AI-based Framework for Deep Learning Applications in Grinding
2020 IEEE 18th World Symposium on Applied Machine Intelligence and Informatics (SAMI)(2020)
摘要
Rejection costs for a finish-machined gearwheel with grinding burn can rise to the order of 10,000 euros each. A reduction in costs by reducing rejection rate by only 5-10 pieces per year already amortizes costs for data-acquisition hardware for online process monitoring. The grinding wheel wear, one of the major influencing factors responsible for the grinding burn, depends on a large number of influencing variables like cooling lubricant, feed rate, circumferential wheel speed and wheel topography. In the past, machine learning algorithms such as Support Vector Machines (SVM), Hidden Markov Models (HMM) and Artificial Neural Networks (ANN) have proven effective for the predictive analysis of process quality. In addition to predictive analysis, AI-based applications for process control may raise the resilience of machining processes. Using machine learning methods may also lead to a heavy reduction of cost amassed due to a physical inspection of each workpiece. With this contribution, information from previous works is leveraged and an AI-based framework for adaptive process control of a cylindrical grinding process is introduced. For the development of such a framework, three research objectives have been derived: First, the dynamic wheel wear needs to be modelled and measured, because of its strong impact on the resulting workpiece quality. Second, models to predict the quality features of the produced workpieces depending on process setup parameters and materials used have to be established. Here, special focus is set on deriving models that are independent of a specific wheel-workpiece-pair. The opportunity to use such a model in a variety of grinding configurations gives the production line consistent process support. Third, the resilience of analytical models regarding graceful degradation of sensors needs to be tackled, since the stability of such systems has to be guaranteed to be used in productive environments. Process resilience against human errors and sensor failures leads to a minimization of rejection costs in production. To do so, a framework is presented, where virtual sensors, upon the failure or detection of an erroneous signal from physical sensors, will be activated and provide signals to the downstream smart systems until the process is completed or the physical sensor is changed.
更多查看译文
关键词
Cylindrical Grinding,Wheel Wear,Virtual Sensors,Process Resilience,Artificial Intelligence
AI 理解论文
溯源树
样例
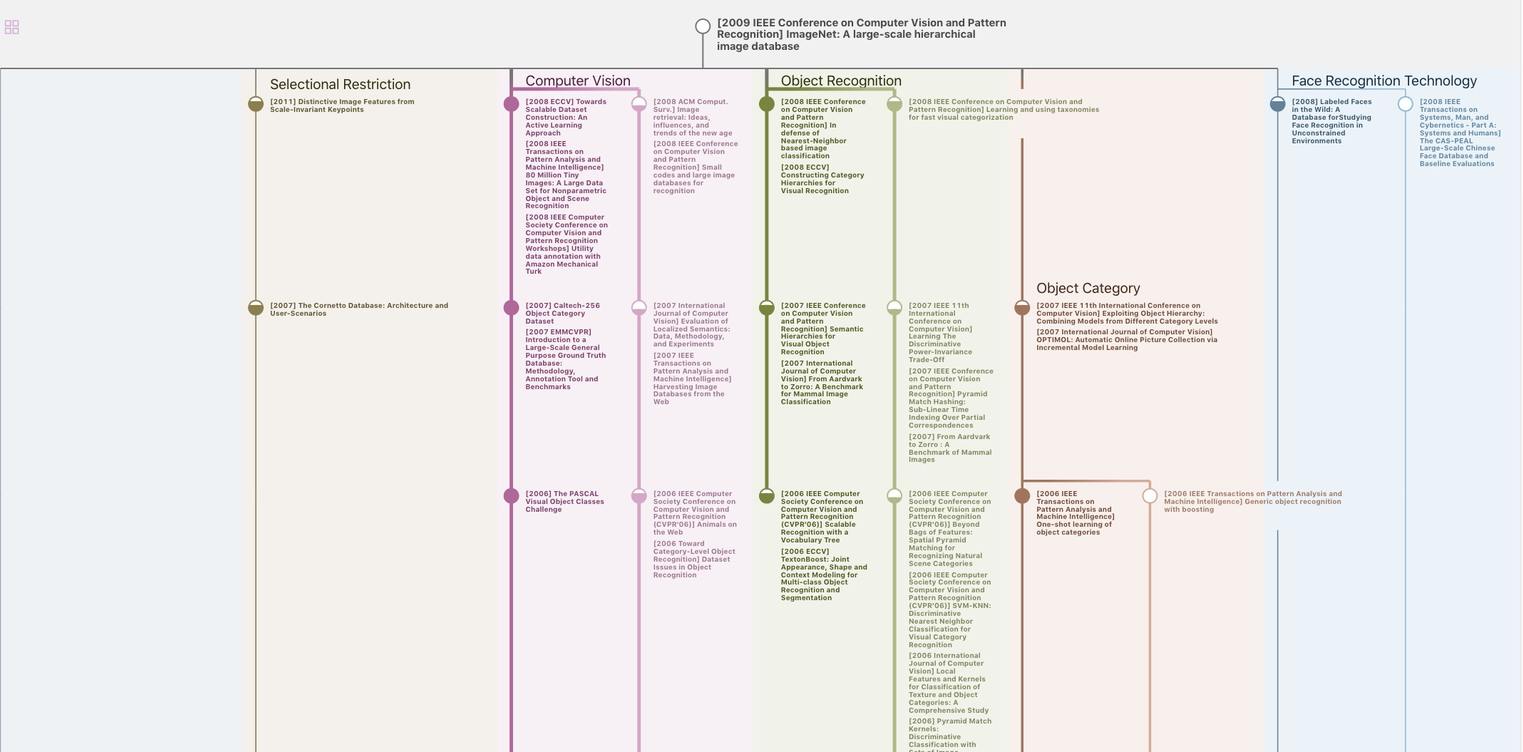
生成溯源树,研究论文发展脉络
Chat Paper
正在生成论文摘要