A New Approach For Detecting Concept Drift And Measuring Its Intensity In Large Datasets
INTERNATIONAL JOURNAL OF COMPUTER SCIENCE AND NETWORK SECURITY(2016)
摘要
The importance of data mining in general and classification in particular has increased in recent years due to the overwhelming amount of digital data that is produced world-wide on a daily basis. In classification, data tuples are mapped to a limited number of classes. The classifier learns (or derives) a classification model from a pre-classified dataset. The learned classification model can be represented in different forms such as a decision tree, set of rules, or support vector machines, to name a few. After the classifier completes the learning phase, it can predict the class of newly added data based on the model that it learned. Quite often a concept drift may occur due to changes in the environment, style, trend, or for many other reasons. Data that used to map to, say, class_a before the drift, now maps to class_b. But based on the knowledge embodied in the model, the system will still wrongfully predict class_a for the same data. This difference between what the model would predict and the actual classification is a sign that a concept drift has occurred and the classification model has become obsolete. In this case, a new model needs to be generated. In this paper we introduce a new efficient algorithm for detecting the occurrence of a concept drift and introduce a way of measuring the intensity of the drift. Measuring the intensity of the drift is important because it impacts how we may choose to deal with it going forward.
更多查看译文
关键词
Classification, Concept Drift, Drift detection, Big Data
AI 理解论文
溯源树
样例
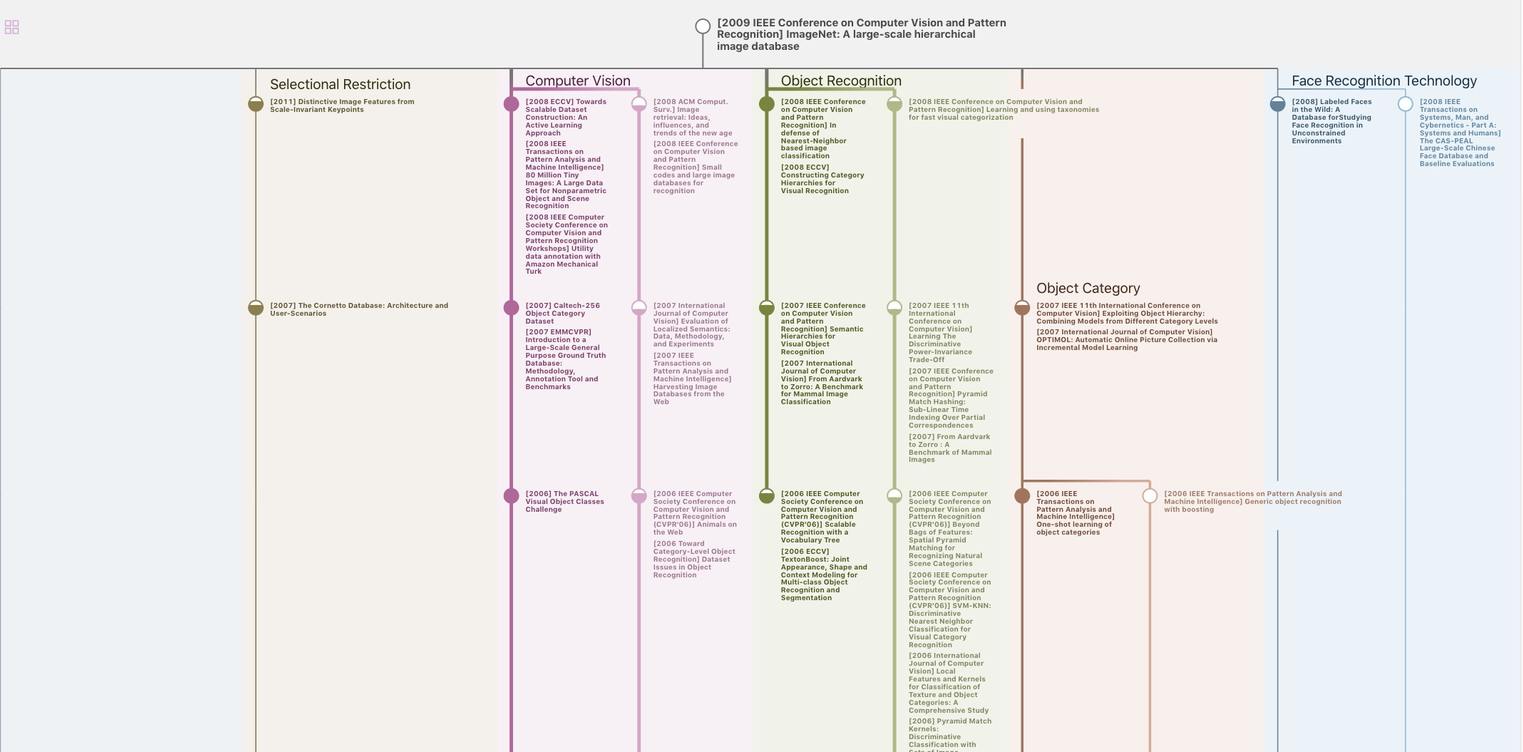
生成溯源树,研究论文发展脉络
Chat Paper
正在生成论文摘要