Long Range Person And Vehicle Detection
ARTIFICIAL INTELLIGENCE AND MACHINE LEARNING IN DEFENSE APPLICATIONS II(2020)
摘要
Automatic detection and tracking of persons and vehicles can greatly increase situational awareness in many military applications. Various methods for detection and tracking have been proposed so far, both for rule-based and learning approaches. With the advent of deep learning, learning approaches generally outperform rule-based approaches. Pre-trained neural networks on datasets like MS COCO can give reasonable detection performance on military datasets. However, for optimal performance it is advised to optimize the training of these pre-trained networks with a representative dataset. In typical military settings, it is a challenge to acquire enough data, and to split the training and test set properly.In this paper we evaluate fine-tuning on military data and compare different pre- and post-processing methods. First we compare a standard pre-trained RetinaNet detector with a fine-tuned version, trained on similar objects, which are recorded at distances different than the distance in the test set. On the aspect of distance this train set is therefore out-of-distribution. Next, we augment the training examples by both increasing and decreasing their size. Once detected, we use a template tracker to follow the objects, compensating for any missing detections.We show the results on detection and tracking of persons and vehicles in visible imagery in a military long range detection setting. The results show the added value of fine-tuning a neural net with augmented examples, where final network performance is similar to human visual performance for detection of targets, with a target area of tens of pixels in a moderately cluttered land environment.
更多查看译文
关键词
person detection, vehicle detection, tracking, training neural nets, augmentation, RetinaNet
AI 理解论文
溯源树
样例
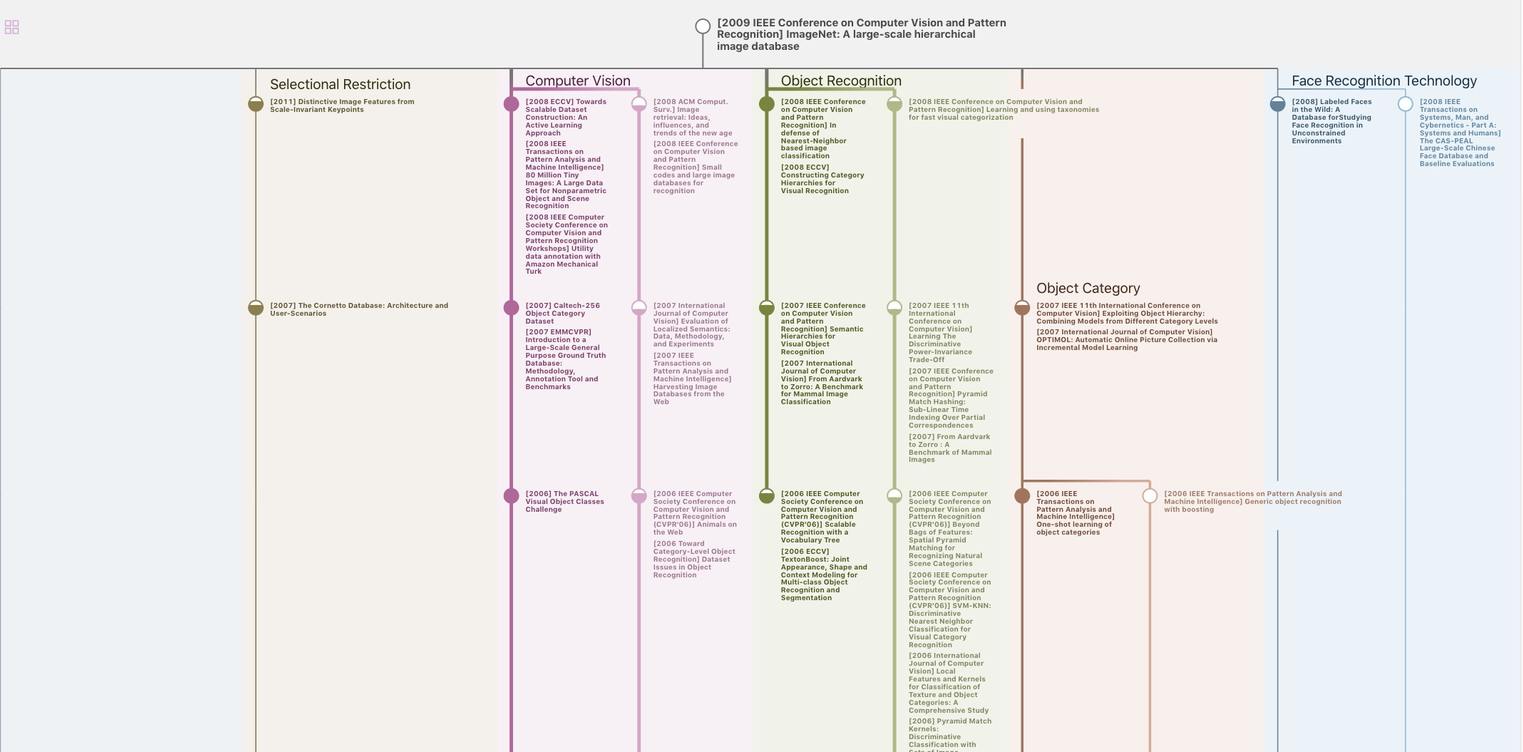
生成溯源树,研究论文发展脉络
Chat Paper
正在生成论文摘要