Bayesian Variable Selection With Applications In Health Sciences
MATHEMATICS(2021)
摘要
In health sciences, identifying the leading causes that govern the behaviour of a response variable is a question of crucial interest. Formally, this can be formulated as a variable selection problem. In this paper, we introduce the basic concepts of the Bayesian approach for variable selection based on model choice, emphasizing the model space prior adoption and the algorithms for sampling from the model space and for posterior probabilities approximation; and show its application to two common problems in health sciences. The first concerns a problem in the field of genetics while the second is a longitudinal study in cardiology. In the context of these applications, considerations about control for multiplicity via the prior distribution over the model space, linear models in which the number of covariates exceed the sample size, variable selection with censored data, and computational aspects are discussed. The applications presented here also have an intrinsic statistical interest as the proposed models go beyond the standard general linear model. We believe this work will broaden the access of practitioners to Bayesian methods for variable selection.
更多查看译文
关键词
Bayes factor, bayesian model averaging, censored data, conventional prior, multiplicity correction, singular models
AI 理解论文
溯源树
样例
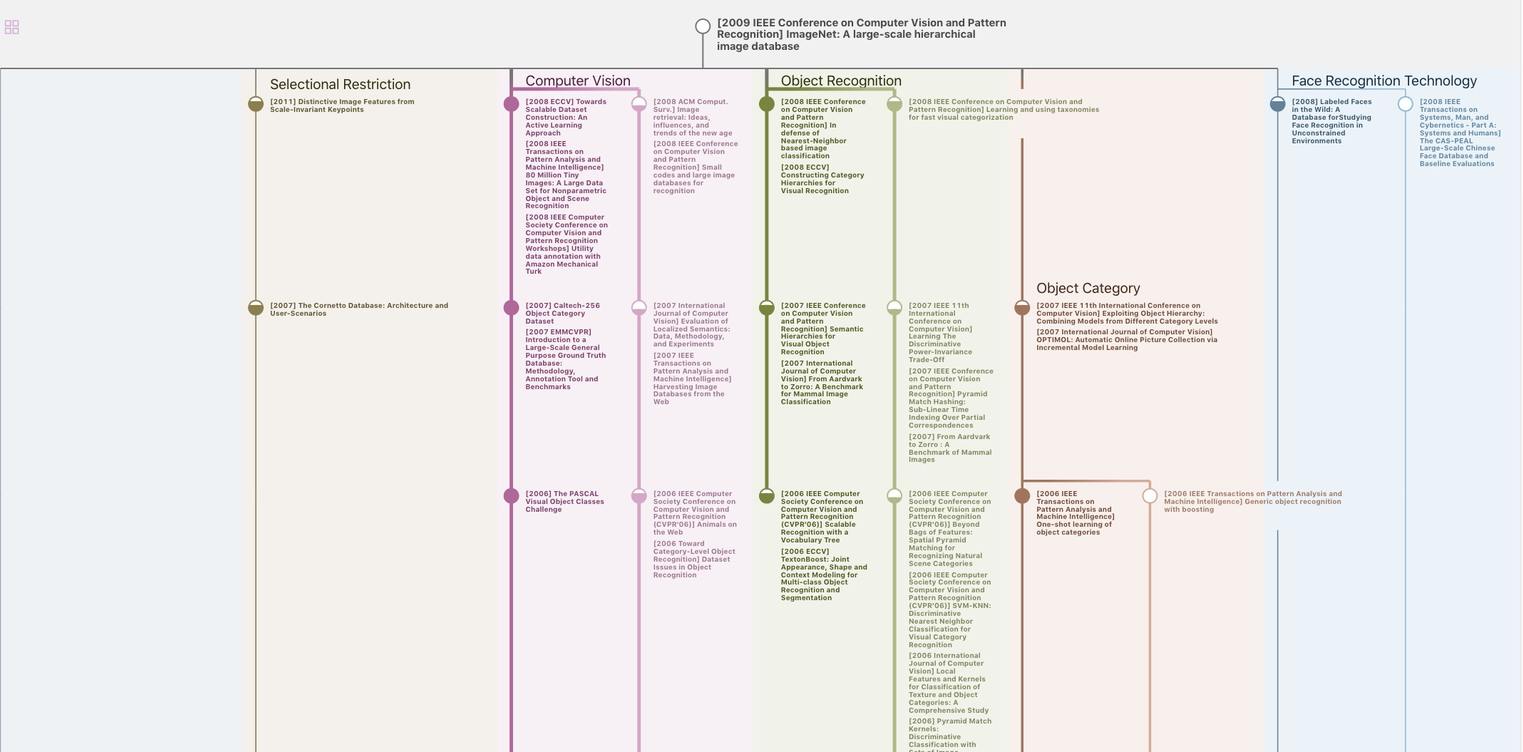
生成溯源树,研究论文发展脉络
Chat Paper
正在生成论文摘要