Evaluation Of An Automated Bone Age Assessment Shows Better Performance In Older Patients
JOURNAL OF MEDICAL IMAGING AND HEALTH INFORMATICS(2021)
摘要
Objective. To assess performance of the automated deep learning algorithm for bone age evaluation on Polish population. Current manual method is time-consuming and was shown to be prone to high intra- and inter-rater variability: therefore, automated methods are being developed and tested. Materials and Methods. The dataset of 2374 hand radiographs from patients aged 0-19 were analyzed by radiologists and later by one of the available automated methods for bone age assessment. Smaller subset of 100 cases was selected and radiographs were assessed by four physicians blinded to the chronological age and compared with algorithms predictions and scores derived from radiological report. Results. For whole dataset Lin's concordance coefficient was 0.96. mean absolute difference between algorithms predictions and physicians assessment was 0.91 years. Analysis of different age groups in the dataset revealed the lowest correlation before 4.5 years of age (Lin's concordance coefficient 0.69). Conclusions. The algorithm showed good correlation with ground truth on the whole dataset, however performance on radiographs of patients under 4.5 years of age needs further improvements. To facilitate the development of the automated bone age estimation subset of 100 cases with clinicians scoring of bone maturity is published as supplementary material to this research.
更多查看译文
关键词
Machine Learning, Deep Learning, Bone Age Assessment, Paediatric Radiology
AI 理解论文
溯源树
样例
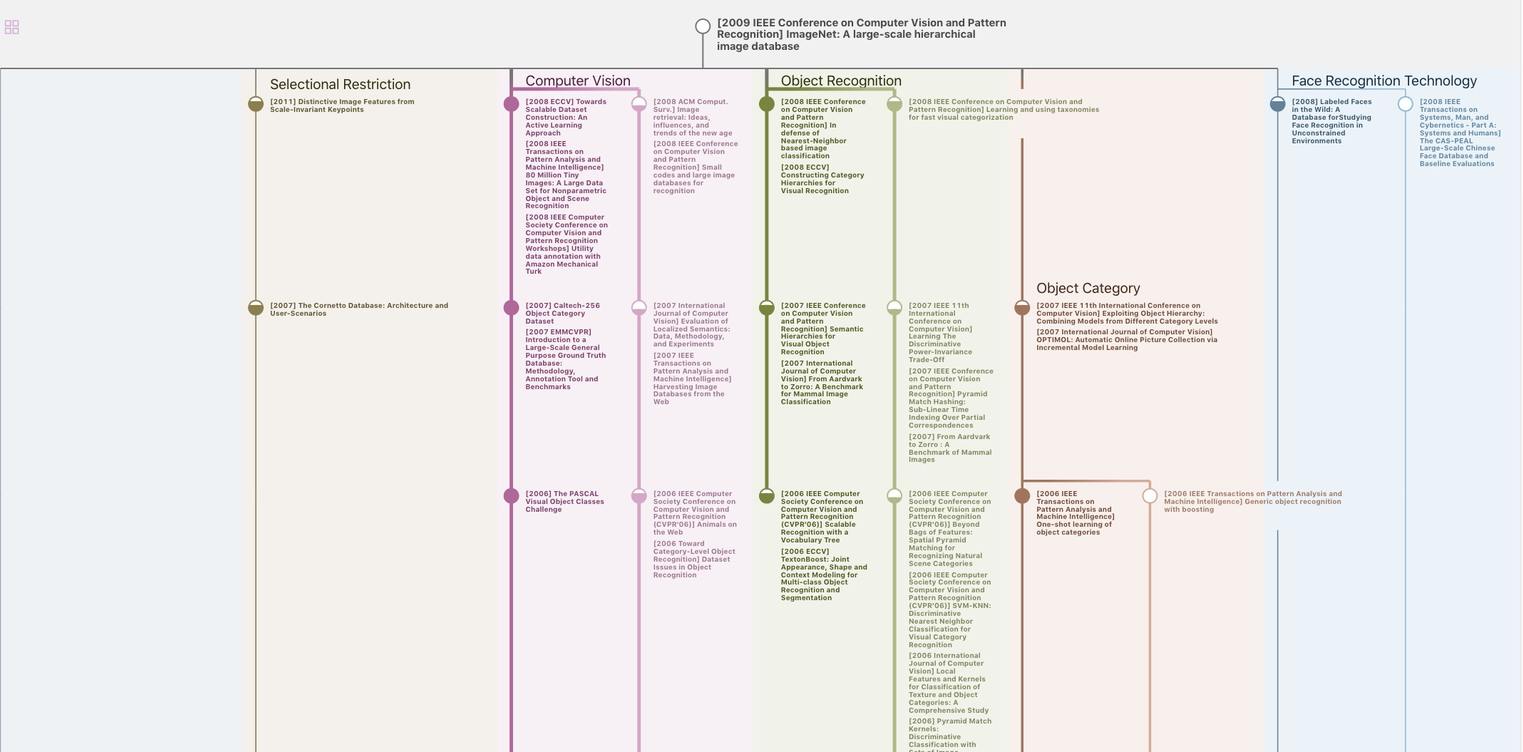
生成溯源树,研究论文发展脉络
Chat Paper
正在生成论文摘要