Prediction Techniques For Diagnosis Of Diabetic Disease: A Comparative Study
INTERNATIONAL JOURNAL OF COMPUTER SCIENCE AND NETWORK SECURITY(2018)
摘要
The objective of supervised learning is to construct a distribution model by considering class values for the purpose of prediction. Nowadays data mining is playing an important role for prediction of diseases in the healthcare industry. Data mining process is used for feature selection, information extraction as well as to discover the unknown pattern and relations from the unstructured data. These patterns can be used to write a wise prescription for patients.This paper introduces an expert system for the early prediction of a diabetic patient by using data mining classification techniques. This work is based on a dataset which comprises of 130-US Hospitals for the years 1999_2008 consisting of 50 attributes and more than 100,000 instances. The evaluation and comparison is performed by using RapidMiner, a software platform of data science, which supports various machine learning steps including results visualization. Random Forest, Decision Stump, Random Tree and ID3 were applied to mine the useful information from data. The extracted information will assist the practitioner to write the precise and wise prescription for diabetic patients.This research work presents description of chosen classification models and dataset. Next, makes evaluation and performs a comparison of performance of 5 classification techniques on chosen dataset. Then, it provides results by considering evlaution metrics such as accuracy, precision and recall. This work finds that the decision tree is the best technique for prediction of disease in diabetic patients.
更多查看译文
关键词
Decision tree, ID3, diabetic patients, data mining
AI 理解论文
溯源树
样例
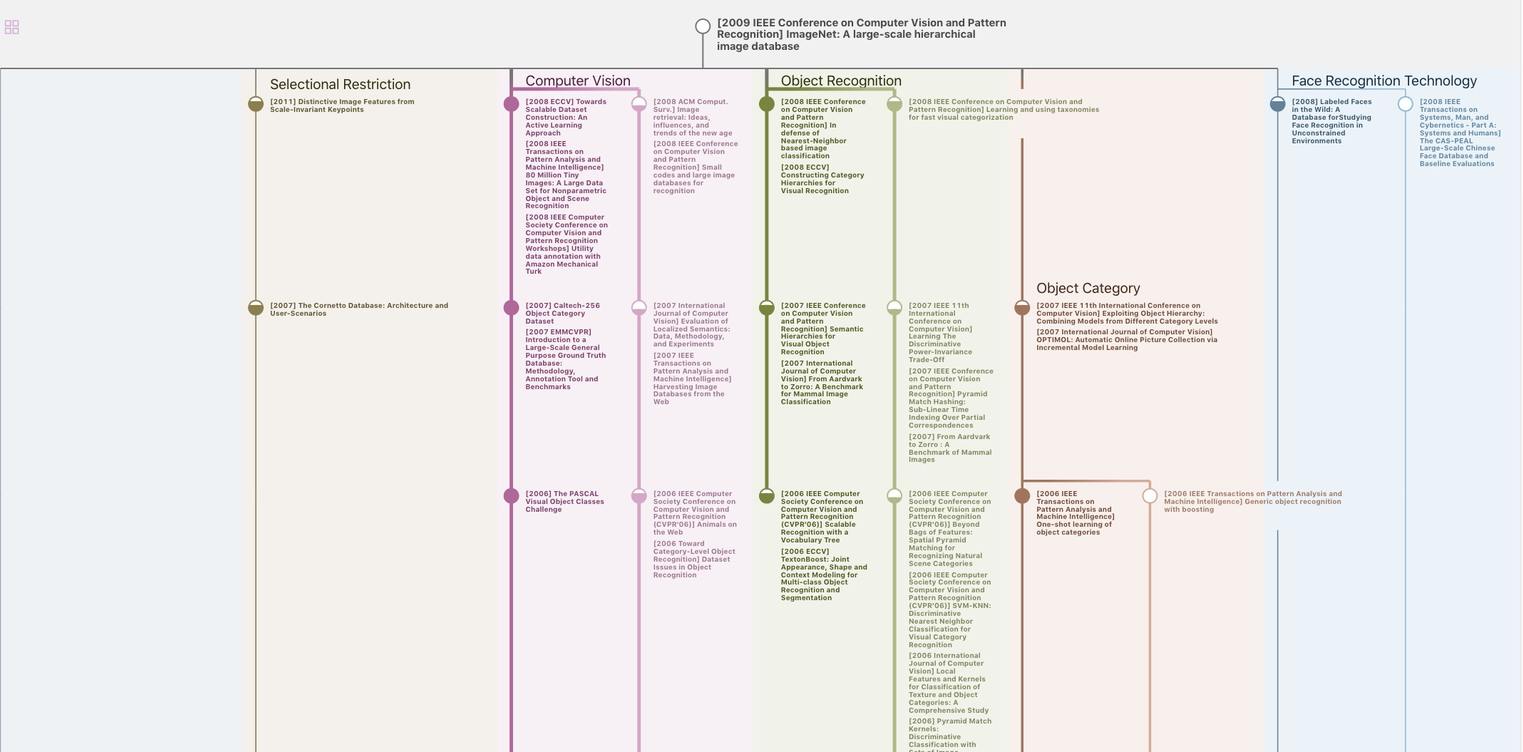
生成溯源树,研究论文发展脉络
Chat Paper
正在生成论文摘要