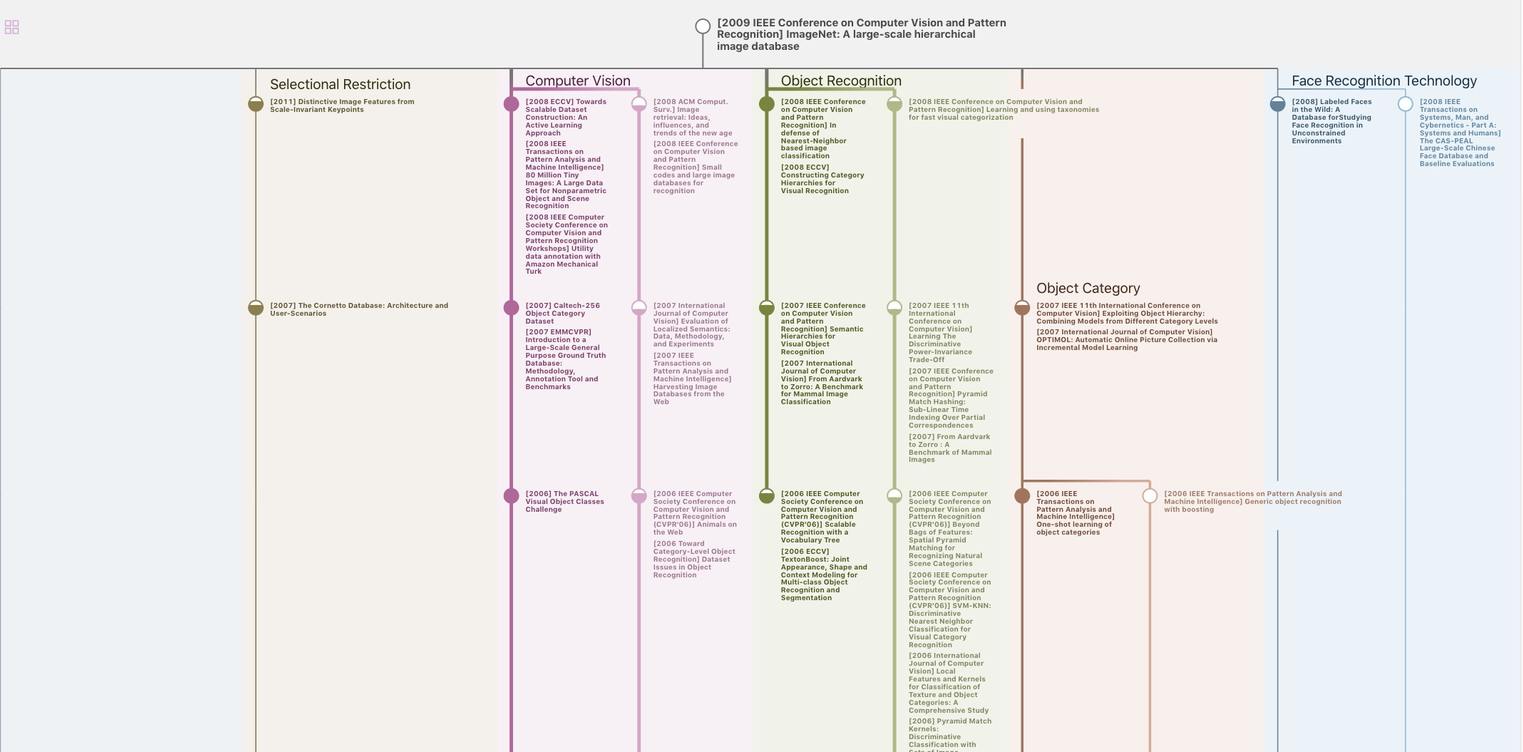
生成溯源树,研究论文发展脉络
Chat Paper
正在生成论文摘要