High-Dimensional Neural Network Atomic Potentials For Examining Energy Materials: Some Recent Simulations
JOURNAL OF PHYSICS-ENERGY(2021)
摘要
Owing to their simultaneous accuracy and computational efficiency, interatomic potentials machine-learned using first-principles calculation data are promising for investigating phenomena closely related to atomic motion in various energy materials. We have been working with one type of these potentials, high-dimensional (HD) neural network potentials (NNPs), and their applications, but we realized that our current understanding of HD NNPs, e.g. the meaning of the atomic energy mapping, remained insufficient, and that tuning their prediction performance for different target properties/phenomena often requires much trial and error. In this article, we illustrate the usefulness of NNPs through our studies on ion migration and thermal transport in energy and related materials. We also share our experiences with data sampling and training strategies and discuss the meaning of atomic energy mapping in HD NNPs.
更多查看译文
关键词
neural network, machine learning, ion migration, thermal transport
AI 理解论文
溯源树
样例
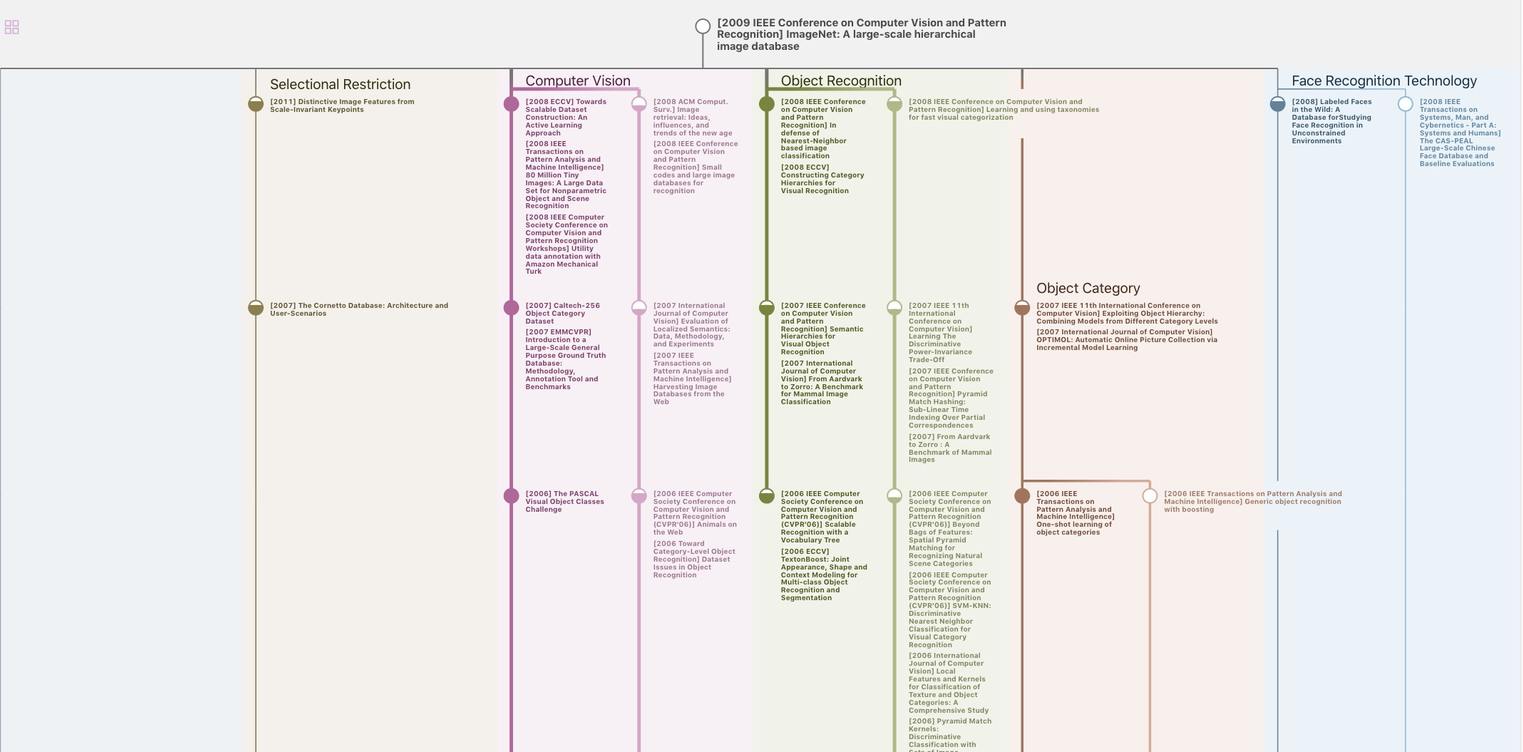
生成溯源树,研究论文发展脉络
Chat Paper
正在生成论文摘要