Reliable State Of Health Condition Monitoring Of Li-Ion Batteries Based On Incremental Support Vector Regression With Parameters Optimization
PROCEEDINGS OF THE INSTITUTION OF MECHANICAL ENGINEERS PART I-JOURNAL OF SYSTEMS AND CONTROL ENGINEERING(2023)
摘要
State of health condition monitoring of Li-ion batteries is an important issue for safe and reliably operation of battery-powered products. Consequently, it remains a challenging subject for industrial and academic studies. In this article, an incremental support vector regression is proposed for battery state of health lifetime estimation. In order to improve the battery state of health forecasting accuracy, the quantum-behaved particle swarm optimization is proposed to define reliably the incremental support vector regression parameters. The validation of the proposed method was done based on the NASA battery data set, and it demonstrates that it yields good performance in remaining useful life estimation of Li-ion batteries. This case study shows that compared with the linear, polynomial regression methods, and compared to previous works, the proposed method can obtain more accurate state of health prediction results. Even for state of health prediction starting from the cycle near capacity regeneration, the proposed model can still accurately estimate the global degradation trend. Furthermore, the proposed quantum-behaved particle swarm optimization-incremental support vector regression combination has greater robustness when the training data contain noise and measurement outliers. This allows satisfactory prediction performances without pre-processing the data manually.
更多查看译文
关键词
Condition monitoring, incremental support vector regression, Li-ion battery, prognostics and health management, state of health, remaining useful life
AI 理解论文
溯源树
样例
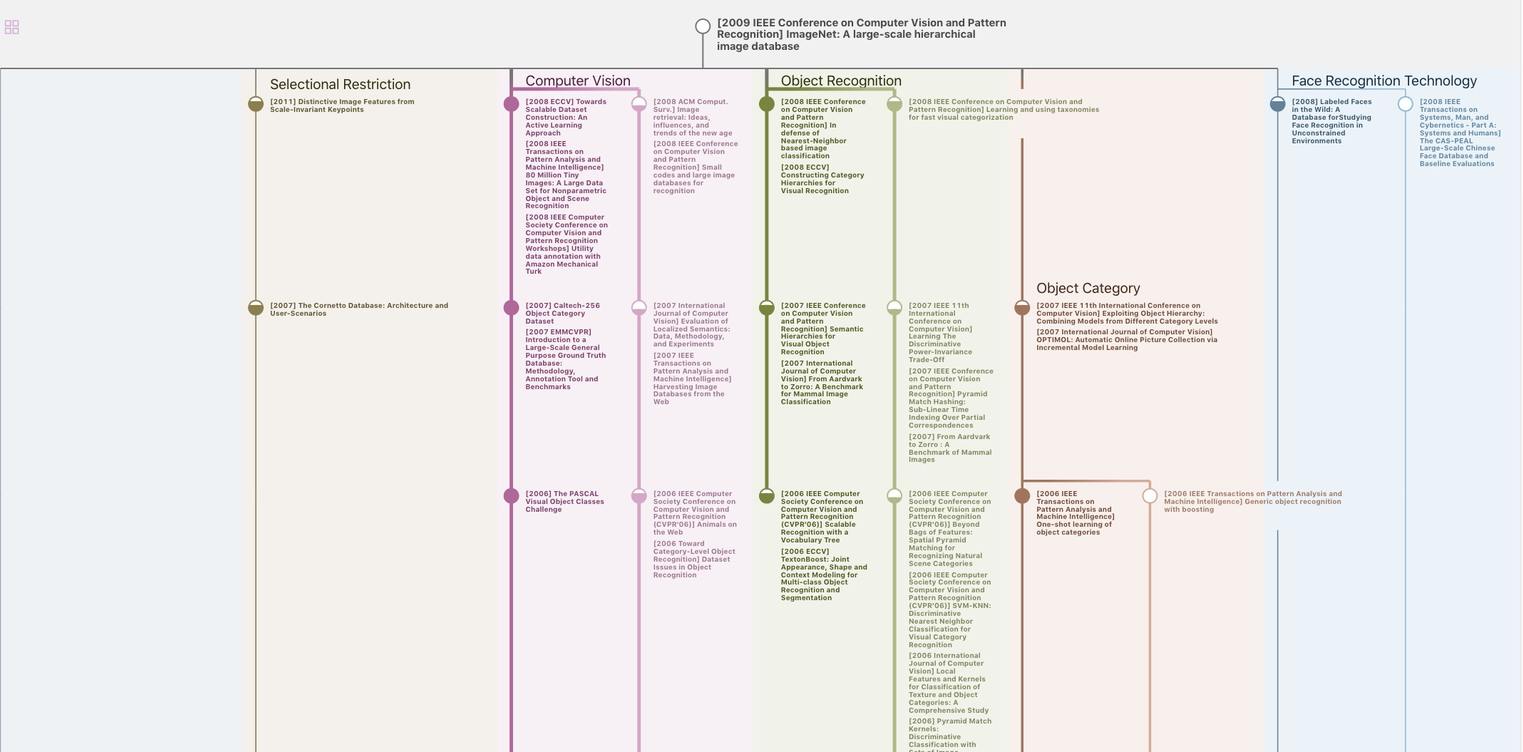
生成溯源树,研究论文发展脉络
Chat Paper
正在生成论文摘要