Matrix Infinitely Divisible Series: Tail Inequalities and Their Applications
IEEE TRANSACTIONS ON INFORMATION THEORY(2020)
摘要
In this paper, we study tail inequalities of the largest eigenvalue of a matrix infinitely divisible (i.d.) series, which is a finite sum of fixed matrices weighted by i.d. random variables. We obtain several types of tail inequalities, including Bennett-type and Bernstein-type inequalities. This allows us to further bound the expectation of the spectral norm of a matrix i.d. series. Moreover, by developing a new lower-bound function for $Q(s)=(s+1)\log (s+1)-s$ that appears in the Bennett-type inequality, we derive a tighter tail inequality of the largest eigenvalue of the matrix i.d. series than the Bernstein-type inequality when the matrix dimension is high. The resulting lower-bound function is of independent interest and can improve any Bennett-type concentration inequality that involves the function $Q(s)$ . The class of i.d. probability distributions is large and includes Gaussian and Poisson distributions, among many others. Therefore, our results encompass the existing work on matrix Gaussian series as a special case. Lastly, we show that the tail inequalities of a matrix i.d. series have applications in several optimization problems including the chance constrained optimization problem and the quadratic optimization problem with orthogonality constraints. In addition, we also use the resulting tail bounds to show that random matrices constructed from i.d. random variables satisfy the restricted isometry property (RIP) when it acts as a measurement matrix in compressed sensing.
更多查看译文
关键词
Linear matrix inequalities, Optimization, Random variables, Eigenvalues and eigenfunctions, Compressed sensing, Gaussian distribution, Covariance matrices, Random matrix, tail inequality, infinitely divisible distribution, largest eigenvalue, optimization, restricted isometry property, compressed sensing
AI 理解论文
溯源树
样例
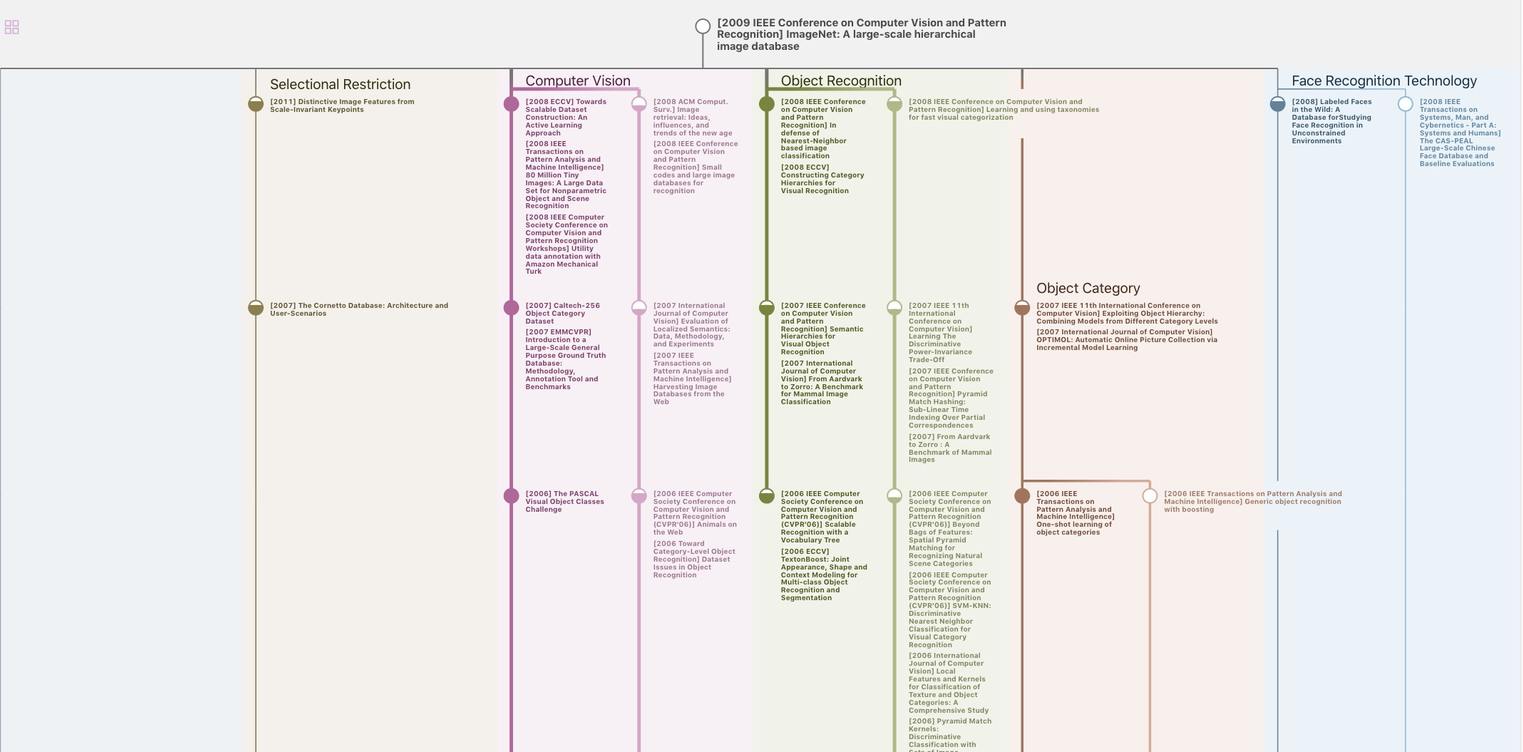
生成溯源树,研究论文发展脉络
Chat Paper
正在生成论文摘要