Probabilistic Machine Learning Assisted Feature-Based Qualification of DED Ti64
JOM(2021)
摘要
Additive manufacturing usually involves the complex interaction of design, materials, and manufacturing, often resulting in long and cost-intensive iterative evaluation cycles. Therefore, it is critical for it to be aligned with the Materials Genome Initiative to develop, produce, and deploy high-throughput components. Recognizing a need, this study leverages a feature-based qualification (FBQ) methodology to decompose a complex structure by identifying critical performance-limiting features, for the purpose of reducing the cost and time of DED process qualification. A hybrid-physics-based multi-objective optimization tool was used to predict processing-structure-property relationships in thin-walled builds. The probabilistic ML models achieved targeted predictions with half the sample space when compared with conventional DOEs, while also being 37–50% more reliable with respect to regression tools with linear basis function. Although the current model developed is specific to Ti64 builds in a RPMi557 powder-feed DED machine, the FBQ methodology may be more universally employed to other material-modality combinations.
更多查看译文
AI 理解论文
溯源树
样例
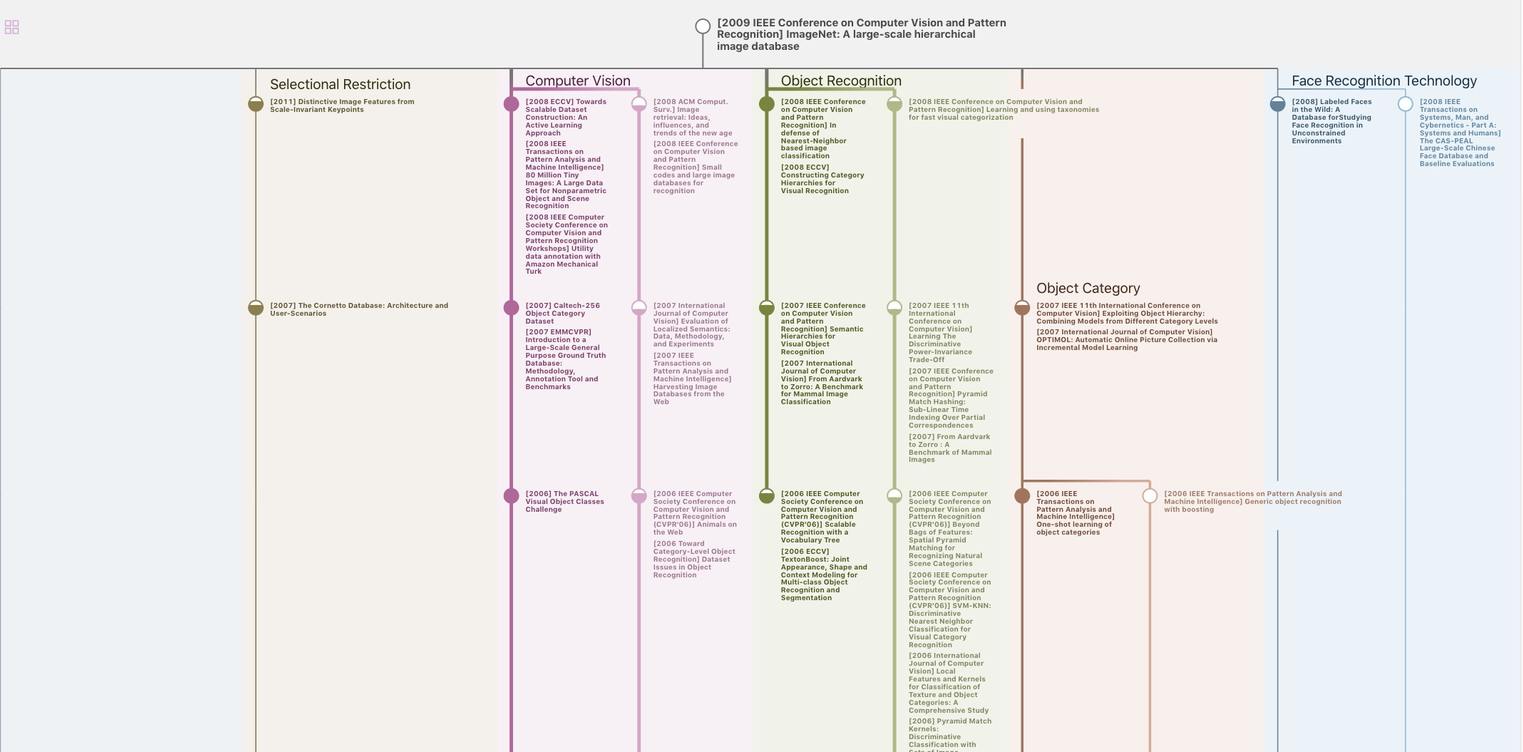
生成溯源树,研究论文发展脉络
Chat Paper
正在生成论文摘要