Approximate explicit feature map for computational augmentation of RGB images of hematoxylin and eosin stained histopathological specimens
MEDICAL IMAGING 2021 - DIGITAL PATHOLOGY(2021)
摘要
Hyperspectral imaging (HSI) is demonstrating the growing capability for disease diagnosis and surgical cancer resection. That is mainly due to high spectral resolution of HSI when compared with its color (RGB) counterparts. However, increased spectral resolution is often associated with the loss of spatial resolution. That combined with high cost hinders applicability of HSI. Herein, we propose computational approach that attempts to mimic the HSI. It is using an approximate explicit feature map (aEFM) to augment raw and/or stain normalized RGB images of the hematoxylin and eosin stained histopathological specimen. We demonstrate on two public labeled datasets, related to breast cancer and nuclei, the statistically significant improvement of performance of binary (caner vs. non-cancer) segmentation of augmented RGB images in comparison with the results achieved on their RGB counterparts. For the breast cancer, balanced accuracy is increased from 76.56%+/- 9.05% to 80.42%+/- 9.23% and Fi score from 13.34%+/- 6.46% to 17.33%+/- 6.36%. For nuclei, balanced accuracy is increased from 68.68%+/- 9.25% to 79.99%+/- 8.77% and F-1 score from 46.92%+/- 15.10% to 63.31%+/- 14.50%. While l(0) constrained nonnegative matrix factorization was used for binary segmentation (h)erein, we conjecture that aEFM based augmentation of RGB images can improve performance of more sophisticated segmentation methods such as deep networks.
更多查看译文
关键词
hyperspectral microscopic image,RGB microscopic image,explicit feature map,computational augmentation,segmentation,histopathology
AI 理解论文
溯源树
样例
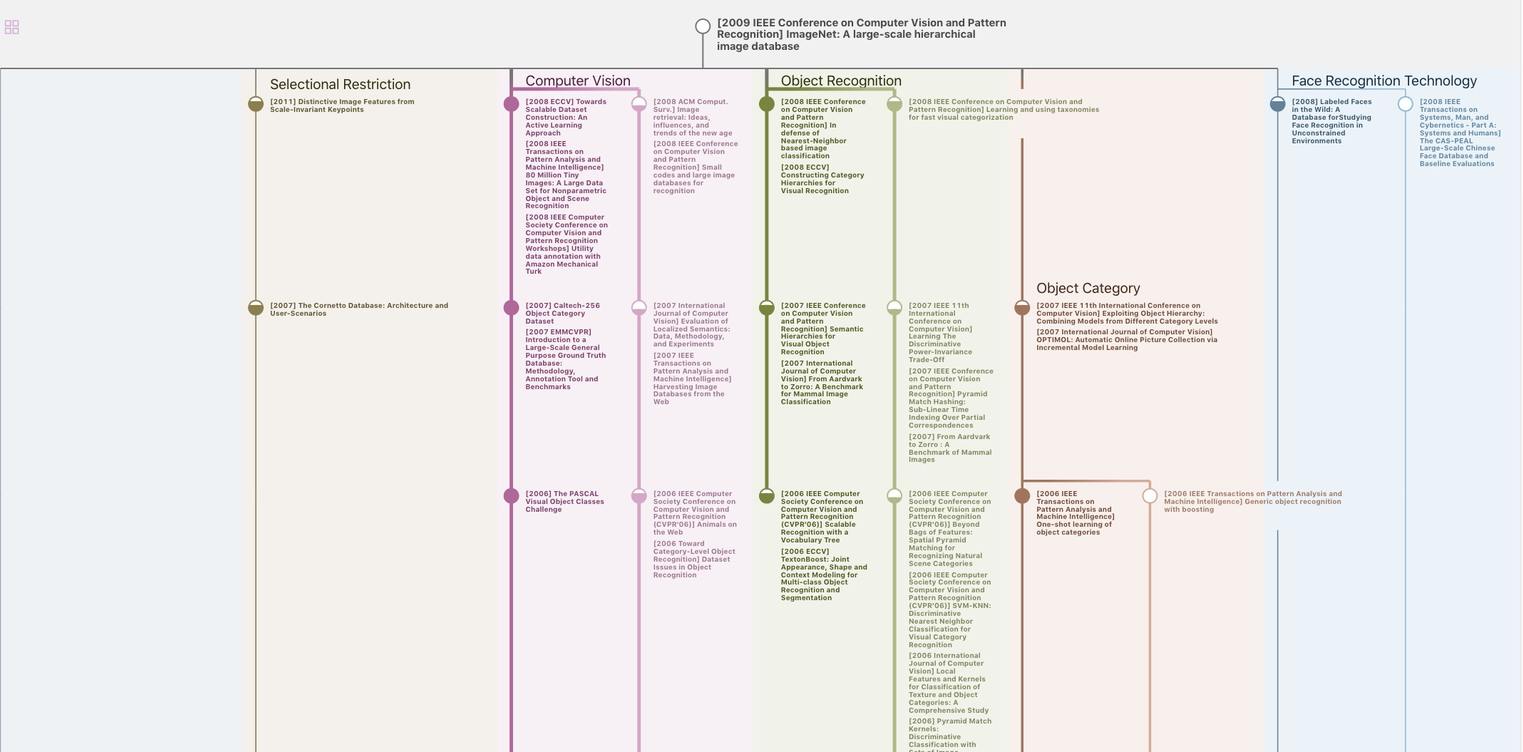
生成溯源树,研究论文发展脉络
Chat Paper
正在生成论文摘要