Class-Imbalanced Dynamical Financial Distress Prediction Based On Synthetic Minority Oversampling Technique And Local Weighted Scheme Integrated With Support Vector Machine
2018 25TH ANNUAL INTERNATIONAL CONFERENCE ON MANAGEMENT SCIENCE & ENGINEERING(2018)
摘要
This study explores the problem of class-imbalanced dynamical financial distress prediction (FDP) based on synthetic minority oversampling technique (SMOTE) and local weighted scheme (LWS) integrated with support vector machine (SVM). LWS is used for dynamically constructing ensemble FDP models by reordering the sequence of data batches and time weighting the base classifiers. The machine learning approach of SVM is adopted as base models. Since SMOTE is a classical data-level approach for treating class imbalance, it is combined with LWS-SVM for solving the problem of class imbalance in dynamical FDP. The two dynamical FDP models of LWS-SVM without SMOTE and LWS-SVM with SMOTE are respectively constructed and their performance is empirically compared. Totally 2,628 Chinese listed companies are collected as samples, among which438 are financial distress samples and 2190 are financial normal samples. Empirical results show that class imbalance has serious impact on LWS-SVM without SMOTE, and the process of SMOTE integrated with LWS-SVM can greatly improve the performance for class-imbalanced dynamical FDP. Therefore, this paper potentially promotes the novel integration of artificial intelligence technology for treating the real-world problem of class-imbalanced dynamical FDP.
更多查看译文
关键词
Class imbalance, Dynamical financial distress prediction, Local weighted scheme, SMOTE, Support vector machine
AI 理解论文
溯源树
样例
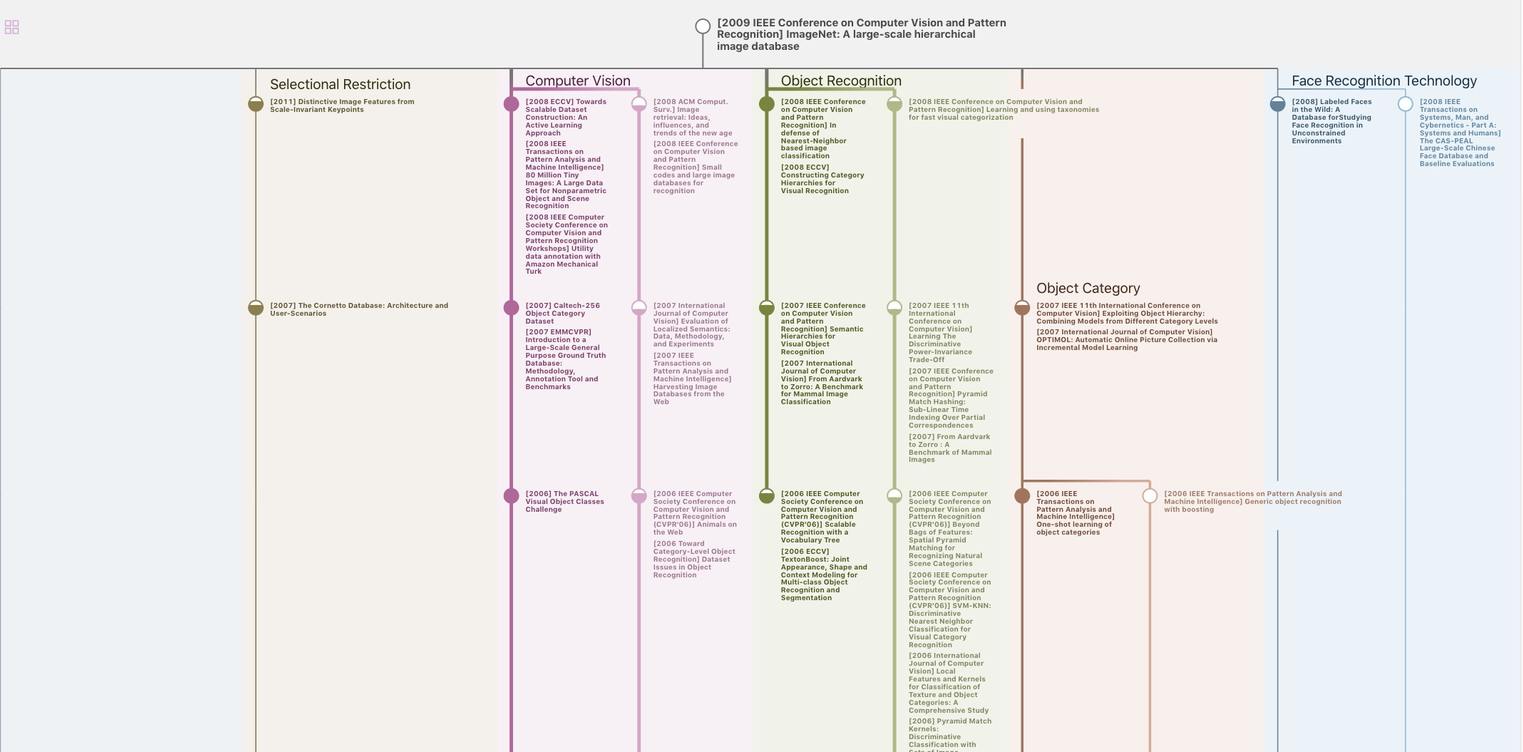
生成溯源树,研究论文发展脉络
Chat Paper
正在生成论文摘要