Experimental Evaluation Of Pso Based Transfer Learning Method For Meteorological Visibility Estimation
ATMOSPHERE(2021)
摘要
Estimation of Meteorological visibility from image characteristics is a challenging problem in the research of meteorological parameters estimation. Meteorological visibility can be used to indicate the weather transparency and this indicator is important for transport safety. This paper summarizes the outcomes of the experimental evaluation of a Particle Swarm Optimization (PSO) based transfer learning method for meteorological visibility estimation method. This paper proposes a modified approach of the transfer learning method for visibility estimation by using PSO feature selection. Image data are collected at fixed location with fixed viewing angle. The database images were gone through a pre-processing step of gray-averaging so as to provide information of static landmark objects for automatic extraction of effective regions from images. Effective regions are then extracted from image database and the image features are then extracted from the Neural Network. Subset of Image features are selected based on the Particle Swarming Optimization (PSO) methods to obtain the image feature vectors for each effective sub-region. The image feature vectors are then used to estimate the visibilities of the images by using the Multiple Support Vector Regression (SVR) models. Experimental results show that the proposed method can give an accuracy more than 90% for visibility estimation and the proposed method is effective and robust.
更多查看译文
关键词
visibility evaluation, feature extraction, particle swarm optimization, transfer learning
AI 理解论文
溯源树
样例
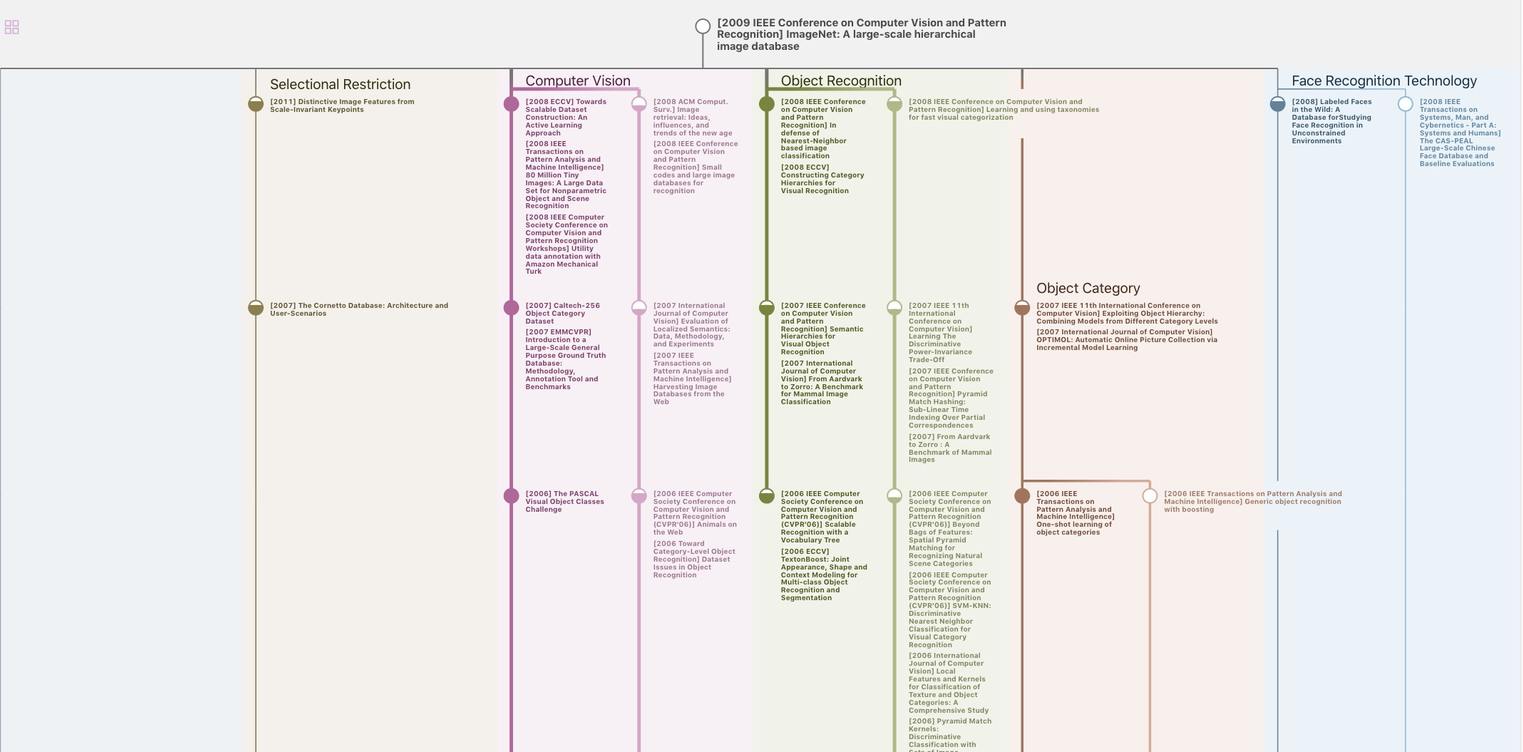
生成溯源树,研究论文发展脉络
Chat Paper
正在生成论文摘要