Multiclass Classification Of Apg Signals Using Elm For Cvd Risk Identification: A Real-Time Application
16TH INTERNATIONAL CONFERENCE ON BIOMEDICAL ENGINEERING(2017)
摘要
In this paper, we present a non-invasive method of classifying a subject's health as "Healthy" or "At Risk" of cardiovascular disease (CVD). The novelty of the work lies in recognizing the rare case of a young subject with cardiovascular disease as well as old subjects who are healthy, and the real-time implementation of CVD risk analysis. Thirty healthy and thirty pathological signals are pre-processed using Empirical Mode Decomposition (EMD), later, the analysis of the acceleration plethysmogram (APG) signals are carried out. Seven features of the wave contour are extracted along with actual age of the subject, four classes are identified using an extreme learning machine (ELM) classifier, and we made four groups which are, Healthy Young, Unhealthy Young, Healthy Old, and Unhealthy Old. Implementation of the proposed system is done on a Raspberry Pi 2 using the Python programming language. The training of the classifier and prediction of CVD risk group, using the extracted features, takes on average 17.83 milliseconds. The overall accuracy of the system is 86%.
更多查看译文
关键词
Cardiovascular diseases, APG signals, Empirical Mode Decomposition, Real-Time implementation, Extreme Learning Machine
AI 理解论文
溯源树
样例
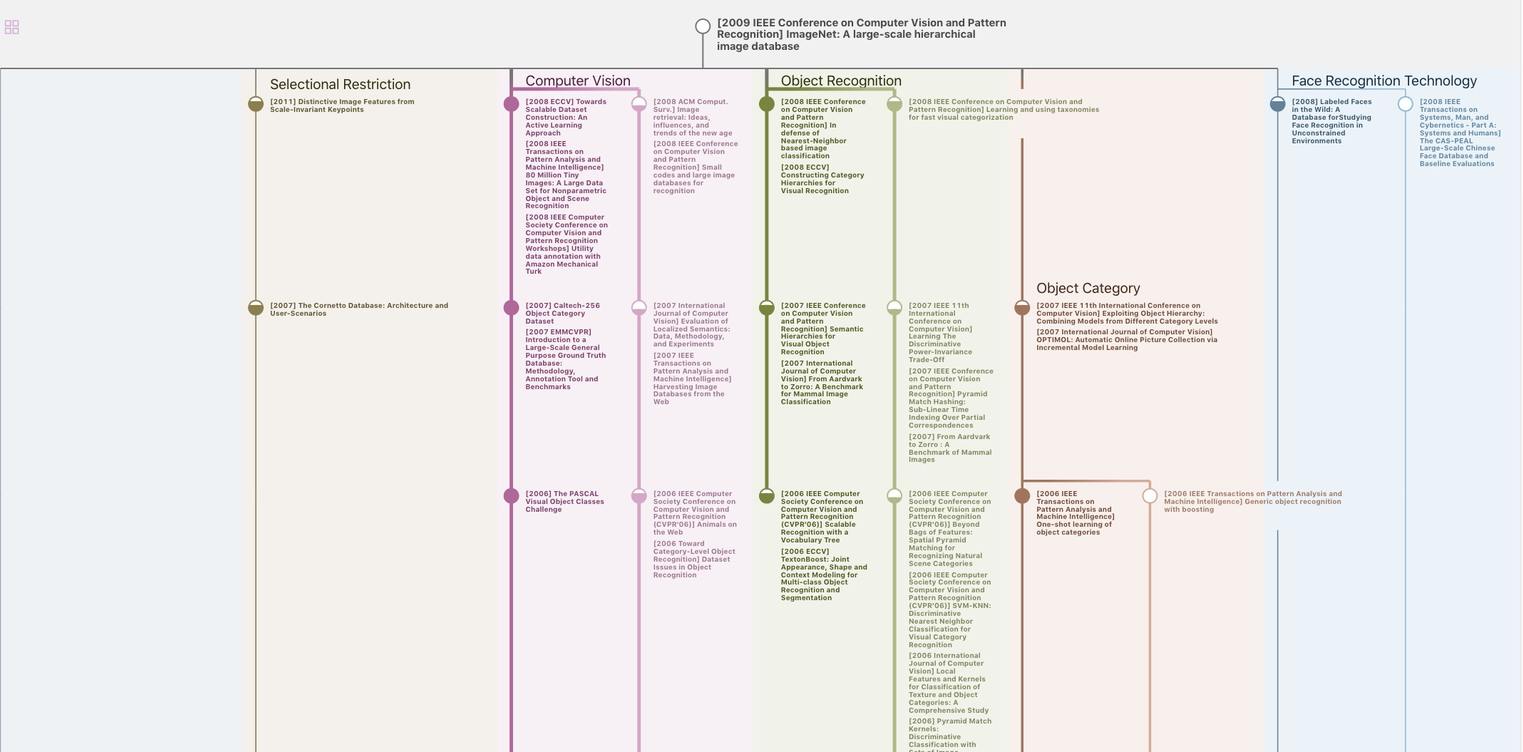
生成溯源树,研究论文发展脉络
Chat Paper
正在生成论文摘要