A Hybrid Filter-Wrapper Feature Selection Approach For Authorship Attribution
INTERNATIONAL JOURNAL OF INNOVATIVE COMPUTING INFORMATION AND CONTROL(2019)
摘要
Many criminals make use of the convenience of anonymity in the cyber world to conduct inappropriate or illegal activities. Authorship attribution aims to identify the most likely author from potential suspects for evidence collection and forensic investigation. Authorship attribution is typically achieved by employing classification algorithms to identify the author based on various writing-style features. However, not all features are useful (relevant) and irrelevant or redundant features may even deteriorate the classification accuracy and slow down the processing time. Feature selection as important data processing techniques can solve this problem, but they have not been investigated in authorship attribution. In this paper, we propose a novel hybrid filter-wrapper feature selection approach to authorship attribution tasks, where a rich set of writing-style features, including syntactic features, lexical features, and structural features, is extracted in order to include all available useful information. In the proposed approach, a correlation based filter feature selection method is used to filter out irrelevant features and then a particle swarm optimization based wrapper method is proposed for feature selection to further remove redundant features, select only relevant features. Experiments on real-life Blog and E-mail datasets show that the proposed approach can improve the classification performance by selecting only a small subset of features, and achieve better classification performance than filter only, wrapper only, and a commonly used wrapper method (linear forward selection).
更多查看译文
关键词
Feature selection, Filter-wrapper, Particle swarm optimization, Authorship attribution, Forensic
AI 理解论文
溯源树
样例
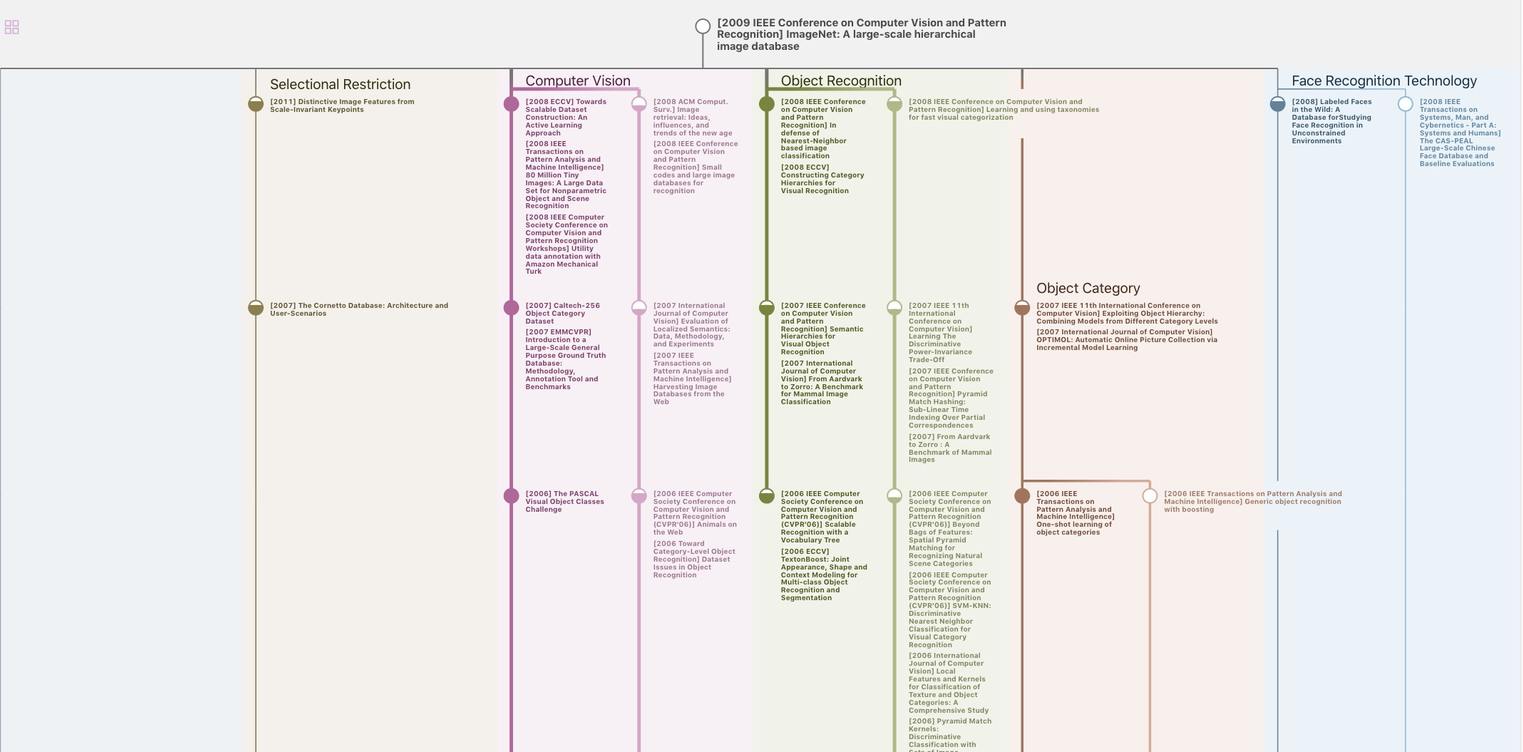
生成溯源树,研究论文发展脉络
Chat Paper
正在生成论文摘要