Hierarchical Learning Of Iree Classifiers For Large-Scale Plant Species Identification
2015 IEEE 9TH INTERNATIONAL CONFERENCE ON SEMANTIC COMPUTING (ICSC)(2015)
摘要
A hierarchical learning algorithm is developed for supporting large-scale plant species identification. A visual tree is first constructed for organizing large numbers of plant species hierarchically in a coarse-to-line fashion. For the line-grained plant species at the sibling leaf nodes under the same parent node, they share significant common visual properties but still contain subtle visual differences, a multi-task structural learning algorithm is developed to train their inter-related classifiers jointly for enhancing their discrimination power. For the coarse-grained categories at the sibling non-leaf nodes under the same parent node, a hierarchical classifier training algorithm is developed to leverage both the tree structure (i.e., inter-level constraint) and the common prediction structures shared among their sibling child nodes (i.e., inter-level visual correlation) to train their inter-related classifiers hierarchically. Our experimental results on large-scale plant images have demonstrated the effectiveness of our algorithm on large-scale plant species identification.
更多查看译文
AI 理解论文
溯源树
样例
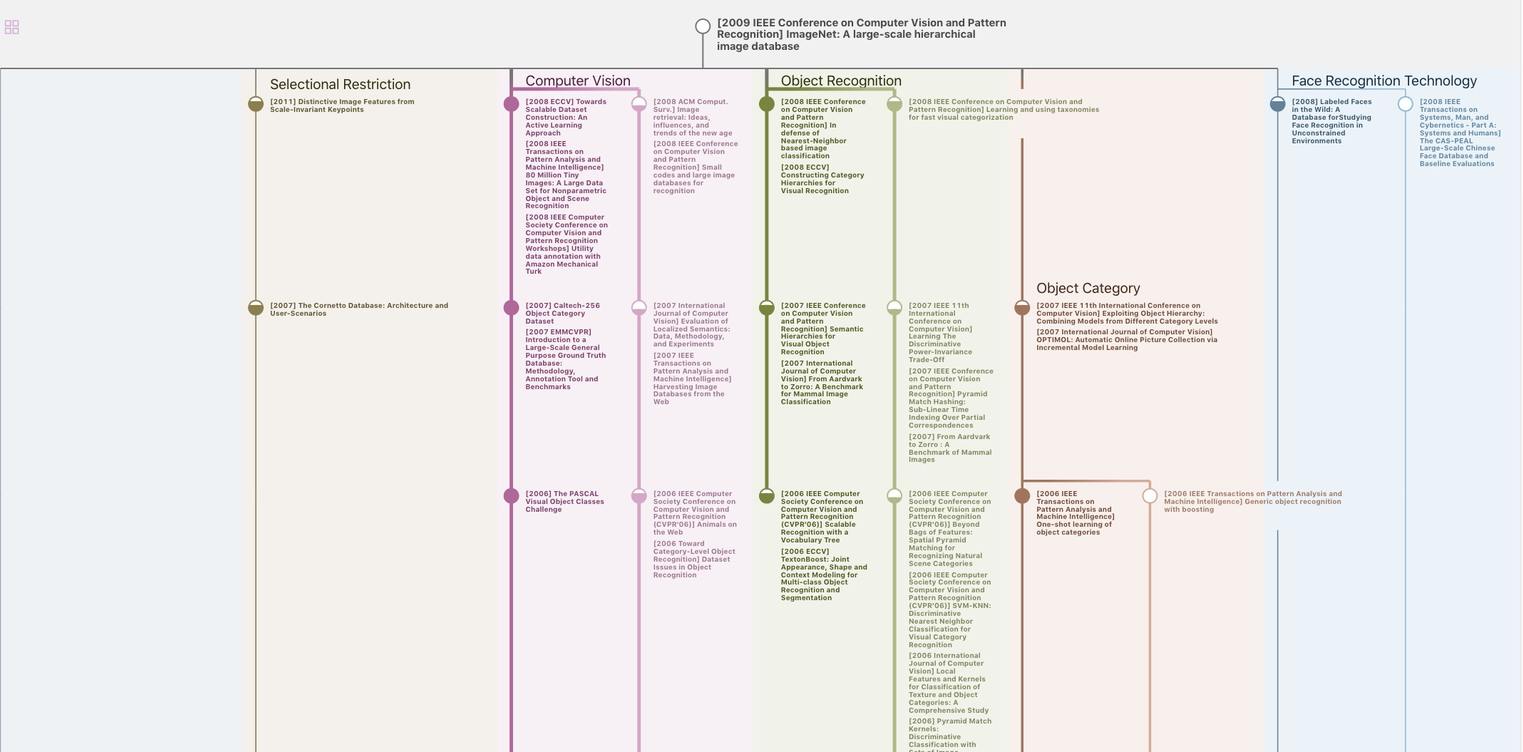
生成溯源树,研究论文发展脉络
Chat Paper
正在生成论文摘要